From chess-playing robots to a future beyond our control: Prof Stefan Leijnen discusses the challenges of AI and its evolution
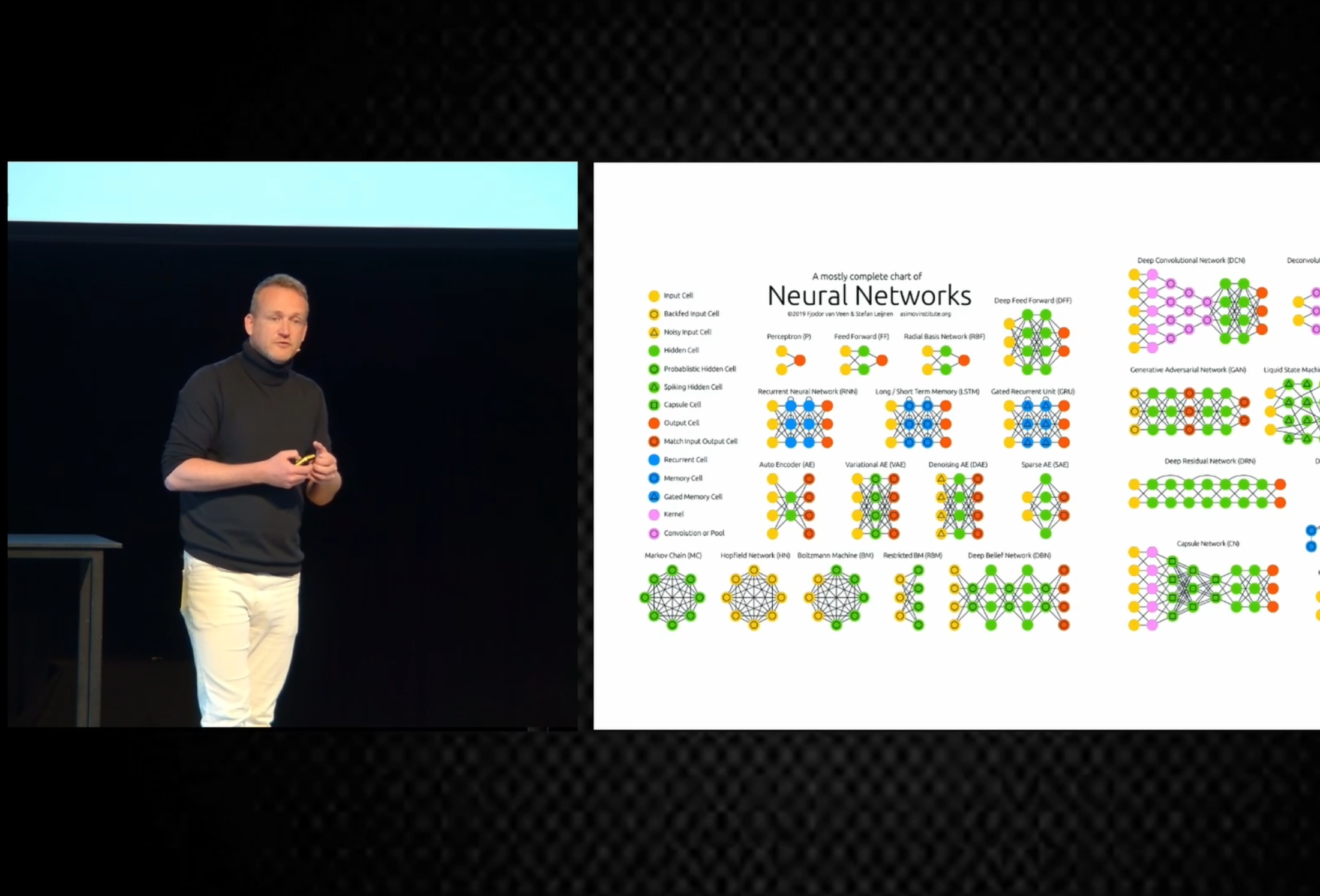
Stefan’s Leijnen experience spans cutting-edge AI research and public policy. As professor in applied AI, he focuses on machine learning, generative AI, and the emergence of artificial sentience. As the lead for EU affairs for the Dutch National Growth Fund project AiNed, Stefan plays a pivotal role in defining public policy that promotes innovation while protecting citizens’ rights.
In this captivating presentation, Stefan takes us on a journey through 250 years of artificial intelligence, from a chess-playing robot in 1770 to the modern complexities of machine learning. With thought-provoking anecdotes he draws parallels between the past and the ethical challenges we face today. As the lead in EU AI policy, Stefan unpacks how AI is reshaping industries, from Netflix’s algorithms to self-driving cars, and why we need to prepare for its profound societal impacts.
Below we summarize his insights as a series of lessons and share the full video presentation and its transcript.
Prepare to rethink what you know about AI and its future.
Lesson 1. AI behaviour is underpinned by models that cannot be understood by humans
10 years ago, Netflix asked the following question: “How do we know that the categories that we designed with our limited capacity as humans are the best predictors for your viewing preferences? We categorize people according to those labels, but those might not be the best labels. So let’s reverse engineer things: now that we have all this data, let’s decide what categories are the best predictors for our viewership.” And they did that. And so they come up with 50 dimensions or 50 labels, all generated by the computer, by AI. And 20 of them made a lot of sense: gender, age, etc. But for 30 of those 50 labels, you could not identify the category. That means that the machine uncovered a quality among people that we don’t have a word for. For Netflix this was great because it meant they now had 30 more predictors. But on the other hand, it’s a huge problem. Because now if you want to change something in those labels or you want to change something in the way that you use the model, you no longer understand what you’re dealing with.
Watch the video clip.
Lesson 2. AI’s versatility can lead to hidden – and very hard – ethical problems
Let’s say the camera of a self-driving car spots something and there’s a 99% chance that it is just a leaf blowing by, and a 1% chance that it’s a child crossing the street. Do you break? Of course, you would break in a 1% chance. But now let’s lower the chance to 0.1% or 0.01%. At what point do you decide to break?
The point, of course, is that we never make that decision as humans. But with rule-based programs, you have to make that decision. So it becomes an ethical problem. And these kind of ethical problems are much more difficult to solve than technological problems. Because who’s going to answer that? Who’s going to give you this number? It’s not the programmer. The programmer will go to the manager or to the CEO and they will go to the legal division or to the insurer or to the legislator. And nobody’s willing to provide an answer. For moral reasons (and for insurance reasons), it’s very difficult to solve this problem. Now, of course, nowadays there’s a different approach: just gather the data and calculate the probability of breaking that humans have. But in doing so, you have moved the ethical challenge under the rug. But it’s still there. So don’t get fooled by those strategies.
Watch the video clip.
Lesson 3. AI impact is unpredictable. But its impact won’t be just technological. It will be societal, economical, and likely political
There are other systems technologies like AI. We have the computer, we have the internet, we have the steam engine and electricity. And if you think about the steam engine, when it was first discovered, nobody had a clue of the implications of this technology 10, 20 or 30 years down the line. The first steam engines were used to automate factories. So instead of people working on benches close to each other, the whole workforce was designed along the axis of this steam engine so everything would be mechanically automated. This meant a lot of changes to the workforce. It meant that work could go for hours on end, even in the evenings and in the weekends. That led to a lot of societal changes. So labor forces emerged, you had unions, you had new ideologies popping up. The steam engine also became a lot smaller. You got the steam engine on railways. Railways meant completely different ways of warfare, economy, diplomacy. The world got a lot smaller. This all happened in the time span of several decades. We will see similar effects that are completely unpredictable as AI gets rolled out in the next couple of decades. Most of these effects of the steam engine were not technological. They were societal, economical, sometimes political. So it’s also good to be aware of this when it comes to AI.
Watch the video clip.
Lesson 4. The interfaces with AI will evolve in ways we do not yet anticipate.
The AI that we know now is very primitive. Because what we see today in AI is a very old interface. With ChatGPT, it’s a text command prompt. When the first car was invented, it was a horseless carriage. When the first TV was invented, it was essentially radio programming with an image glued on top of it. Now, for most of you who have been following the news, you already see that the interfaces are developing very rapidly. So you’ll get voice interfaces, you’ll get a lot more personalization with AI. This is a clear trend.
Watch the video clip.
Watch the full video.
(Full transcript below.)
Full transcript.
The transcript has been edited for brevity and clarity.
Prof Leijnen: Does anybody recognize this robot that I have behind me? Not many people. Well, that’s not surprising because it’s a very old robot. This robot was built in the year 1770, so over 250 years ago. And this robot can play chess.
And it was not just any chess-playing robot. It was actually an excellent chess-playing robot. He won most games. And as you can imagine at that time, it was a celebrity. This robot played against Benjamin Franklin. It played against emperors and kings. It also played a game against Napoleon Bonaparte. We know what happened because there were witnesses there. In fact, Napoleon being the smart man that he is, he decided to play a move in chess that’s not allowed just to see the robot’s reaction. What the robot did is it took the piece that Napoleon moved and put it back in its original position.
Napoleon being inventive, did the same illegal move again. Then the robot took the piece and put it beside the board as though it’s no longer in the game. Napoleon tried a third time and then the robot wiped all the pieces off the board and decided that the game was over, to the amusement of the spectators. Then they set up the pieces again, played another game and Napoleon lost. Well, to me, this is really intelligence.
You might think or not think that this is artificial intelligence. If you think this is not artificial, you’re right. Because there’s this little cabinet which has like magnets and strings and there was a very small person that could fit inside this cabinet and who was very good at playing chess. And of course, he is the person who played the game. People only found out about 80 years after the facts when the plans were revealed by the son of von Kempelen.
Now, there was another person who played against this robot and his name was Charles Babbage. And Charles Babbage is the inventor of this machine, the analytical engine. And it’s considered by many to be the first computer in the world. It was able to calculate logarithms. Interestingly, Babbage played against the robot that you just saw. He also lost in 18 turns. But I like to imagine that Babbage must have been thinking how does this robot work, what’s going on inside.
As some of you may know, a computer actually beat the world champion in chess, Gary Kasparov in 1997. So you could say in this story spanning 250 years is a nice story arc. Because now we do have AI that can play and win chess, which was the original point of the chess-playing robots. So we’re done. We’re actually done with AI. We have AI now. The future is here. But at the same time, we’re not done at all. Because now we have this AI and we don’t know how to use it. And we don’t know how to develop it further.
There is a nice example from Netflix, the streaming company. They collect a lot of data. They have your age, gender, postal code, maybe your income. They know things about you. And then based on those categories, they try to predict with machine learning what type of series and movies you like to watch. And this is essentially their business model. Now, 10 years ago they asked the following question: “How do we know that the categories that we designed with our limited capacity as humans are the best predictors for your viewing preferences? We categorize people according to those labels, but those might not be the best labels. So let’s reverse engineer this machine learning algorithm. And now that we have all this data, let’s decide what categories are the best predictors for your viewership.”
And they did that. And they came up with 50 dimensions or 50 labels that you could attach to a viewer, all generated by the computer, by AI. And 20 of them made a lot of sense. So you would see men here, women there. You would see an age distribution. And there was very clear preferences in viewership. Of course, not completely uniform, but you could identify the categories and you could attach a label to them.
Now for 30 of those 50 labels, you could not identify the category. For example, for one of the 30 categories, you would see people on the left side, people on the right side. On the left side, they had a strong preference for the movie “American Beauty.” And on the right side, they had a strong preference for X on the beach. And nobody had any clue what discerned the group on the left from the group on the right. So that means that there was a quality in those groups of people that we don’t have a word for. We don’t know how to understand that. Which for Netflix was great because it means they had now 30 more predictors they could use to do good predictions. But on the other hand, it’s a huge problem. Because now if you want to change something in those labels or you want to change something in the way that you use the model, you no longer understand what you’re dealing with.
And this is essentially the topic of what I am talking about today. How do you manage something you can’t comprehend? Because essentially that’s what AI is. And this is not just a problem for companies implementing AI. We all know plenty of examples of AI going wrong. And when it goes wrong, it tends to go wrong quite deeply. Like in this case, if you ask AI to provide you with an image of a salmon, the AI is not wrong. Statistically speaking, it is the most likely image of a salmon you’ll find on the internet. But of course we know that this is not what was expected. And this is not just a bug. It’s a feature of AI.
I teach AI, I teach students how to program AI systems and machine learning systems. If I ask my students to come up with a program, without using machine learning or AI, that filters out the dogs from the cakes in these kind of images. It will be very difficult because it’s very hard to come up with a rule set that can discern A from B. At the same time, we know that for AI, machine learning, this is a very easy task. And that’s because the AI programs itself. Or in other words, AI can come up with a model that is so complex that we don’t understand how it works anymore, but it still produces the outcome that we’re looking for. In this case, a category classifier.
And that’s great because those very complex models allow us to build systems of infinite complexity. There’s no boundary to the complexity of the model. Just the data that you use, the computing power that you use, the fitness function that you use, but those things we can collect. But it’s also terrible because we don’t know how to deal with this complexity anymore. It’s beyond our human comprehension.
Now, about 12, 13 years ago, I was in Mountain View at Google. They had a self-driving car division. The head of their self-driving car division explains the following problem to us. He said: “We have to deal with all kinds of technical challenges. But what do you think our most difficult challenge is?” Now, this was a room full of engineers. So they said, “Well, the steering or locating yourself on the street or how do I do image segmentation?” He said, “No, you’re all wrong. Those are all technical problems that can be solved. There’s a much deeper underlying problem here. And that’s the problem of when do I break?”
Let’s say the camera spots something and there’s a 99% chance that it’s a leaf blowing by. And it’s a 1% chance that it’s a child crossing the street. Do you break? Well, of course, you would break in a 1% chance. But now we lower the chance to 0.1 or 0.01. At what point do you decide to break? The point, of course, is that we never make that decision as humans. But when you program a system like that, you have to make a decision because it’s rule-based. So you have to say if the probability is below this and that, then I break. So it becomes an ethical problem. And these kinds of ethical problems are much more difficult to solve than technological problems. Because who’s going to answer that? Who’s going to give you this number? It’s not the programmer. The programmer will go to the manager or to the CEO and they will go to the legal division or to the insurer or to the legislator. And nobody’s willing to provide an answer. For moral reasons, also for insurance reasons, it’s very difficult to solve this problem, he said. Now, nowadays they have a different approach. They just gather the data and they say based on this data, this is the probability of breaking that humans have. And so they moved the ethical challenge under the rug. But it’s still there. Don’t get fooled by those strategies.
The examples I showed, of Netflix and Google, are from tech companies. But you see it everywhere. We also know that AI is going to play a major role in healthcare in the future. Not just in medicine, but also in caring for the elderly, for monitoring, for prevention, etc. This raises lots of ethical questions. Is this desirable? Here we see a woman who needs care. There’s no care for her. This is from the documentary “Still Alice”. And there’s this robot companion taking care of her, mental care. Is this what we want or is this not what we want? Again, it’s not a technical question. It’s a moral question.
In the last 10, 15 years and in the foreseeable future, AI has moved from the lab to society. ChatGPT is adopted at a much higher rate than most companies know. A large percentage of employees use ChatGPT. But if you ask company CEOs, they probably mention a lower number because many employees use these kind of tools without the company knowing it. We know that a majority of jobs in the Netherlands will be affected by AI, either by full of partial displacement, or AI complementing their work. And we also know that there are enormous opportunities in terms of automation. So on the one hand, it’s very difficult to manage such technology, not just its bugs, but its intrinsic properties. On the other hand, it provides enormous promises for problems that we don’t know how else to solve.
So it’s wise to take a step back and think more deeply and more long-term about the effects of this technology – before we start thinking about how to innovate and how to regulate the technology. What helps us is looking back a little bit. There are other systems technologies like AI. We have the computer, we have the internet, we have the steam engine and electricity. And if you think about the steam engine, when it was first discovered, nobody had a clue of the implications of this technology 10, 20 or 30 years down the line. The first steam engines were used to automate factories. Instead of people working on benches close to each other so they could talk, the whole workforce was designed along the axis of this steam engine so everything would be mechanically automated. This meant a lot of changes to the workforce. It meant that work could go on hour after hour, even in the evenings and in the weekends because now you have this machine, and you want to keep using it. That led to societal changes. You had labor forces, you had unions, you had new ideologies popping up. The steam engine also became a lot smaller. You got the steam engine on railways. Railways meant completely different ways of warfare, economy, diplomacy. The world got a lot smaller. This all happened in the time span of several decades but we will see similar effects that are completely unpredictable as AI gets rolled out in the next couple of decades. Most of these effects of the steam engine were not technological. They were societal, economical, sometimes political. So it’s also good to be aware of this when it comes to AI.
A second element of AI is the speed at which it develops. I’ve been giving talks about artificial creativity for about 10 years now and 10, 8 years ago, it was very easy for me to create a talk. I could just show people this image and then I would say, this cat does not exist and people would be taken aback. This was the highlight of my presentation. Now I show you this image and nobody raises an eyebrow. And then two years later, I had to show this image. Again, I see no reaction from you. I don’t expect any reaction, by the way. But it shows just how fast it goes and how quickly we adopt and get used to these kind of tools. And it also raises the question: given what was achieved in the past 8 years, where will we be in 25 years from now? You can, of course, apply AI in completely new different fields, similar as was done with the steam engine: creating new materials, coming up with new inventions, new types of engineering. We already know that AI has a major role to play in creativity and in coding.
We also know that the AI that we know now is very primitive. I’ll be giving another speech in 10 years and the audience won’t be taken aback by anything. Because what we see today is AI with a very old interface. At ChatGPT, it’s the interface of the internet [i.e. a text command prompt], of the previous system technology. And that’s always been the case. When the first car was invented, it was a horseless carriage. When the first TV was invented, it was essentially radio programming with an image added to it. And now what we have with AI is an internet browser with an AI model behind it. And for most of you who have been following the news, you see that the interfaces are developing very rapidly. You’ll get voice interfaces, you’ll get a lot more personalization with AI. This is also a trend that’s very clear.
So we talked a bit about the history, we talked a bit about the pace of development, about the complexity being a feature, not a bug. We can also dive a little bit more into the technology itself. This is a graph I designed five years ago with a student of mine. It’s called the Neural Network Zoo. And what you see is from the top left, all the way to the bottom right, is the evolution of neural network architectures. Interestingly, at the bottom right, this is called the transformer architecture. Essentially, the evolution stopped and most AI that you hear about nowadays, most AI developed at Microsoft and Google and OpenAI and others are based on this transformer architecture. So there was this Cambrian explosion of architectures, and then suddenly it converged.
Until five years or so AI models were proliferating. Now they’re also converging. Nowadays, we talk about OpenAI’s GPT, we talk about Google’s Gemini, we talk about Meta’s LAMA, Mistral. There aren’t that many models. So not just the technology has been locking in, but the models themselves as well. So you see huge conversions into only a very limited set of players and models. And this is of course due to the scaling laws. It becomes very difficult to play in this game. But it’s very interesting that on the one hand you have a convergence to a limited set of models in a limited set of companies. And on the other hand, you have this emergence of new functionalities coming out of these large scale models. So they surprise us all the time, but they’re only a very limited set of models that are able to surprise us. And these developments, these trends, all inform the way that we regulate this technology.
This is currently how the European Union thinks about regulating this technology. You have four categories. (1) A minimal risk category where there’s not much or hardly any legislation. (2) A limited risk. For example, if I interact with a chatbot, I have to know I’m interacting with a chatbot and not a human. The AI has to be transparent. (3) A high risk category, where there will be all kinds of ethical checks around, let’s say toys or healthcare or anything that has a real risk for consumers or citizens or society. (4) Unacceptable risk, which is AI systems that can subconsciously influence you, do social scoring, etc. Those will all be forbidden under new legislation (the EU AI Act).
I’ll end the presentation with this final quote, because I think this is essentially where we are right now: “The real problem of humanity is the following: we have paleolithic emotions, medieval institutions and with AI, god-like technology.” (E.O. Wilson).