AI in Financial Services
We’re working with retail banks, private banks, insurers, and credit card companies to use predictive analytics and machine learning to streamline decision-making, reduce risk and enhance customer experience.
We’re rapidly advancing the use cases of AI across financial services through our work with numerous pioneering banks and institutions.
From using predictive analytics to optimize loan decisions to applying machine learning to improve fraud detection and prevention – the value you achieve from AI depends on your organization’s readiness to implement these solutions. We make sure you’re prepared – in terms of data foundations, leadership engagement, and the capability within your teams.
Extending the frontiers of performance with artificial intelligence in finance
We’re working across the financial services sector to optimize operations, enhance customer experience, and elevate performance with data and AI. Retail banks, private banks, insurers, credit card companies – we’ve guided a wide variety of financial clients right from their first tentative steps in AI all the way to complex, boundary breaking applications.
The financial services industry has a huge appetite for AI and machine learning. And in many cases, they’ve built a sophisticated data team to help deliver it. We work with your teams to complement and enhance their data and AI work, so it can be developed and scaled to deliver value across the widest possible range of use cases.
Our clients
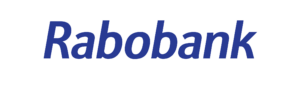
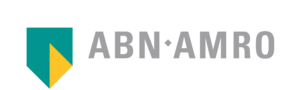
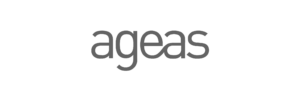
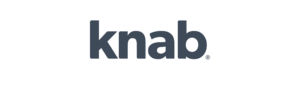
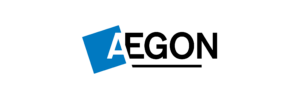
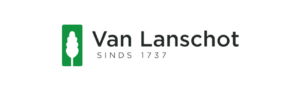
Our impact
25%
Valuation accuracy improvement
Our AI-powered valuation platform for a global fleet management business improved accuracy across their 32 geographic markets.
€100 m
Additional asset value
By enhancing value prediction by 4% for a world-leading automotive leasing company our Program added €100m additional value to the balance sheet.
75%
Cost reduction
Our XGBoost-powered modelling engine reduced costs by 75% for an International Asset Management client
Benchmark your AI maturity
Discover how your AI maturity ranks against peers and what to do next to become an AI-native organisation.
Learn more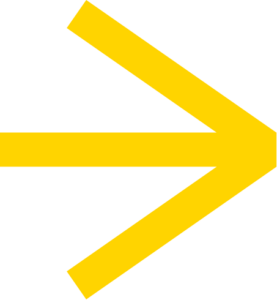