Booking.com is one of the world's largest online travel agencies, handling over a million reservations per day across 2.7 million properties in over 220 countries and in over 40 languages.
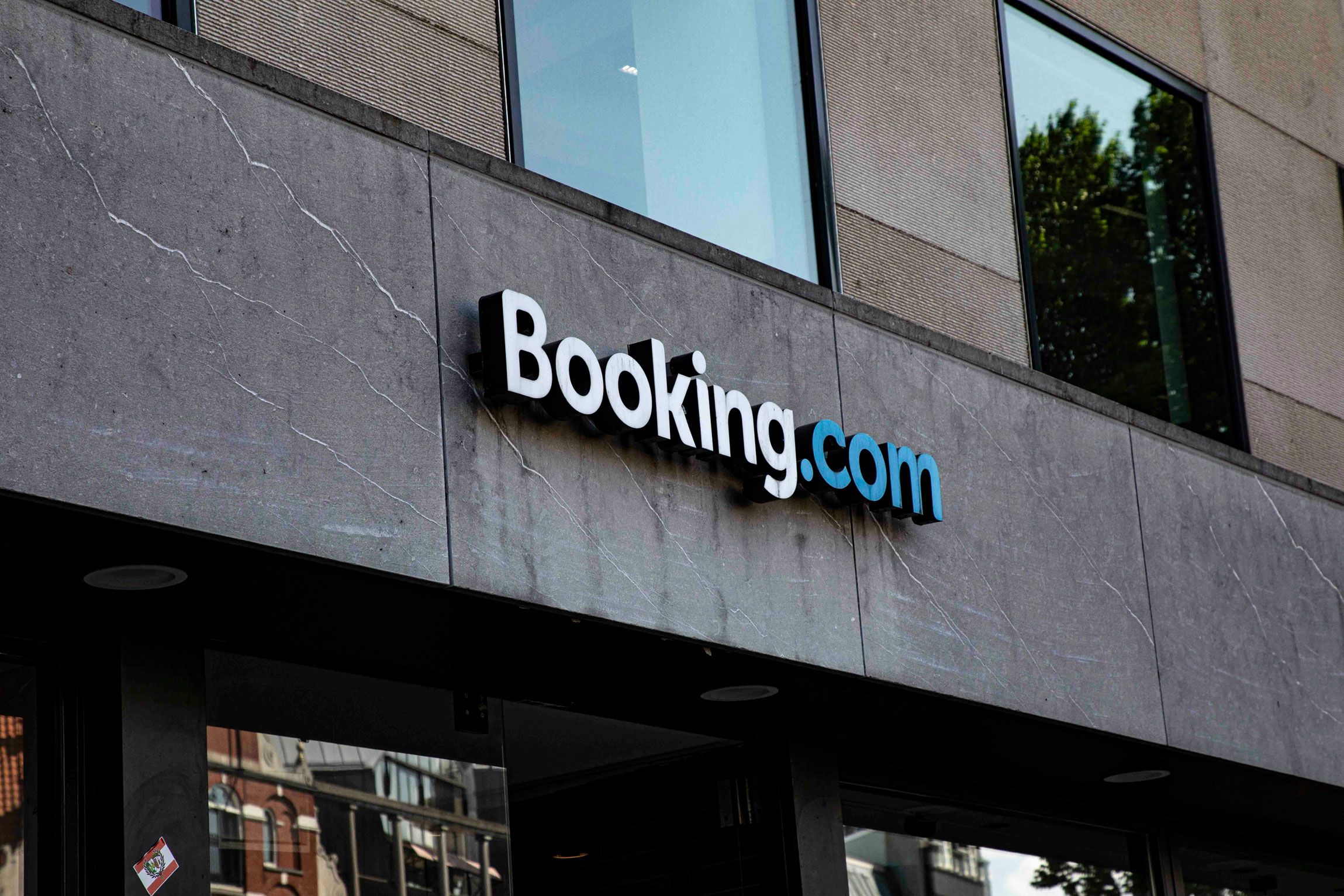
Challenge
Booking.com’s Customer Service (CS) center employs thousands of agents to handle over a million phone and email interactions, in 43 different languages, every day. Scheduling a workforce of such scale and complexity has been a challenge for some time. But with rapid growth in new markets, the daily 13-week forecast accuracy of CS Workload – a key metric – was deteriorating. In some markets by more than 25%. The goal was to halve forecast errors with a system that agents and managers could easily understand and adopt.
Solution
We developed a prototype which could easily scale on proof of concept and tested in two language markets – one European and one Asian. Our approach was based on four principles:
- Understand the business dynamics that inform the patterns.
- Smartly combine data and select only the most important drivers to reduce complexity.
- Thoroughly test different techniques and manage outliers by identifying and understanding them.
- Build a system that integrates a learning loop to correct forecast deviations.
Outcome
The total workload forecast error decreased by 60% for the pilot European language and 50% for the pilot Asian language. This was primarily driven by a 75% improvement in Contact Volume Forecast accuracy. A 60% improvement in Handling Time Forecast accuracy was also a major factor. When applied to the remaining 41 Customer Service languages, only two did not show improvement. These two outliers will be the initial focus of our test & learn approach.