Home
Artificial Intelligence
Jonathan Dijkslag on growing and maintaining the company's edge in biotechnology and life sciences
In the latest episode of our podcast, Jonathan Dijkslag, Global Manager of Data Insights & Data Innovation at Enza Zaden, one of the world’s leading vegetable breeding companies sits down with Ties Carbo, Principal at Rewire. With over 2,000 employees and operations in 30 countries, Enza Zaden has built its success on innovation, from pioneering biotechnology in the 1980s to embracing the transformative power of Data & AI today.
Jonathan shares insights into the company’s data-driven journey, the challenges of integrating traditional expertise with modern technology, and how cultivating a culture of trust, speed, and adaptability is shaping Enza Zaden’s future. Tune in to discover how this Dutch leader is using data to revolutionize vegetable breeding and stay ahead in a competitive, innovation-driven industry.
Watch the full interview
The transcript below has been edited for clarity and length.
Ties Carbo: Thank you very much for joining this interview. Can you please introduce yourself?
Jonathan Dijkslag: I work at Enza Zaden as the global manager of Data Insights and Innovation. My mission is to make Enza Zaden the most powerful vegetable-breeding company using data.
"It's about how to bring new skills, new knowledge to people, and combine it all together to deliver real impact."
Jonathan Dijkslag
Ties Carbo: Can you tell us a little bit about your experience on working with Data & AI?
Jonathan Dijkslag: What made Enza very successful is its breeding expertise. We are working in a product-driven market and we are really good at it. Enza grew over time thanks to the expertise of our people. In the 80s, biotech came with it. And I think we were quite successful in adapting to new technologies.
Now we are running into an era where data is crucial. So I think that data is at the heart of our journey. We are a company that values our expertise. But also we are really aware of the fact that expertise comes with knowledge, and with having the right people able to leverage that knowledge in such a way that you make impact. And that's also how we look at this journey. So it's about how to bring new skills, new knowledge to people, and combine it all together to deliver real impact.
Ties Carbo: It sounds like you're in a new step of a broader journey that started decades ago. And now, of course, Data & AI is a bit more prevalent. Can you tell us a little bit more about some successes that you're having on the Data & AI front?
Jonathan Dijkslag: There are so many, but I think the biggest success of Enza is that with everything we do, we are a conscious of our choices. Over the past three to five years, we have spent quite some money on the foundation for success. So where some companies are quite stressed out because of legacy systems, we do very well in starting up the right initiatives to create solid foundations and then take the time to finalize them. And I think that is very powerful within Enza. So over the past years we have built new skills based on scalable platforms in such a way that we can bring real concrete value in almost all fields of expertise in the company.
Ties Carbo: Can you maybe give a few examples of some challenges you encountered along the way?
Jonathan Dijkslag: I think the biggest challenge is that the devil is in the details. That's not only from a data journey perspective, but also in our [functional] expertise. So the real success of Enza with data depends on really high, mature [functional] expertise combined with very high, mature expertise in data. And bridging functional experts with data experts is the biggest challenge. It has always been the biggest challenge. But for a company like Enza, it's particularly complex.
Another complexity is we have quite some people who for decades have used data heavily in their daily work. Yet the way we use data nowadays is different than compared to decades ago. So the challenge is how to value the contributions made today and at the same time challenge people to take the next step with all the opportunities we see nowadays.
"We learned the hard way that if you don't understand – or don't want to understand what our purpose is as a company, then you will probably not be successful."
Jonathan Dijkslag
Ties Carbo: I was a bit intrigued by your first response – bridging the functional expertise with the Data & AI expertise. What's your secret to doing that?
Jonathan Dijkslag: I think it really comes back to understanding the culture of your company. When I joined the company, I knew that it would take time to understand how things work today. I needed to show my respect to everything that has brought so much success to the company in order to add something to the formula.
Every company has a different culture. Within Enza, the culture is pretty much about being Dutch. Being very direct, clear, result-driven, don't complain. Just show that you have something to add. And that's what we try to do. And learn, learn and adapt. For instance, in the war on talent, we made some mistakes. We thought maybe business expertise is not always that important. But we learned the hard way that if you don't understand – or don't want to understand what our purpose is as a company, then you will probably not be successful. And that means something to the pace and the people you can attract to the company. So it’s all about understanding the existing culture, and acting on it. And sometimes that means that you need more time than you’d like to have the right people or have the right projects finalized and create the impact you want.
"We have to create a mechanism whereby we're not too busy. We have to create a change model where you can again and again adapt to new opportunities."
Jonathan Dijkslag
Ties Carbo: What advice would you give to other companies that embark on a journey like this?
Jonathan Dijkslag: This is the new normal. The complexity is the new normal. So we have to think about how we can bring every day, every week, every month, every year more and more change. And people tend to say “I'm busy, we’re busy, wait, we have to prioritize.” I think we have to rethink that model. We have to create a mechanism whereby we're not too busy. We have to create a change model where you can again and again adapt to new opportunities. And I think this is all about creating great examples. So sometimes it’s better to make fast decisions that afterwards you would rate with a seven or an eight, but you did it fast. You were very clear and you made sure that the teams work with this decision that’s rated seven, rather than thinking for a long period about the best business case or the best ROI. So I think the speed of decision-making close to the area impact. I think that that's the secret.
People talk a lot about agility. I think for me, the most important part of agility is the autonomy to operate. Combined with very focused teams and super fast decision-making and the obligation to show what you did.
Ties Carbo: How is it to work with Rewire?
Jonathan Dijkslag: For me, it's very important to work with people who are committed. I have a high level of responsibility. I like to have some autonomy as well. And to balance those things, I think it's very important to be result-driven and also to show commitment in everything you do. And what we try to do in our collaboration with Rewire is to create a commitment to results. Not only on paper, but instead both parties taking ownership of success. And we measure it concretely. That's the magic. We're really in it together and there's equality in the partnership. That's how it feels for me. So I think that that's the difference.
We work a lot with genetic data. So our challenges are very specific. Life science brings some complexity with it. And we were looking for a partner who can to help us develop the maturity to understand and work with confidence with the corresponding data. And thanks to their experience in the field of life science, they showed that they understand this as well. And that's very important because trust is not easily created. And if you show that you brought some successes in the world of life science - including genetic data - that helps to get the trust, and step into realistic cases.
Ties Carbo: Thank you.
About the authors
Jonathan Dijkslag is the Global Manager of Data Insights & Data Innovation at Enza Zaden, where he drives impactful data strategies and innovation in one of the world's leading vegetable breeding companies. With over 15 years of experience spanning data-driven transformation, business insights, and organizational change, Jon has held leadership roles at Pon Automotive, where he spearheaded transitions to data-driven decision-making and centralized business analytics functions. Passionate about aligning technology, culture, and strategic goals, Jon is dedicated to creating tangible business impact through data.
Ties Carbo is Principal at Rewire.
Allard de Boer on scaling data literacy, overcoming challenges, and building strong partnerships at Adevinta - owner of Marktplaats, Leboncoin, Infojobs and more.
In this podcast, Allard de Boer, Director of Analytics at Adevinta (a leading online classifieds which includes brands like Marktplaats, Leboncoin, mobile.de, and many more) sits down with Rewire Partner Arje Sanders to explore how the company transformed its decision-making process from intuition-based to data-driven. Allard shares insights on the challenges of scaling data and analytics across Adevinta’s diverse portfolio of brands.
Watch the full interview
The transcript below has been edited for clarity and length.
Arje Sanders: Can you please introduce yourself and tell us something about your role?
Allard de Boer: I am the director of analytics at Adevinta. Adevinta is a holding company that owns multiple classifieds brands like Marktplaats in the Netherlands, Mobile.de in Germany, Leboncoin in France, etc. My role is to scale the data and analytics across the different portfolio businesses.
Arje Sanders: How did you first hear about Rewire?
Allard de Boer: I got introduced to Rewire [then called MIcompany] six, seven years ago. We started working on the Analytics academy for Marktplaats and have worked together since.
We were talking about things like statistical significance, but actually only a few people knew what that actually meant. So we saw that there are limits to our capabilities within the organization.
Allard de Boer
Arje Sanders: Can you tell me a little about the challenges that you were facing and wanted to resolve in that collaboration? What were your team challenges?
Allard de Boer: Marktplaats is a technology company. We have enormous technology, we have a lot of data. Every solution that employees want to create has a foundation in technology. We had a lot of questions around data and analytics and every time people threw more technology at it. That ran into limitations because the people operating the technology were scarce. So we needed to scale in a different way.
What we needed to do is get more people involved in our data and analytics efforts and make sure that it was a foundational capability throughout the organization. This is when we started thinking about how to scale specific use cases further. See how we can take what we have, but then make it common throughout the whole organization and then scale specific use cases further.
For example, one problem is that we did a lot of A/B testing and experimentation. Any new feature on Marktplaats was tested through A/B testing. It was evaluated on how it performed on customer journeys, and how it performed on company revenue. This was so successful that we wanted to do more experiments. But then we ran into the limitations of how many people can look at the experimentation, and how many people understand what they're actually looking at.
We were talking about things like statistical significance, but actually only a few people knew what that actually meant. So we saw that there are limits to our capabilities within the organization. This is where we started looking for a partner that can help us to scale employee education to raise the level of literacy within our organization. That's how we came up with Rewire.
We were an organization where a lot of gut feeling decision-making was done. We then shifted to data-driven decision-making and instantly saw acceleration in our performance.
Allard de Boer
Arje Sanders: That sounds quite complex because I assume you're talking about different groups of people with different capability levels, but also over different countries. How did you approach the challenge?
Allard de Boer: Growth came very naturally to us because we had a very good model. Marktplaats was growing very quickly in the Netherlands. Then after a while, growth started to flatten out a bit. We needed to rethink how we run the organization. We moved towards customer journeys and understanding customer needs.
Understanding those needs is difficult because we're a virtual company. Seeing what customers do, understanding what they need is something we need to track digitally. This is why the data and analytics is so vital for us as a company. If you have a physical store, you can see how people move around. If your store is only online, the data and analytics is your only corridor to understanding what the customer needs are.
When we did this change at Marktplaats, people understood instantly that the data should be leading when we make decisions. We were an organization where a lot of gut feeling decision-making was done. We then shifted to data-driven decision-making and instantly saw acceleration in our performance.
Arje Sanders: I like that move from gut feeling-based decision-making to data-driven decision-making. Can you think of a moment when you thought that this is really a decision made based on data, and it's really different than what was done before?
Allard de Boer: One of the concepts we introduced was holistic testing. Any problem we solve on Marktplaats is like a Rubik's Cube. You solve one side, but then all the other sides get messed up. For example we introduced a new advertising position on our platform, and it performed really well on revenue. However, the customers really hated it. When only looking at revenue, we thought this is going well. However, if you looked at how customers liked it, you saw that over time the uptake would diminish because customer satisfaction would go down. This is an example of where we looked at performance from all angles and were able to scale this further.
After training people to know where to find the data, what the KPIs mean, how to use them, what are good practices, what are bad practices, the consumption of the data really went up, and so did the quality of the decisions.
Allard de Boer
Arje Sanders: You mentioned that in this entire transformation to become more data-driven, you needed to do something about people's capabilities. How did you approach that?
Allard de Boer: We had great sponsorship from the top. We had a visionary leader in the leadership team. Because data is so important to us as a company, everybody from the receptionist to the CEO, needs to have at least a profound understanding of the data. Within specific areas, of course, we want to go deeper and much more specific. But everybody needs to have access to the data, understand what they're looking at, and understand how to interpret the data.
Because we have so many data points and so many KPIs, you could always find a KPI that would go up. If you only focus on that one but not look at the other KPIs, you would not have the full view of what is actually happening. After training people to know where to find the data, what the KPIs mean, how to use them, what are good practices, what are bad practices, the consumption of the data really went up, and so did the quality of the decisions.
Arje Sanders: You said that you had support from the top. How important is that component?
Allard de Boer: For me, it’s vital. There's a lot of things you can do bottom up. This is where much of the innovation or the early starting of new ideas happen. However, when the leadership starts leaning in, that’s when things accelerate. Just to give you an example, I was responsible for implementing certain data governance topics a few years back. It took me months to get things on the roadmap, and up to a year to get things solved. Then we shifted the company to focus on impact and everybody had to measure their impact. Leadership started leaning in and I could get things solved within months or even weeks.
Arje Sanders: You've been collaborating with Rewire on the educational program. What do you like most about that collaboration, about this analytics university that we've developed together?
Allard de Boer: There are multiple things that really stand out for me. One is the quality of the people. The Rewire people are the best talent in the market. Also, when developing this in-house analytics academy, I was able to work with the best people within Rewire. It really helped to set up a high-quality program that instantly went well. It's also a company that oozes energy when you come in. When I go into your office, I'm always welcome. The drinks are always available at the end of the week. I think this also shows that it is a company of people working well together.
Arje Sanders: What I like a lot in those partnerships is that it comes from two sides. Not every partnership flourishes, but those that flourish, those are the ones that also open up to a real partnership. Once you have that, then you really see an accelerated collaboration. I like that a lot about that, working together.
Allard de Boer: Yes. Rewire is not just a vendor for us. You guys have been a partner for us from the start. I've always shared this as a collaborative effort. We could have never done this without Rewire. I think this is also why it's such a strong partnership over the many years.
About the authors
Allard de Boer is the Director of Global Data & Analytics at Adevinta, where he drives the scaling of data and analytics across a diverse portfolio of leading classifieds brands such as Marktplaats, Mobile.de, and Leboncoin. With over a decade of experience in data strategy, business transformation, and analytics leadership, Allard has held prominent roles, including Global Head of Analytics at eBay Classifieds Group. He is passionate about fostering data literacy, building scalable data solutions, and enabling data-driven decision-making to support business growth and innovation.
Arje Sanders is Partner at Rewire.
Joris Lindenhovius from Rabobank discusses the strategies, challenges, and successes in building data governance at the bank
In a recent interview, Joris Lindenhovius, Head of Data Management at Rabobank, and Rewire Principal Ties Carbo discuss Rabobank’s journey to establish robust data governance and foster a data-driven culture across its workforce, highlighting the technical and human elements essential for success. The discussion sheds light on the power of shared responsibility, strategic governance, and hands-on training to embed data as a core asset within a company.
Watch the full interview
The transcript below has been edited for clarity and length.
Ties Carbo: Joris, thanks for joining us on this interview. Can you please introduce yourself?
Joris Lindenhovius: I work at Rabobank and I'm the head of the data management department. I'm responsible for data management standards, having oversight of the implementation of those standards and making sure that our data is of the highest quality and always available and accessible to everyone within the bank.
We have data management as a strategic topic within Rabobank. So we report back to the managing board on its progress. Our vision is of taking stock of our most business critical reports and models, making sure that we implement our data management standards, and reporting to the managing board because it's a strategic topic – not only for us, but also for the supervisor. So scoping, focusing and monitoring our progress, because it's part of our vision.
Ties Carbo: Can you elaborate on a data-driven application or AI use case that had a significant impact?
Joris Lindenhovius: My team is a policy team. We draw up data management standards and we have the oversight bank-wide on the implementation but we're not responsible for the implementation itself. So if you look at success, it's about drawing up a master plan where you have a five-year commitment from the managing board, and a five-year budget to make that impact. So making sure that data is a strategic asset is one of our biggest successes.
Secondly, we have three levels of data governance: we have a data governance board at a strategic level, with participation of the managing board. We have a tactical committee. We also have an operational board that steers our data management program. And we are continuously refining and improving our governance. That can be seen as a success.
Data management is not only about technology, it's not only about plans, it's also about people.
Joris Lindenhovius
Data management is not only about technology, it's not only about plans, it's also about people. With the literacy team, the [GAIN-enabled] academy in place, my people deliver data training one day per week to the 42 thousand employees of the bank. We have a mandatory training, e-learning trainings. That's a huge success because it makes people aware of the fact that they're part of our data challenges.
Then we have a sponsor program that the managing board and the people under it must take to understand their position vis-à-vis our data challenges. This is also a big success. Finally, we just have to make sure that we keep on doing this and we keep on being successful in implementing our data management standards.
The biggest lesson is that you cannot do it alone. You have to share the responsibilities. Appoint data owners.
Joris Lindenhovius
Ties Carbo: What lessons could you share with others, whether banks or other companies?
Joris Lindenhovius: The biggest lesson is that you cannot do it alone. You have to share the responsibilities. Appoint data owners: make report owners or model owners responsible for the quality of the data, even though they have a limited amount of influence. But they have to know that they're responsible for it and they have to organize things. So sharing the ownership, sharing the responsibilities, I think is one of the biggest learnings. It's not just about training them, but it's also about putting them into the position – with the help of governance layers – to do their work. All of them are really busy and we're just adding new roles and responsibilities. So they have to understand what's in it for them. You teach that in the beginning and afterwards you hold them responsible for doing what they should be doing. You also help them by giving them insights, data and information to see whether we are moving in the right direction. But the biggest learning is sharing their responsibilities throughout the organization and putting people in a position to transform the organization into a data-driven organization.
Ties Carbo: How is it to work with Rewire? What stands out for you?
Joris Lindenhovius: I've been directly involved with Rewire at two companies, as part of creating a data-driven culture – or a data analytics-driven culture. I think the most important thing is that you take people away from their day-to-day job and have them focus during a full day or multi-day training on what is data, what is data analytics, what it means for you, and engage them through exercises to create that energy on this specific topic. Because before that it's mostly an opinion that they have because of the articles that they've read. By engaging them through a [data training] program you can tap into what it data is and what it means for them. That's what I was involved with at KPN and later at Rabobank – creating that culture using these training sessions. So taking employees out of their normal work – not an hour, not two hours – but for a whole day or two, put them through a program and letting them understand what data is and what it can be. That's what I think of when I think of Rewire.
About the authors
Joris Lindenhovius is an expert in data management and strategy, currently serving as Head of Global Data Management at Rabobank. With over 20 years in the field, Joris has held key leadership roles across sectors, including as Chief Data Officer at KPN, where he led the transformation of KPN’s data program and centralized data organization. He’s known for his deep expertise in data governance, data quality, and building data-driven cultures within organizations.
Ties Carbo is Principal at Rewire.
Exploring the path from AI's bold promises to practical business impact: insights from industry leader Tom Goodwin and Rewire CEO Wouter Huygen
In this exclusive interview, Tom Goodwin and Wouter Huygen dive into the complexities of integrating AI into today’s businesses, exploring the tension between transformative potential and organizational realities. They discuss the importance of balancing visionary goals with practical steps, the challenges of scaling innovation within legacy systems, and the human aspect of embracing AI. From the potential of generative AI to the importance of incremental change, Goodwin and Huygen delve into the complexities of driving meaningful progress in today’s fast-paced digital world. This conversation offers a look into the journey of bringing cutting-edge technology to life in a way that reshapes industries while staying grounded in real-world applications.
Wouter Huygen: Tom, you spend a lot of time thinking about data, AI, but also talking to industry leaders. What is the most intriguing question on your mind in this field?
Tom Goodwin: That's a big question. For me, the biggest challenge is reconciling, on the one hand, the incredible opportunities that we have and the extraordinary things that technology can do. And, on the other hand, the reality of people and organizations.
Tom Goodwin: Whenever I work with a big company, individually people are incredible. Individually people are expert, they're well-intentioned, they work hard. You put all of these people together in a company and things get really messy. Most companies have to deal with cultures that are not great with change. They have to deal with legacy technology that they can't make a business case to change. So by far the hardest and the most wonderful thing I have to do is plot this line between possibility and what's realistic. You know, what can you rethink? How big a risk can you take? How do you make the business case for this change? And that's why I'm really interested to meet you and talk to you guys, because this is what you're doing.
I'm quite often the provocateur, I go in and I say, "Couldn't the world be amazing? What if we thought this way?" And then normally I get to leave before I have to do much about it. So I'm always interested to know in your position what it's like. How do you take the possibilities of technology and turn that into a real action plan?
Wouter Huygen: It's indeed striking the right balance between staying pragmatic and keeping eyes on short- or medium-term opportunities, versus going towards that vision or dot on the horizon, right? The big promise. Because ideally you move as a company to that big promise. We can all reinvent our business with AI. But it's not easy to paint that picture, but sometimes it's possible. But then it's only a picture. And you will only get there step by step. So how do you chop it up in steps that you can realistically take? Where do you start and how do you scope it?
Typically it boils down to defining value pools with use cases and doing that in a smart way. So making a smart assessment about what is the possible or the potential impact of these use cases, how feasible they are, and then doing a common sense prioritization. But that's only the very first step. And then the next step is how do you go the whole journey from conception to MVP, to scaling it, and to changing the business along with it, right? So that it doesn't just become this new fancy thing on top of a process or on top of a business, but that it is actually integrated into your processes - and the processes themselves evolve.
Tom Goodwin: There's always this really interesting tension, I think, between the fancy and frivolous, and new and exciting technology. And actually the degree to which really profound change comes from things that are very boring. So anything you can take a photograph of gets the client's imagination. A picture of a drone looks very exciting. A picture of a robot looks very exciting. In reality quite a lot of companies can be really transformed by people using collaborative software or with a better use of microservices layers or a better way to run meetings. Quite often the most profound technology doesn't look very interesting. So I always find it quite hard to balance the sexy with the profound and the stuff that can happen quickly and looks good in the company report and the thing that may take five or ten years. For me, technical debt is a huge problem because it's very hard to get people really excited about changing the foundations of a company when often you can't see those things.
Wouter Huygen: On that, sometimes the first step is changing a process using data and maybe not even very sophisticated AI, but make some data-driven common sense decisions. That could be a first step out of which you then become more sophisticated. Do you think you need to walk before you can run, or could you run a marathon right from the start?
Tom Goodwin: It's a very good question. I think it probably depends a lot on the business itself and their culture. There are times when if you aim too far, you end up losing people. But there are times where if you're not ambitious enough, by the time things get scaled back, it becomes very incremental.
It's always quite interesting for me because I trained to be an architect rather than something in technology. And for me, physical infrastructure and company infrastructure have a very similar parallel. There are times when you look at an airport and you think rather than adding a cabin or building a new terminal, we should probably just build an airport that's brand new in a completely different place. And I find that these incremental changes - because the capital is easier to justify, because they carry less risk, they can be done more quickly, and people can get on board with more quickly – I find that they tend to be the solution that people defer to. And normally that means that people work very hard just to maintain something that's not quite as good as it can be.
The cost of doing nothing is often thought to be zero, when in fact, it's quite expensive.
Tom Goodwin
So it's interesting for me when you get a technology that's developing as rapidly as AI, because the growth curve of possibility is so rapid, it almost becomes easier to justify something which is bolder and more ambitious because you can do something with it now and the value that it adds can be so great. But it's difficult. People are not good with taking risks. People are not good with something that can't be quantified. The cost of doing nothing is often thought to be zero, when in fact, it's quite expensive. How do you motivate people to take a risk and to invest in these new ways of doing?
Wouter Huygen: Part of it is the business case. That's by far the most important thing. And it should be a business case that has a sufficient amount of realism to it. Because lofty business cases are easy. Back of the envelope calculations, we can all make them. And then it helps to have experience in actually developing these use cases all the way, up until the point they are embedded, implemented, scaled in an organization. Because then you actually learn what it takes to get there and to get the impact out of it. Just having the wheels running. Next time you are in the beginning of such a cycle it will help make a realistic estimate – not just of its theoretical potential, but how much we actually can get from it. And being able to explain that gives both the inspiration about the opportunity and sufficient tangibility around the reality of things. Those together, obviously, for an executive, is the recipe for saying, "Okay, let's do it."
Tom Goodwin: Does it limit your ability to be incredibly ambitious? What I mean by this is that it's very hard to quantify the value of doing something that's never been done before.
Wouter Huygen: It does. And it depends on the leaders. The ones that dare to take a leap and are a bit more willing to take risks, have a drive to innovate in their industry – those are the business leaders that stretch the boundaries.
Tom Goodwin: This event is obviously mainly focused on AI, but AI as a lever for accomplishing more. Are there particular elements or aspects to AI that you're most interested in personally or most inspired by?
Wouter Huygen: I'm curious by nature. I've always been curious about technology. What I like about AI is everything that doesn't work yet or is not yet very clear. Once it's clear, I tend to lose interest a little bit because then I know how it works. So this whole new wave of generative AI is actually, for me, a candy store because there are so many unknowns. There's so much stuff still to be found out.
At the same time, what I'm inspired by is not so much the technology per se, but what you can do with it. In that sense, we're shifting from what we now call traditional AI or predictive AI (which is basically machine learning, predicting quantitative values), to a whole new class, which is generative AI. I'm interested in both. This new class – GenAI – catches my attention because it has all these new promises, up until autonomous agents and autonomous organizations. But what I also wonder about is to what extent we are done with the first wave – that is, traditional or predictive AI. If I look at most organizations, they are still a long way from capturing the value from that.
Now everybody is all over the moon with GenAI. So there is, you could say, too much focus on the hype and the technology, but that's not what it is about. It is about what you do with it and how you change your organization with it. And you should not separate these AI classes. Actually, in most use cases, they blend, they work together. It's about systems thinking and how do you use different technologies to create new possibilities to innovate the way you serve your customers, or how you run your processes.
Tom Goodwin: A very interesting time, I think, because every time there's a new demo or a new launch from an AI company, it seems completely magical. But sometimes it doesn't seem that helpful. So I'm really interested to know what happens in the next year when people like you or I are comfortable enough with what this technology means to start bringing together user needs, bringing together companies' needs and then bringing together the technology and to make a much better way to do things. At the moment, it seems very pushed by Silicon Valley. It's like a metaphorical laboratory with people wearing white coats, juicing a formula. I'm fascinated to know what we're going to cook with it and what does it taste like.
Wouter Huygen: That's indeed the smoke and mirrors that I tend to talk about. And it's very hard: I read about it daily, maybe an hour per day. And even I have many open questions or can be confused. So let alone people that don't spend that much time on it. We've all seen the Klarna case. They automated 700 agents or so. And I wonder: is it actually a very sophisticated Q&A engine? A sophisticated chatbot? Or did they really integrate into the back end? Does the chatbot talk to back end systems? Does it solve problems? I don't know.
People are so quick to adopt these things that have become a UX layer. But actually, it's the system below that really matters.
Tom Goodwin
Tom Goodwin: This is the question. People are so quick to adopt these things that have become a UX layer. But actually, it's the system below that really matters. It's great to talk to a chatbot that sounds like a human, but does it actually have the power to refund me? It's great to have a chatbot that tells you if your flight's running on time, but does it let me change to a different flight? And it's fascinating because it's the stuff beneath the surface, these things can completely transform the world; they can give us better experiences than we've ever had before; and they can stop us having to phone up a call centre and wait for 30 minutes. Or they can just become another thing to get frustrated with before we have to pick up the phone and do it a different way. It's a very interesting time, I think.
Wouter Huygen: We were talking about chatbots and what is really the state of the art. And whether it's more than an interface, whether it's actually technology that is able to integrate with other systems to interact and carry out tasks. And part of that, obviously, is also the whole question around manageability and performance bounds on these agents. If you look at different companies applying this technology in a real customer facing setting, what is your view on that?
Tom Goodwin: We are very early on in this. I think companies have been very quick to embrace this because they think they can save a lot of money. So we can talk about Klarna and their apparent success so far. We could also talk about Air Canada having a hallucinating chatbot. We can talk about lawyers that are going into cases badly prepared. This brings home to me something which I'm really, really passionate about, which is that these decisions shouldn't be driven by the CFO. They should be driven by someone more like the CMO. Like technology is always a lever to get more out. And AI is an amazing lever to our humanity and our brains and our empathy. And I don't want people to put less in and then multiply it to get the same out. And I don't want people to put nothing in and get very little out. I want people to put the same energy or more into this and get even more out. So it can't be how can we have chatbots do our jobs. It should be how can technology make people who do customer service be even better at their jobs? How can it listen to conversations and suggest information to assistants? How can it automatically do the mundane work in the background? How can we keep humans in the loop? How can we do what we've always done with technology?
Technology has always made our jobs better and more valuable and more human by being a lever to us. Because of ATMs [cash dispensers] the people who were handing out money all day got to have conversations with clients and probably upsell them on a mortgage. How can we use this to make sure that we do better work rather than stuff cheaper and faster? What's your vision for AI? Rewire has been very, very quick to embrace this technology and to really understand what it means. What's your vision? How do you see everything going?
It's much more the fundamental change in terms of how organizations work, operate and how they create value. That's going to be the real change.
Wouter Huygen
Wouter Huygen: Interesting question. I think the divide between the technology and the pace of change in organizations is going to increase, unfortunately. So there's a lot of talk about the pace of change when it comes to generative AI, the fact that it will come to market faster than new technologies did in the past. There is some truth to it because the infrastructure is there. Ten years ago or so, all these algorithms already existed. You could build an XGBoost model with a flick of the switch. However, the infrastructure to implement those algorithms at scale in an organization and embed them in a process was not there yet. So there wasn't kind of a vehicle for AI to diffuse in organizations. By and large, most large organizations are there now. So there's a fertile ground for GenAI, and yes, that will go faster. But as previously discussed, it's much more the fundamental change in terms of how organizations work, operate and how they create value. That's going to be the real change. And that's still going to be hard work and will require creativity in terms of rethinking how we can deliver value to our customers, how to change our systems and operating models and all the rest of it. That's going to take time.
At the same time, the technology will rapidly evolve and there will be all kinds of new applications coming out, but that's on top of incumbent businesses. And I think that the topic du jour, when it comes to GenAI, is whether this pace of change is going to continue. Because the industry says yes. And they all go by the scaling hypothesis, which until now still seems to be valid – more compute, more data, and performance will increase. But there are also AI researchers who point to the technology in generative AI, and give evidence that its flaws are a feature of the technology. And that won't change by scaling it even more, let alone that at some point we'll run out of data. In that case, we will hit a plateau somewhere in the coming years. And is that a problem? No, not at all, because there's so much value to be created with what we got in the last few years. And how that has to be adopted by organizations and how organizations can innovate with that. So, to me, it doesn't really matter, whether we hit the plateau or not. Obviously to the industry, yes, because at some point the valuation of OpenAI will drop if that's the case. But to most organizations globally, it's irrelevant.
Watch the full interview
About the authors
Tom Goodwin is the four time #1 in “Voice in Marketing” on LinkedIn with over 725,000 followers on the platform. He currently heads up “All We Have Is Now”, a digital business transformation consultancy, working with Clients as varied as Stellantis, Merck, Bayer, and EY to rethink how they use technology. Tom hosts “The Edge” a TV series focusing on technology and innovation, and “My Wildest Prediction”, a podcast produced and distributed by Euronews. He has published the book “Digital Darwinism” with Kogan Page, and has spoken in over 100 cities across 45 countries. With a 23 year career that spans creative, PR, digital and media agencies, Tom is an industry provocateur as a columnist for the Guardian, TechCrunch and Forbes and frequent contributor to GQ, The World Economic Forum, Ad Age, Wired, Ad Week, Inc, MediaPost and Digiday. To find out more about Tom, visit www.tomgoodwin.co.
Wouter Huygen is partner and CEO at Rewire.
Why combining Retrieval-Augmented Generation with Knowledge Graphs holds transformative potential for AI.
Google it! How many times have you said that before? Whether you're settling a friendly debate or hunting for that perfect recipe, Google is our go-to digital oracle. But have you ever wondered what happens behind that simple search bar? At the heart of Google's search magic lies something called a Knowledge Graph—a web of interconnected information that's revolutionizing how AI understands our queries. And guess what? This same technology, along with RAG (Retrieval-Augmented Generation), is transforming how businesses handle their own data. In this article we’ll explore the journey from data to insight, where RAG and Knowledge Graphs are the stars of the show!
The Power of RAG: Enhancing AI's Knowledge Base
Retrieval-Augmented Generation, or RAG, is a fascinating approach that's changing the game in AI-powered information retrieval and generation. But what exactly is RAG, and why is it causing such excitement in the AI community?
At its core, RAG addresses a fundamental limitation in GenAI models like ChatGPT: their knowledge is strictly bounded by their training data. Consider this: if you ask ChatGPT's API (which doesn’t have the web search capability) about the 2024 Euro Cup winner, it can’t give you the answer as this event occurred after its last knowledge or training update. The same applies to company-specific queries like "What were our Q3 financial results?" The model inherently cannot access such private, domain-specific information because it's not part of its training data. This inability to access real-time or specialized information significantly limits the range of responses these models can generate.
RAG offers a solution by combining user prompts with relevant information retrieved from external sources, such as proprietary databases or real-time news feeds. This augmented input enables GenAI models to generate responses that are both contextually richer and up-to-date, allowing them to answer questions even when their original training data doesn't contain the necessary information. By bridging the gap between AI training and the dynamic world of information, RAG expands the scope of what GenAI can achieve, especially for use cases that demand real-time relevance or access to confidential data.
How does RAG work its magic?
Now that we've established how RAG addresses the limitations of traditional GenAI models, let’s break down the four essential steps to getting RAG to work: data preparation, retrieval, augmentation, and generation.
Like most AI systems, effective RAG is dependent on data: it requires the external data to be prepared in an accessible way that is optimized for retrieval. When a query is made, relevant information is retrieved and augmented to this query. The model finally generates a well-informed response, leveraging both its natural language capabilities and the retrieved information. Here's how these steps unfold.
1. Data Preparation: This crucial first step involves parsing raw input documents into an appropriate format (usually text) and splitting them into manageable chunks. Each text chunk is converted into a high-dimensional numerical vector that encapsulates its meaning, called an embedding. These embeddings are then stored in a vector database, often with an index for efficient retrieval.
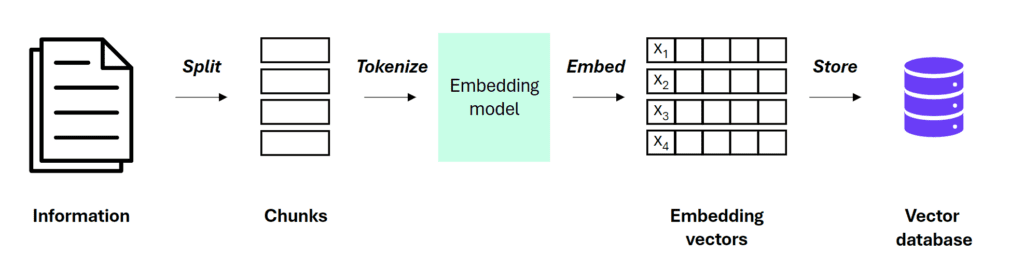
2. Retrieval: When a user asks a question, RAG embeds the query (with the same embedding model that was used to embed the original text chunks), searches the database for similar vectors (similarity search) and then retrieves the most relevant chunks.
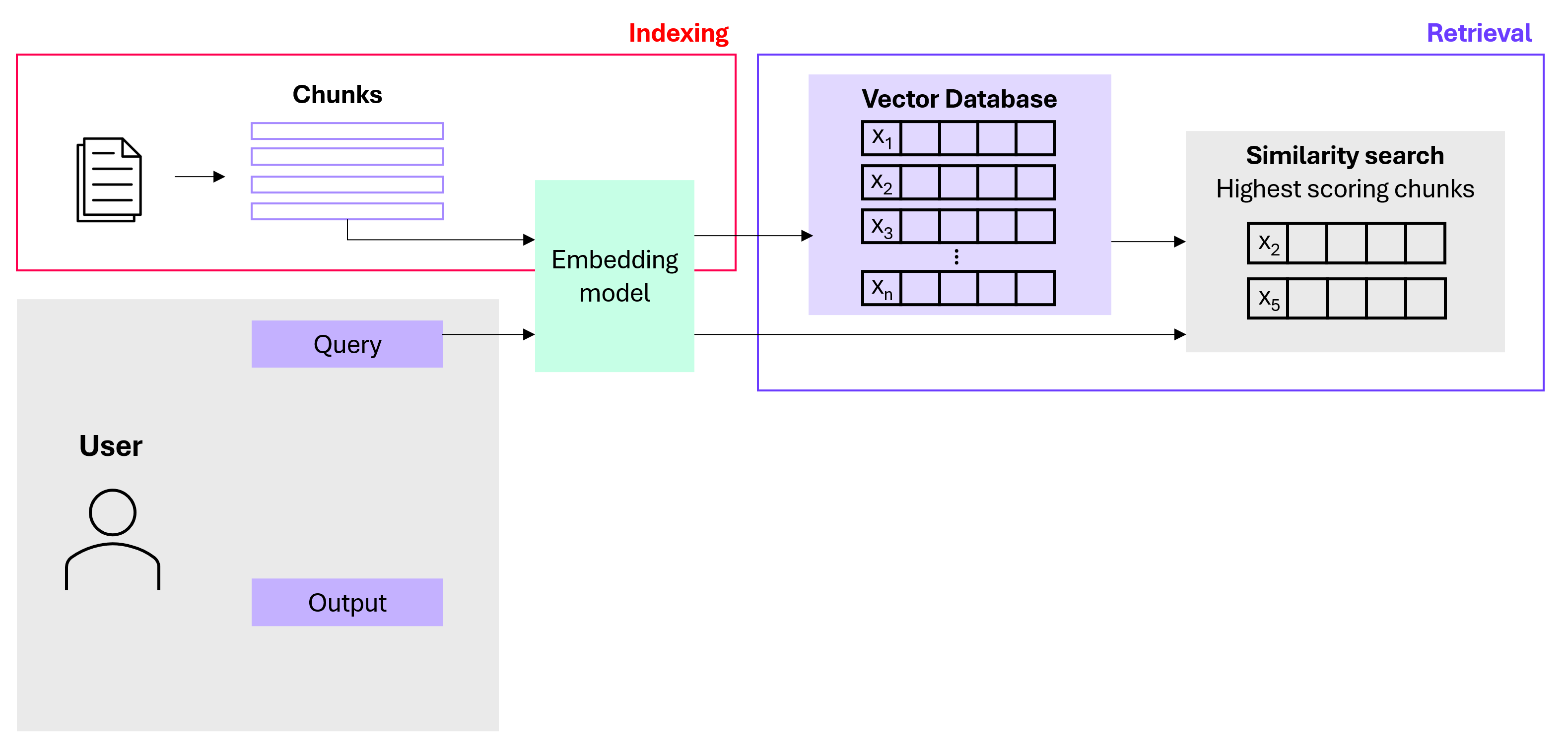
3. Augmentation: The most relevant results from the similarity search are used to augment the original prompt.
4. Generation: The augmented prompt is sent to the Large Language Model (LLM), which generates the final response.
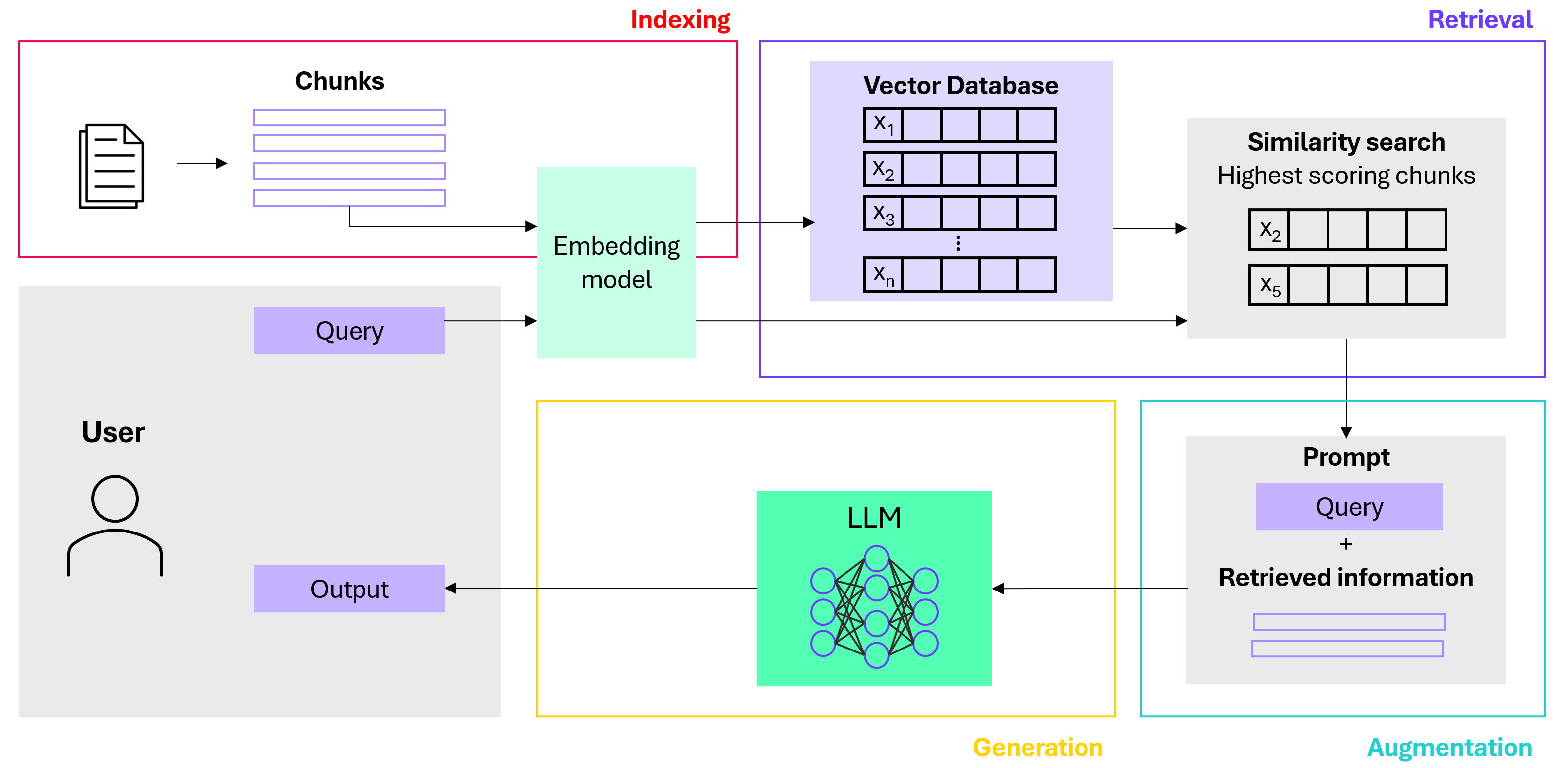
The beauty of RAG is that it doesn't fundamentally change the model's behavior or linguistic style. Instead, it enhances the model's knowledge base, allowing it to draw upon a vast repository of information to provide more informed and accurate responses.
While RAG has numerous applications, its impact on business knowledge management stands out. In organizations with 50+ employees, vital knowledge is often scattered across systems like network drives, SharePoint, S3 buckets, and various third-party platforms, making it difficult and time-consuming to retrieve essential information.
Now, imagine if finding company data was as easy as a Google search or asking ChatGPT. RAG makes this possible, offering instant, accurate access to everything from project details to financial records. By connecting disparate data sources, RAG centralizes knowledge, streamlines workflows and turns scattered information into a strategic asset that enhances productivity and decision-making.
Knowledge Graphs: Mapping the Connections
While RAG excels at retrieving relevant information, it doesn’t always capture the broader context of interconnected data. In complex business environments, where relationships between projects, departments, and clients are crucial, isolated facts alone may not tell the full story. This is where RAG falls short: it retrieves data, but it doesn’t always "understand" how these pieces fit together. Here, Knowledge Graphs (KGs) come in, mapping connections between entities to create a structured, context-rich network of relationships that deepens AI’s understanding.
How do Knowledge Graphs work?
A Knowledge Graph (KG) is a network of interconnected concepts, where nodes represent entities (e.g., people, projects, or products), and edges show the relationships between them. KGs are especially powerful because they can easily incorporate new information and relationships, making them adaptable to changing knowledge.
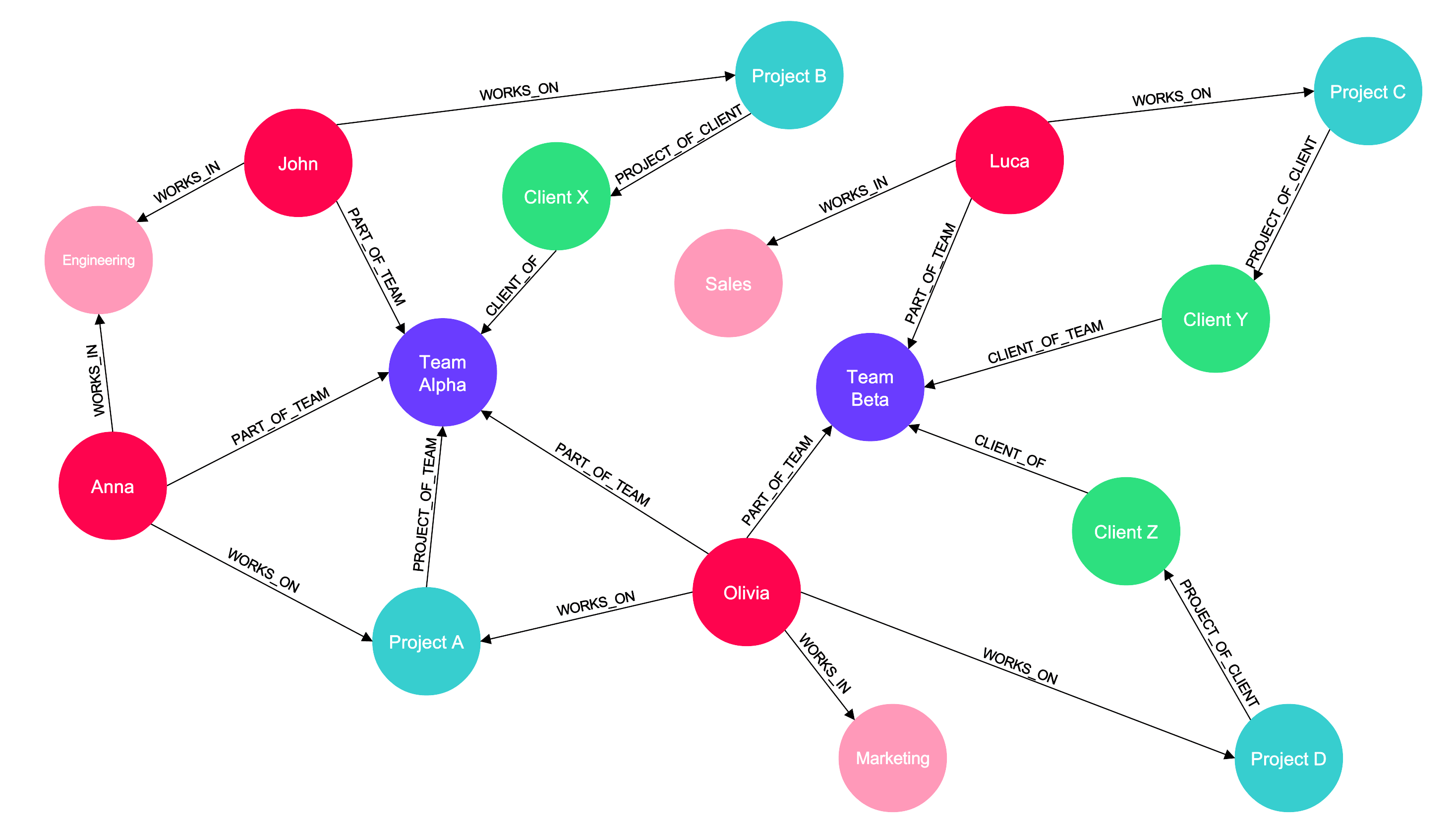
For businesses, a unified view of their data is invaluable. Knowledge Graphs organize complex relationships between employees, teams, projects, and departments, transforming scattered data into a structured network of meaningful connections. This structured approach empowers GenAI by enabling more intelligent, context-aware outputs based on deep, interconnected insights.
Imagine a GenAI-powered workflow: when a user poses a question, the system translates it into a query, perhaps leveraging a graph query language like Gremlin. The Knowledge Graph responds by surfacing relevant relationships—such as past interactions with a client or the expertise of specific team members. GenAI then synthesizes this enriched data into a clear, actionable response. Whether HR is identifying the ideal candidate for a project or sales is retrieving a client's complete history, integrating KGs into AI workflows allows businesses to extract these interconnected insights.
Bringing Knowledge Graphs and RAG Together: Introducing GraphRAG
Both RAG and Knowledge Graphs tackle a fundamental challenge in AI: enabling machines to understand and use information more like humans do. Each technology excels in different areas—RAG is particularly strong at retrieving unstructured, text-based information, while Knowledge Graphs are powerful for organizing and connecting information in meaningful ways. Notably, Knowledge Graphs can now also incorporate unstructured data processed by LLMs, allowing them to reliably retrieve and utilize information that was originally unstructured. This synergy between RAG and Knowledge Graphs creates a complementary system capable of managing diverse information types, making their integration especially valuable for internal knowledge management in businesses, where a wide range of data must be effectively utilized.
Here's how this powerful combination works:
1. Building the Knowledge Graph with RAG: We start by setting up a Knowledge Graph based on the relationships in the company's data, using RAG right from the start. This process involves chunking all internal documents and embedding these chunks. By applying similarity searches on these embeddings, RAG uncovers connections within the data, helping to shape the structure of our Knowledge Graph as it is being built.
2. Connecting Documents to the Graph: Once we have our Knowledge Graph, we connect the embeddings of the chunked documents to the corresponding end nodes. For instance, all embedded documents regarding Project A are connected to the Project A node in the graph. The result is a rich Knowledge Graph where some nodes are linked to embedded chunks of internal documents.
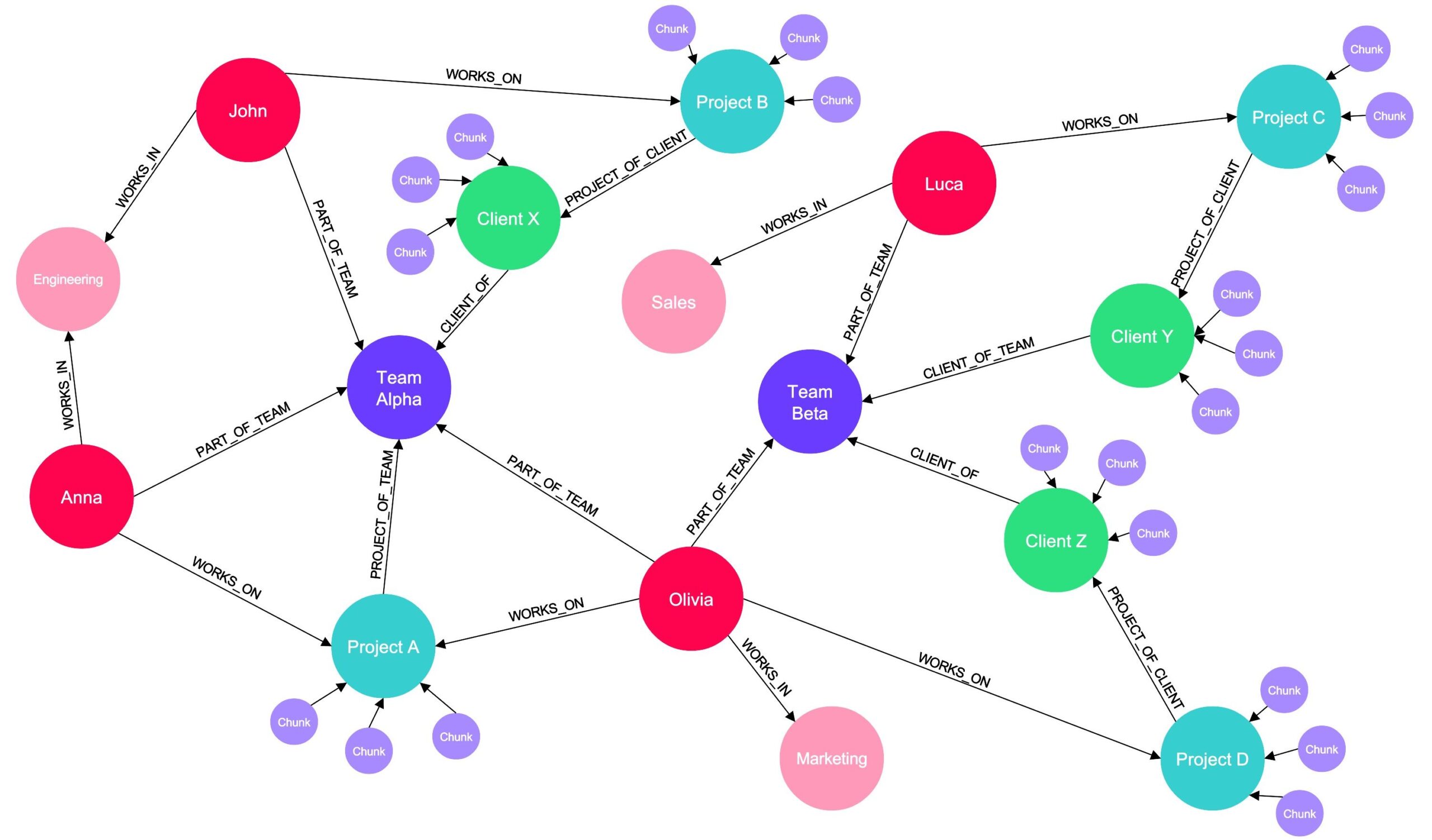
3. Leveraging RAG for Complex Queries: This is where RAG again comes into play. For questions that can be answered based purely on the Knowledge Graph structure, we can quickly provide answers. But for queries requiring detailed information from documents, we use RAG:
- We navigate to the relevant node in the Knowledge Graph (e.g., Project A).
- We retrieve all connected embeddings (e.g., all embedded chunks connected to Project A).
- We perform a similarity search between these embeddings and the user's question.
- We augment the original user prompt with the most relevant chunks (use database keys to obtain the chunks corresponding to the relevant embeddings) .
- Finally, we pass this augmented prompt to a LLM to generate a comprehensive answer.
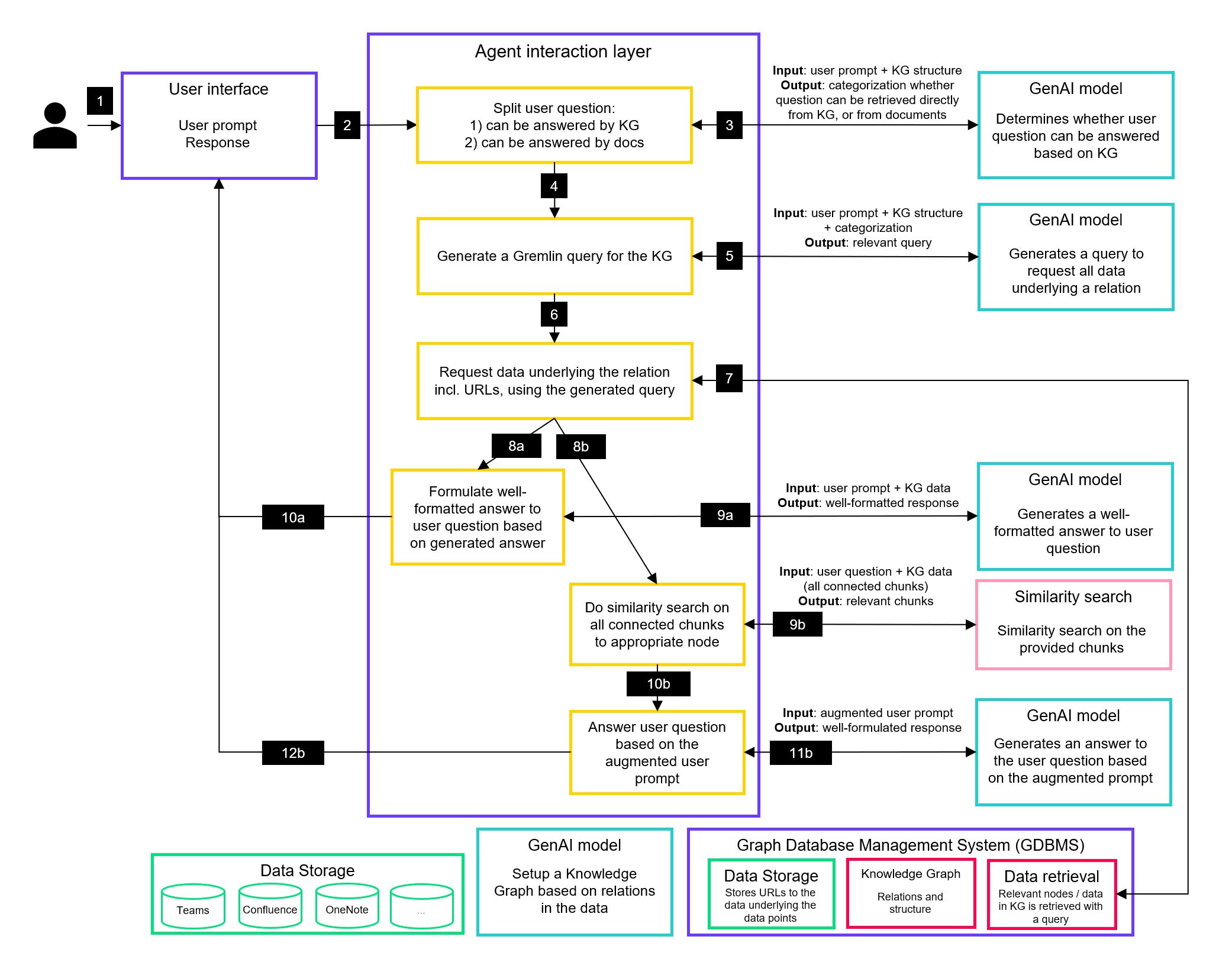
This hybrid approach combines the best of both worlds. The Knowledge Graph offers a structured overview of the company's information landscape, enabling quick responses to straightforward queries while also efficiently guiding RAG to the most relevant subset of document chunks. This significantly reduces the number of chunks involved in similarity searches, optimizing retrieval. Meanwhile, RAG excels at performing deeper, more detailed searches when necessary, providing comprehensive answers without the computational burden of scanning all documents for each query. Together, they create a more efficient, scalable, and intelligent system for handling business knowledge.
Unlocking Actionable Insights: The Future with RAG and Knowledge Graphs
Practically speaking, RAG and Knowledge Graphs revolutionize how we interact with data by making information both accessible and deeply connected. It’s like searching your company’s entire database as effortlessly as using Google, but with precise, up-to-date answers. The impact? Streamlined workflows, faster decision-making, and the discovery of connections you may not have realized were there.
The impact of combining RAG with Knowledge Graphs extends far beyond basic knowledge management, reshaping various industries through its ability to link real-time, context-aware insights with complex data structures. In customer service, this technology could enable support bots to deliver highly personalized assistance by connecting past interactions, product histories, and troubleshooting steps into a seamless, context-aware experience. The financial sector benefits from enhanced fraud detection capabilities, as GraphRAG can map intricate transactional relationships and retrieve specific records for thorough investigation of suspicious patterns. Additionally, healthcare organizations can use the technology's potential to revolutionize patient care by creating comprehensive connections between diagnoses, treatments, and real-time medical records, while simultaneously matching patients with relevant clinical trials based on their detailed medical histories. In the terms of supply chain management, GraphRAG can empower teams with real-time disruption alerts and relationship mapping among suppliers and inventory, enabling more agile responses to sudden changes. And as a last example, market intelligence teams may gain a significant advantage through dynamic insights that link competitor data, emerging trends, and current information, facilitating proactive strategic planning.
By combining retrieval and relationship mapping, RAG and Knowledge Graphs turn complex data into actionable insights—making it easier than ever to find exactly what you need, when you need it.
The Challenge of Building GraphRAG: Unlocking Data’s Potential with Precision and Scalability
While combining RAG with KGs holds transformative potential, implementing this hybrid solution introduces significant technical and organizational challenges. The core complexity lies in seamlessly integrating two sophisticated systems—each with unique infrastructures, requirements, and limitations—into a unified, scalable architecture that can evolve with growing data demands. Other key challenges include:
- Data Consistency and Integration: Consolidating data from multiple sources like SharePoint, S3, and internal databases requires a robust integration framework to maintain cross-platform consistency, data integrity, and efficient real-time updates with minimal latency.
- Graph Structure and Adaptability: Designing a Knowledge Graph that accurately models an organization’s data relationships requires a deep understanding of interconnected entities, processes, and history. This graph must be adaptable to incorporate new data and evolving relationships, necessitating rigorous planning and proactive maintenance.
- System Optimization for Real-Time Performance: For large-scale deployments, the system must support complex queries with minimal latency. Achieving this balance requires an optimized allocation of computational resources to ensure high performance, especially during real-time retrieval and similarity searches.
- Security and Access Control: Integrating RAG with Knowledge Graphs requires careful handling of sensitive data, including strong access controls, clear permission settings, and regulatory compliance to keep proprietary information secure and private. For example, a knowledge management system should restrict access to sensitive files—such as confidential financial reports or HR documents—so that employees see only information they are authorized to access.
By addressing these challenges through a structured, security-focused approach, organizations can unlock the full potential of their data, building a scalable foundation for advanced insights and innovation.
This article was written by Anna Thoolen, Working Student, Mirte Pruppers, Data Scientist, and Simon Koolstra, Principal (30 Oct 2024).
From chess-playing robots to a future beyond our control: Prof Stefan Leijnen discusses the challenges of AI and its evolution
Stefan’s Leijnen experience spans cutting-edge AI research and public policy. As professor in applied AI, he focuses on machine learning, generative AI, and the emergence of artificial sentience. As the lead for EU affairs for the Dutch National Growth Fund project AiNed, Stefan plays a pivotal role in defining public policy that promotes innovation while protecting citizens’ rights.
In this captivating presentation, Stefan takes us on a journey through 250 years of artificial intelligence, from a chess-playing robot in 1770 to the modern complexities of machine learning. With thought-provoking anecdotes he draws parallels between the past and the ethical challenges we face today. As the lead in EU AI policy, Stefan unpacks how AI is reshaping industries, from Netflix’s algorithms to self-driving cars, and why we need to prepare for its profound societal impacts.
Below we summarize his insights as a series of lessons and share the full video presentation and its transcript.
Prepare to rethink what you know about AI and its future.
Lesson 1. AI behaviour is underpinned by models that cannot be understood by humans
10 years ago, Netflix asked the following question: “How do we know that the categories that we designed with our limited capacity as humans are the best predictors for your viewing preferences? We categorize people according to those labels, but those might not be the best labels. So let’s reverse engineer things: now that we have all this data, let’s decide what categories are the best predictors for our viewership.” And they did that. And so they come up with 50 dimensions or 50 labels, all generated by the computer, by AI. And 20 of them made a lot of sense: gender, age, etc. But for 30 of those 50 labels, you could not identify the category. That means that the machine uncovered a quality among people that we don’t have a word for. For Netflix this was great because it meant they now had 30 more predictors. But on the other hand, it’s a huge problem. Because now if you want to change something in those labels or you want to change something in the way that you use the model, you no longer understand what you’re dealing with.
Watch the video clip.
Lesson 2. AI’s versatility can lead to hidden – and very hard – ethical problems
Let’s say the camera of a self-driving car spots something and there’s a 99% chance that it is just a leaf blowing by, and a 1% chance that it’s a child crossing the street. Do you break? Of course, you would break in a 1% chance. But now let’s lower the chance to 0.1% or 0.01%. At what point do you decide to break?
The point, of course, is that we never make that decision as humans. But with rule-based programs, you have to make that decision. So it becomes an ethical problem. And these kind of ethical problems are much more difficult to solve than technological problems. Because who’s going to answer that? Who’s going to give you this number? It’s not the programmer. The programmer will go to the manager or to the CEO and they will go to the legal division or to the insurer or to the legislator. And nobody’s willing to provide an answer. For moral reasons (and for insurance reasons), it’s very difficult to solve this problem. Now, of course, nowadays there’s a different approach: just gather the data and calculate the probability of breaking that humans have. But in doing so, you have moved the ethical challenge under the rug. But it’s still there. So don’t get fooled by those strategies.
Watch the video clip.
Lesson 3. AI impact is unpredictable. But its impact won’t be just technological. It will be societal, economical, and likely political
There are other systems technologies like AI. We have the computer, we have the internet, we have the steam engine and electricity. And if you think about the steam engine, when it was first discovered, nobody had a clue of the implications of this technology 10, 20 or 30 years down the line. The first steam engines were used to automate factories. So instead of people working on benches close to each other, the whole workforce was designed along the axis of this steam engine so everything would be mechanically automated. This meant a lot of changes to the workforce. It meant that work could go for hours on end, even in the evenings and in the weekends. That led to a lot of societal changes. So labor forces emerged, you had unions, you had new ideologies popping up. The steam engine also became a lot smaller. You got the steam engine on railways. Railways meant completely different ways of warfare, economy, diplomacy. The world got a lot smaller. This all happened in the time span of several decades. We will see similar effects that are completely unpredictable as AI gets rolled out in the next couple of decades. Most of these effects of the steam engine were not technological. They were societal, economical, sometimes political. So it’s also good to be aware of this when it comes to AI.
Watch the video clip.
Lesson 4. The interfaces with AI will evolve in ways we do not yet anticipate.
The AI that we know now is very primitive. Because what we see today in AI is a very old interface. With ChatGPT, it’s a text command prompt. When the first car was invented, it was a horseless carriage. When the first TV was invented, it was essentially radio programming with an image glued on top of it. Now, for most of you who have been following the news, you already see that the interfaces are developing very rapidly. So you’ll get voice interfaces, you’ll get a lot more personalization with AI. This is a clear trend.
Watch the video clip.
Watch the full video.
(Full transcript below.)
Full transcript.
The transcript has been edited for brevity and clarity.
Prof Leijnen: Does anybody recognize this robot that I have behind me? Not many people. Well, that’s not surprising because it’s a very old robot. This robot was built in the year 1770, so over 250 years ago. And this robot can play chess.
And it was not just any chess-playing robot. It was actually an excellent chess-playing robot. He won most games. And as you can imagine at that time, it was a celebrity. This robot played against Benjamin Franklin. It played against emperors and kings. It also played a game against Napoleon Bonaparte. We know what happened because there were witnesses there. In fact, Napoleon being the smart man that he is, he decided to play a move in chess that’s not allowed just to see the robot’s reaction. What the robot did is it took the piece that Napoleon moved and put it back in its original position.
Napoleon being inventive, did the same illegal move again. Then the robot took the piece and put it beside the board as though it’s no longer in the game. Napoleon tried a third time and then the robot wiped all the pieces off the board and decided that the game was over, to the amusement of the spectators. Then they set up the pieces again, played another game and Napoleon lost. Well, to me, this is really intelligence.
You might think or not think that this is artificial intelligence. If you think this is not artificial, you’re right. Because there’s this little cabinet which has like magnets and strings and there was a very small person that could fit inside this cabinet and who was very good at playing chess. And of course, he is the person who played the game. People only found out about 80 years after the facts when the plans were revealed by the son of von Kempelen.
Now, there was another person who played against this robot and his name was Charles Babbage. And Charles Babbage is the inventor of this machine, the analytical engine. And it’s considered by many to be the first computer in the world. It was able to calculate logarithms. Interestingly, Babbage played against the robot that you just saw. He also lost in 18 turns. But I like to imagine that Babbage must have been thinking how does this robot work, what’s going on inside.
As some of you may know, a computer actually beat the world champion in chess, Gary Kasparov in 1997. So you could say in this story spanning 250 years is a nice story arc. Because now we do have AI that can play and win chess, which was the original point of the chess-playing robots. So we’re done. We’re actually done with AI. We have AI now. The future is here. But at the same time, we’re not done at all. Because now we have this AI and we don’t know how to use it. And we don’t know how to develop it further.
There is a nice example from Netflix, the streaming company. They collect a lot of data. They have your age, gender, postal code, maybe your income. They know things about you. And then based on those categories, they try to predict with machine learning what type of series and movies you like to watch. And this is essentially their business model. Now, 10 years ago they asked the following question: “How do we know that the categories that we designed with our limited capacity as humans are the best predictors for your viewing preferences? We categorize people according to those labels, but those might not be the best labels. So let’s reverse engineer this machine learning algorithm. And now that we have all this data, let’s decide what categories are the best predictors for your viewership.”
And they did that. And they came up with 50 dimensions or 50 labels that you could attach to a viewer, all generated by the computer, by AI. And 20 of them made a lot of sense. So you would see men here, women there. You would see an age distribution. And there was very clear preferences in viewership. Of course, not completely uniform, but you could identify the categories and you could attach a label to them.
Now for 30 of those 50 labels, you could not identify the category. For example, for one of the 30 categories, you would see people on the left side, people on the right side. On the left side, they had a strong preference for the movie “American Beauty.” And on the right side, they had a strong preference for X on the beach. And nobody had any clue what discerned the group on the left from the group on the right. So that means that there was a quality in those groups of people that we don’t have a word for. We don’t know how to understand that. Which for Netflix was great because it means they had now 30 more predictors they could use to do good predictions. But on the other hand, it’s a huge problem. Because now if you want to change something in those labels or you want to change something in the way that you use the model, you no longer understand what you’re dealing with.
And this is essentially the topic of what I am talking about today. How do you manage something you can’t comprehend? Because essentially that’s what AI is. And this is not just a problem for companies implementing AI. We all know plenty of examples of AI going wrong. And when it goes wrong, it tends to go wrong quite deeply. Like in this case, if you ask AI to provide you with an image of a salmon, the AI is not wrong. Statistically speaking, it is the most likely image of a salmon you’ll find on the internet. But of course we know that this is not what was expected. And this is not just a bug. It’s a feature of AI.
I teach AI, I teach students how to program AI systems and machine learning systems. If I ask my students to come up with a program, without using machine learning or AI, that filters out the dogs from the cakes in these kind of images. It will be very difficult because it’s very hard to come up with a rule set that can discern A from B. At the same time, we know that for AI, machine learning, this is a very easy task. And that’s because the AI programs itself. Or in other words, AI can come up with a model that is so complex that we don’t understand how it works anymore, but it still produces the outcome that we’re looking for. In this case, a category classifier.
And that’s great because those very complex models allow us to build systems of infinite complexity. There’s no boundary to the complexity of the model. Just the data that you use, the computing power that you use, the fitness function that you use, but those things we can collect. But it’s also terrible because we don’t know how to deal with this complexity anymore. It’s beyond our human comprehension.
Now, about 12, 13 years ago, I was in Mountain View at Google. They had a self-driving car division. The head of their self-driving car division explains the following problem to us. He said: “We have to deal with all kinds of technical challenges. But what do you think our most difficult challenge is?” Now, this was a room full of engineers. So they said, “Well, the steering or locating yourself on the street or how do I do image segmentation?” He said, “No, you’re all wrong. Those are all technical problems that can be solved. There’s a much deeper underlying problem here. And that’s the problem of when do I break?”
Let’s say the camera spots something and there’s a 99% chance that it’s a leaf blowing by. And it’s a 1% chance that it’s a child crossing the street. Do you break? Well, of course, you would break in a 1% chance. But now we lower the chance to 0.1 or 0.01. At what point do you decide to break? The point, of course, is that we never make that decision as humans. But when you program a system like that, you have to make a decision because it’s rule-based. So you have to say if the probability is below this and that, then I break. So it becomes an ethical problem. And these kinds of ethical problems are much more difficult to solve than technological problems. Because who’s going to answer that? Who’s going to give you this number? It’s not the programmer. The programmer will go to the manager or to the CEO and they will go to the legal division or to the insurer or to the legislator. And nobody’s willing to provide an answer. For moral reasons, also for insurance reasons, it’s very difficult to solve this problem, he said. Now, nowadays they have a different approach. They just gather the data and they say based on this data, this is the probability of breaking that humans have. And so they moved the ethical challenge under the rug. But it’s still there. Don’t get fooled by those strategies.
The examples I showed, of Netflix and Google, are from tech companies. But you see it everywhere. We also know that AI is going to play a major role in healthcare in the future. Not just in medicine, but also in caring for the elderly, for monitoring, for prevention, etc. This raises lots of ethical questions. Is this desirable? Here we see a woman who needs care. There’s no care for her. This is from the documentary “Still Alice”. And there’s this robot companion taking care of her, mental care. Is this what we want or is this not what we want? Again, it’s not a technical question. It’s a moral question.
In the last 10, 15 years and in the foreseeable future, AI has moved from the lab to society. ChatGPT is adopted at a much higher rate than most companies know. A large percentage of employees use ChatGPT. But if you ask company CEOs, they probably mention a lower number because many employees use these kind of tools without the company knowing it. We know that a majority of jobs in the Netherlands will be affected by AI, either by full of partial displacement, or AI complementing their work. And we also know that there are enormous opportunities in terms of automation. So on the one hand, it’s very difficult to manage such technology, not just its bugs, but its intrinsic properties. On the other hand, it provides enormous promises for problems that we don’t know how else to solve.
So it’s wise to take a step back and think more deeply and more long-term about the effects of this technology – before we start thinking about how to innovate and how to regulate the technology. What helps us is looking back a little bit. There are other systems technologies like AI. We have the computer, we have the internet, we have the steam engine and electricity. And if you think about the steam engine, when it was first discovered, nobody had a clue of the implications of this technology 10, 20 or 30 years down the line. The first steam engines were used to automate factories. Instead of people working on benches close to each other so they could talk, the whole workforce was designed along the axis of this steam engine so everything would be mechanically automated. This meant a lot of changes to the workforce. It meant that work could go on hour after hour, even in the evenings and in the weekends because now you have this machine, and you want to keep using it. That led to societal changes. You had labor forces, you had unions, you had new ideologies popping up. The steam engine also became a lot smaller. You got the steam engine on railways. Railways meant completely different ways of warfare, economy, diplomacy. The world got a lot smaller. This all happened in the time span of several decades but we will see similar effects that are completely unpredictable as AI gets rolled out in the next couple of decades. Most of these effects of the steam engine were not technological. They were societal, economical, sometimes political. So it’s also good to be aware of this when it comes to AI.
A second element of AI is the speed at which it develops. I’ve been giving talks about artificial creativity for about 10 years now and 10, 8 years ago, it was very easy for me to create a talk. I could just show people this image and then I would say, this cat does not exist and people would be taken aback. This was the highlight of my presentation. Now I show you this image and nobody raises an eyebrow. And then two years later, I had to show this image. Again, I see no reaction from you. I don’t expect any reaction, by the way. But it shows just how fast it goes and how quickly we adopt and get used to these kind of tools. And it also raises the question: given what was achieved in the past 8 years, where will we be in 25 years from now? You can, of course, apply AI in completely new different fields, similar as was done with the steam engine: creating new materials, coming up with new inventions, new types of engineering. We already know that AI has a major role to play in creativity and in coding.
We also know that the AI that we know now is very primitive. I’ll be giving another speech in 10 years and the audience won’t be taken aback by anything. Because what we see today is AI with a very old interface. At ChatGPT, it’s the interface of the internet [i.e. a text command prompt], of the previous system technology. And that’s always been the case. When the first car was invented, it was a horseless carriage. When the first TV was invented, it was essentially radio programming with an image added to it. And now what we have with AI is an internet browser with an AI model behind it. And for most of you who have been following the news, you see that the interfaces are developing very rapidly. You’ll get voice interfaces, you’ll get a lot more personalization with AI. This is also a trend that’s very clear.
So we talked a bit about the history, we talked a bit about the pace of development, about the complexity being a feature, not a bug. We can also dive a little bit more into the technology itself. This is a graph I designed five years ago with a student of mine. It’s called the Neural Network Zoo. And what you see is from the top left, all the way to the bottom right, is the evolution of neural network architectures. Interestingly, at the bottom right, this is called the transformer architecture. Essentially, the evolution stopped and most AI that you hear about nowadays, most AI developed at Microsoft and Google and OpenAI and others are based on this transformer architecture. So there was this Cambrian explosion of architectures, and then suddenly it converged.
Until five years or so AI models were proliferating. Now they’re also converging. Nowadays, we talk about OpenAI’s GPT, we talk about Google’s Gemini, we talk about Meta’s LAMA, Mistral. There aren’t that many models. So not just the technology has been locking in, but the models themselves as well. So you see huge conversions into only a very limited set of players and models. And this is of course due to the scaling laws. It becomes very difficult to play in this game. But it’s very interesting that on the one hand you have a convergence to a limited set of models in a limited set of companies. And on the other hand, you have this emergence of new functionalities coming out of these large scale models. So they surprise us all the time, but they’re only a very limited set of models that are able to surprise us. And these developments, these trends, all inform the way that we regulate this technology.
This is currently how the European Union thinks about regulating this technology. You have four categories. (1) A minimal risk category where there’s not much or hardly any legislation. (2) A limited risk. For example, if I interact with a chatbot, I have to know I’m interacting with a chatbot and not a human. The AI has to be transparent. (3) A high risk category, where there will be all kinds of ethical checks around, let’s say toys or healthcare or anything that has a real risk for consumers or citizens or society. (4) Unacceptable risk, which is AI systems that can subconsciously influence you, do social scoring, etc. Those will all be forbidden under new legislation (the EU AI Act).
I’ll end the presentation with this final quote, because I think this is essentially where we are right now: “The real problem of humanity is the following: we have paleolithic emotions, medieval institutions and with AI, god-like technology.” (E.O. Wilson).
The 2024 Nobel Prizes in Physics and Chemistry put the spotlight on AI. While the Physics laureates, John Hopfield and Geoffrey Hinton, contributed to its theoretical foundations, two of the three Chemistry laureates – specifically, Demis Hassabis and John Jumper – were rewarded for putting it into use.
John Hopfield developed the Hopfield network in 1982, a form of recurrent artificial neural network that can store and retrieve patterns, mimicking how human memory works. It operates by processing and recognizing patterns even when presented with incomplete or distorted data. His work was significant because it helped bridge the gap between biology and computer science, showing how computational systems could simulate the way the human brain stores and retrieves information.
Geoffrey Hinton co-invented the Boltzmann Machines, a type of neural network that played an important role in understanding how networks can be trained to discover patterns in data. He also popularized the use of backpropagation, an algorithm for training multi-layer neural networks, which considerably improved their capacity to learn complex patterns. Hinton’s contributions ultimately led to AI systems like GPT (Generative Pre-trained Transformers), which underpins ChatGPT, and AlphaFold the AI program that earned Demis Hassabis and John Jumper their Nobel prize in Chemistry.
AlphaFold solved one of biology’s greatest challenges: accurately predicting the 3D structure of proteins from their amino acid sequences. This problem had stumped scientists for decades, as protein folding is essential to understanding how proteins function, which is crucial for drug discovery, disease research, and biotechnology. AlphaFold’s predictions were so accurate that they matched experimental results with near-perfect precision, revolutionizing the field of biology. This breakthrough has wide-ranging implications for medicine and has already begun to accelerate research into diseases, drug discovery, and bioengineering.
Towards AI-driven disruption of traditional business models
Beyond the world of academia and frontier research, the AI techniques developed by the 2024 laureates are permeating the business world too. For one, the capabilities to analyse, identify patterns, and make sense of vast datasets, particularly unstructured data, rely at least partially on them.
From supply chain optimization to consumer behaviour analysis, AI holds the promise of making data-driven decisions faster, and automating a growing range of tasks. Large companies have already launched initiatives to capitalize on this, with some notable successes. Witness the case of a telecom company that generated an ROI 2.5x higher than average thanks to the judicious use of AI; or the case of an energy provider that delivered savings for consumers while increasing its own revenues; or this Supply Chain example that minimized waste and lost sales, while reducing the need for manual intervention at store level. These cases are no exceptions. Increasingly, the deployment of advanced algorithms and data management techniques play a central role in gaining competitive advantage.
Ultimately, AI ability to make sense of vast quantities of data will accelerate innovation and paves the way for new business models that will disrupt existing ones. From biotech to finance and manufacturing, the possibilities are endless, and all industries will eventually be impacted. More prosaically, the breakthroughs of the 2024 Nobel laureates herald an era when AI is not just a futuristic concept, but a key driver of competitiveness right now.
Technology and innovation expert Tom Goodwin on the merits of GenAI and how to leverage its potential.
During Rewire LIVE, we had the pleasure of hosting Tom Goodwin, a friend of Rewire and pragmatic futurist and transformation expert who advises Fortune 500 companies on emerging technologies such as GenAI. Over the past 20 years, he has studied the impact of new technology, new consumer behaviors and the changing rules of business, which makes him uniquely suited to understand the significance of GenAI today.
At the core of Tom’s thinking lies a question that all leaders should ponder: if, knowing everything you know now, were to build your company from scratch, what would it look like? At times counter-intuitive, Tom’s insights, steeped in history, provide valuable clues to answer this question. In this article, we share a handful of them.
INSIGHT 1: Technology revolution happens in two stages. In the first stage we add to what was done before. In the second stage we rethink. That’s when the revolution really happens.
Tom’s insight is derived from the Perez Framework, developed by Carlota Perez, a scholar specialized in technology and socio-economic development. The framework – based on the analysis of all the major technological revolutions since the industrial revolution – stipulates that technological revolutions first go through an installation phase, then a deployment stage. In the installation phase, the technology comes to market and the supporting infrastructure is built. In the deployment phase, society fully adopts the technology. (The transition between the two phases is typically marked by a financial crash and a recovery.)
During the first phase, there’s a frenzy – not dissimilar to the hype that currently surrounds GenAI. Everyone jumps on the technology, everyone talks about it. However, nothing profound really changes. For the most part, the technology only adds to the existing ways of doing things. In contrast, during the second stage, people finally make sense of the technology and use it to rethink the way things are done. That’s when the value is unleashed.
Take electricity as an example. In the first stage, electricity brought the electric iron, the light, the fan, the oven. These were all things that existed before. In the second stage, truly revolutionary innovations emerged: the radio, the TV, the telephone, the microwave, the microwave dinner, factories that operate 24/7, and so on. The second stage required a completely different mindset vis-à-vis what could do be done and how people would behave.
This begs the question: what will be the second stage of GenAI – and more broadly AI – be? What will be the telephone, radio, microwave for AI? Tom’s assertion here is that the degree of transformation is less about how exciting that technology is, and it’s much more about how deeply you change. Better AI will be about systems that are completely rethought and deep integrations, rather than UI patches.
Watch the video clip.
INSIGHT 2: Having category expertise, knowing how to make money, having relationships, and having staff who really know what they’re doing is probably more important than technology expertise.
Across many industries, battle lines are drawn between large traditional companies that have been around for a long time and the digitally-enabled, tech first, mobile-centric startup types. Think Airbnb vs Marriott, Tesla vs. BMW, SpaceX vs NASA, and so on.
The question is who’s going to win. Is it the digitally native companies who have created themselves for the modern era? Or is it the traditional companies that have been around for a long time? Put another way, is it easiest to be a tech company and learn how to make money in your target industry? Or be a big company who already knows how to make money but must now understand what a technology means and adapt accordingly?
Up until recently, the assumption was that the tech companies would win the battle. This proved true for a while: Netflix vs. Blockbusters, Apple vs. Nokia, etc. The assumption was that this would carry on. Understanding the technology was more important than understanding the category.
Tom’s observation is that in the past four years, these assumptions have been challenged. For example, traditional banks have got really good at understanding technology. Neobanks might be good at getting millennials to share the cost of a pizza, but they’re not that good at making money. So there’s this slow realisation that maybe digital-first tech companies are not going to win – because big companies are getting pretty good at change.
Taking a step back, it seems that the narrative of disrupt or die isn’t always true: a lot of the rules of business have not changed; incumbents just need to get a bit more excited about technology. Ultimately, having category expertise, knowing how to make money, having relationships, and having staff who really know what they’re doing is probably more important than tech expertise.
Watch the video clip.
INSIGHT 3: The AI craze is enabling a more flexible investment climate. This is an incentive for leaders to be bold.
Generative AI has spurn heated debates about the evolution of AI and divided experts and observers into two opposing groups: the AI cheerleaders and the sceptics. The former believe that AI is going to change everything immediately. The latter think that it’s a bubble.
History is littered with innovations that went nowhere. A handful of them however proved to be transformational – if in the long run. Only time will tell which group GenAI will join. In the meantime, there’s a growing realization that significant investment may be required to make meaningful steps with AI, hence a more flexible climate for capex – which is an incentive for leaders to be bold.
Tom’s insight reflects this situation: change is hard and expensive, and so regardless of one’s position in the debate, GenAI provides a unique window of opportunity to get the investor that you wouldn’t normally get. It is an amazing time to have an audience who normally wouldn’t listen to you.
Conclusion
These were but a handful of the many insights that Tom shared with us during Rewire LIVE. Taking a step back, it is clear that we are far from having realized the full value of GenAI – and, more broadly, AI. In the words of Tom, AI is a chance to dream really big and leave your mark on the world. It is yours for grab.
About Tom Goodwin
Tom Goodwin is the four time #1 in “Voice in Marketing” on LinkedIn with over 725,000 followers on the platform. He currently heads up “All We Have Is Now”, a digital business transformation consultancy, working with Clients as varied as Stellantis, Merck, Bayer, and EY to rethink how they use technology.
Tom hosts “The Edge” a TV series focusing on technology and innovation, and “My Wildest Prediction”, a podcast produced and distributed by Euronews. He has published the book “Digital Darwinism” with Kogan Page, and has spoken in over 100 cities across 45 countries.
With a 23 year career that spans creative, PR, digital and media agencies, Tom is an industry provocateur as a columnist for the Guardian, TechCrunch and Forbes and frequent contributor to GQ, The World Economic Forum, Ad Age, Wired, Ad Week, Inc, MediaPost and Digiday.
To find out more about Tom, visit www.tomgoodwin.co
Rewire CEO Wouter Huygen reviews the arguments for and against GenAI heralding the next industrial revolution, and how business leaders should prepare.
Is generative AI under- or overhyped? Is it all smoke and mirrors, or is it the beginning of a new industrial revolution? How should business leaders respond? Should they rush to adopt it or should they adopt a wait-and-see approach?
Finding clear-cut answers to these questions is a challenge for most. Experts in the field are equally divided between the cheerleaders and the skeptics, which adds to the apparent subjectivity of the debate.
The GenAI cheerleaders can point to the fact that performance benchmarks keep being beaten. Here the underlying assumption is the “AI Scaling Hypothesis”. That is, as long as we throw in more data and computing power, we’ll make progress. Moreover, the infrastructure required for GenAI at scale is already there: an abundance of cloud-based data and software; the ability to interact with the technology using natural language. Thus, innovation cycles have become shorter and faster.
On the other hand, GenAI skeptics make the following points: first, the limitations of GenAI are not bugs, they’re features. They’re inherent to the way the technology works. Second, GenAI lacks real world understanding. Third, LLMs demonstrate diminishing returns. In short, there are hard limits to the capabilities of GenAI.
The lessons of History indicate that while there might be some overhype around GenAI, the impact could be profound – in the long run. Leaders should therefore develop their own understanding of GenAI and use it to define their vision. Shaping the future is a long-term game that starts today.
Watch the video (full transcript below).
The transcript has been edited for clarity and length.
Generative AI: the new magic lantern?
Anyone recognizes this? If you look closely, not much has changed since. Because this is a basic slide projector. It’s the Magic Lantern, invented around 1600. But it was not only used as a slide projector. It was also used by charlatans, magicians, people entertaining audiences to create illusions. This is the origin of the saying “smoke and mirrors”. Because they used smoke and mirrors with the Magic Lantern to create live projections in the air, in the smoke. So the Magic Lantern became much more than a slide projector – actually a way of creating illusions that were by definition not real.
You could say that Artificial Intelligence is today’s Magic Lantern. We’ve all seen images of Sora, OpenAI’s video production tool. And if you look at OpenAI’s website, they claim that they’re not working on video production. They actually intend to model the physical world. That’s a very big deal if that is true. Obviously it’s not true. At least I think I’m one of the more sceptical ones. But those are the claims being made. If we can actually use these models to model the physical world, that’s a big step towards artificial general intelligence.
Is GenAI overhyped? Reviewing the arguments for and against
If AI is today’s Magic Lantern, it begs the question, where are the smoke and where are the mirrors? And people who lead organizations should ponder a few questions: How good are AI capabilities today? Is AI overhyped? What is the trajectory? Will it continue to go at this pace? Will it slow down? Re-accelerate? How should I respond? Do we need to jump on it? Do we need to wait and see? Let everybody else do the first experience, experience the pains, and then we will adopt whatever works? What are the threats and what are the risks? These are common questions, but given the pace of things, they are crucial.
To answer these questions, one could look to the people who develop all this new technology. But the question is whether we can trust them. Sam Altman is looking for $7 trillion. I think the GDP of Germany is what? $4 trillion or $5 trillion. Last week Eric Schmidt, ex-Google CEO, stated on TV that AI is underhyped. He said the arrival of a non-human intelligence is a very, very big deal. Then the interviewer asked: is it here? And his answer was: it’s here, it’s coming, it’s almost here. Okay, so what is it? Is it here or is it coming? Anyway, he thinks it’s underhyped.
We need to look at the data, but even that isn’t trivial. Because if you look at generative AI, Large Language Models and how to measure their performance, it’s not easy. Because how do you determine if a response is actually accurate or not? You can’t measure it easily. In any case, we see the field progressing, and we’ve all seen the news around models beating bar exams and so on.
The key thing here is that all this progress is based on the AI scaling hypothesis, which states that as long as we throw more data and compute at it, we’ll advance. We’ll get ahead. This is the secret hypothesis that people are basing their claims on. And there are incentives for the industry to make the world believe that we’re close to artificial general intelligence. So we can’t fully trust them in my opinion, and we have to keep looking at the data. But the data tells us we’re still advancing. So what does that mean? Because current systems are anything but perfect. You must have seen ample examples. One is from Air Canada. They deployed a chatbot for their customer service, and the chatbot gave away free flights. It was a bug in the system.
That brings us to the skeptical view. What are the arguments? One is about large language modelling or generative AI in general: the flaws that we’re seeing are not bugs to be fixed. The way this technology works, by definition, has these flaws. These flaws are features, they’re not bugs. And part of that is that the models do not represent how the world works. They don’t have an understanding of the world. They just produce text in the case of a Large Language Model.
On top of that, they claim that there are diminishing returns. If you analyze the performance, for instance, of the OpenAI stuff that’s coming out, they claim that if you look at the benchmarks, it’s not really progressing that much anymore. And OpenAI hasn’t launched GPT-5, so they’re probably struggling. And all the claims are based on these scaling laws, and those scaling laws can’t go on forever. We’ve used all the data in the world, all the internet by now. So we’re probably hitting a plateau. This is the skeptical view. So on the one hand we hear all the progress and all the promises, but there are also people saying, “Look, that’s actually not the case if you really look under the hood of these systems.”
As for questions asked by organization leaders: “What do I need to do?” “How fast is this going?” Here, the predictions vary. In the Dutch Financial Times, here’s an economist saying it’s overhyped, it’s the same as always, all past technology revolutions took time and it will be the same this time. On the other hand, a recent report that came out saying this time is different: generative AI is a different type of technology and this is going to go much faster. The implication being that if you don’t stay ahead, if you don’t participate as an organization, you will be left behind soon.
The argument for generative AI is that the infrastructure is already there. It’s not like electricity, where we had to build power lines. For generative AI, the infrastructure is there. The cloud is rolled out. Software has become modular. And the technology itself is very intuitive. It’s very easy for people to interact with it because it’s based on natural language. All of those arguments are the basis for saying that this is going to go much faster. And I think some of us recognize that.
Looking ahead: how leaders should prepare
There’s a difference between adopting new tools and really changing your organization. When we think about the implications, at Rewire we try to make sense of these polarized views and form our own view of what is really happening and what it means for our clients, for our partners, and the people we work with. We have three key takeaways.
The first one is that we firmly believe that everybody needs to develop their own intuition and understanding of AI. Especially because we’re living in the smoke and mirror phase. It means that it’s important for people who have the role of shaping their organization to understand the technology and develop their own compass of what it can do, to navigate change.
The second is that you need to rethink the fundamentals. You need to think about redesigning things, re-engineering things, re-imagining your organization, asking what if, rather than adopting a tool or a point solution. You must think how your organization is going to evolve, what will it look like in five years’ time and how do we get there?
The third, is that yes, I agree with the fact of this Andrew McAfee, the economist that says generative AI is different because it goes faster. To a certain extent that’s true. But not to the point where full business models and full organizations and end-to-end processes change. Because that’s still hard work, it’s transformational work that doesn’t happen overnight. So the answers are nuanced. It’s not one extreme or the other. It is a long-term game to reap the benefits of this new technology.
What Generative AI can and cannot do. And what it means for the future of business.
Meet Edmond de Belamy, the portrait painting displayed above. On Thursday October 25th in 2018, Edmond was auctioned off by Christie’s for a whopping $432,500. The signature on the bottom right of Edmond shows its artist to be

This impressive-looking formula represents a GAN network: a generative AI-model avant-la-lettre. Edmond de Belamy became the first AI-generated artwork to be auctioned off by a major auction house.
The Belamy portrait was the first time we were truly impressed by the capabilities of a GenAI model. Nowadays, its quality is nothing special. In fact, we have rapidly gotten used to image generation models producing photorealistic images, text generation models that generate e-mail texts and meeting summaries better than we could ourselves, and even LLMs that support us developers and data scientists in writing code.
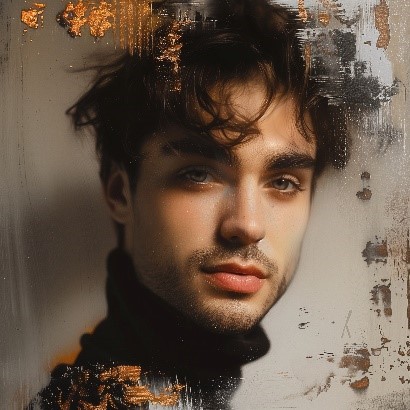
The breakthrough of GenAI, heralded by the release of ChatGPT in December 2022, has truly been amazing. It may almost lead you to believe that the age of humans is over, and the age of machines has now truly begun.
That is, until you start asking these GenAI models the hard questions. Such as:
Can you give me an odd number that does not have the letter “E” in it?
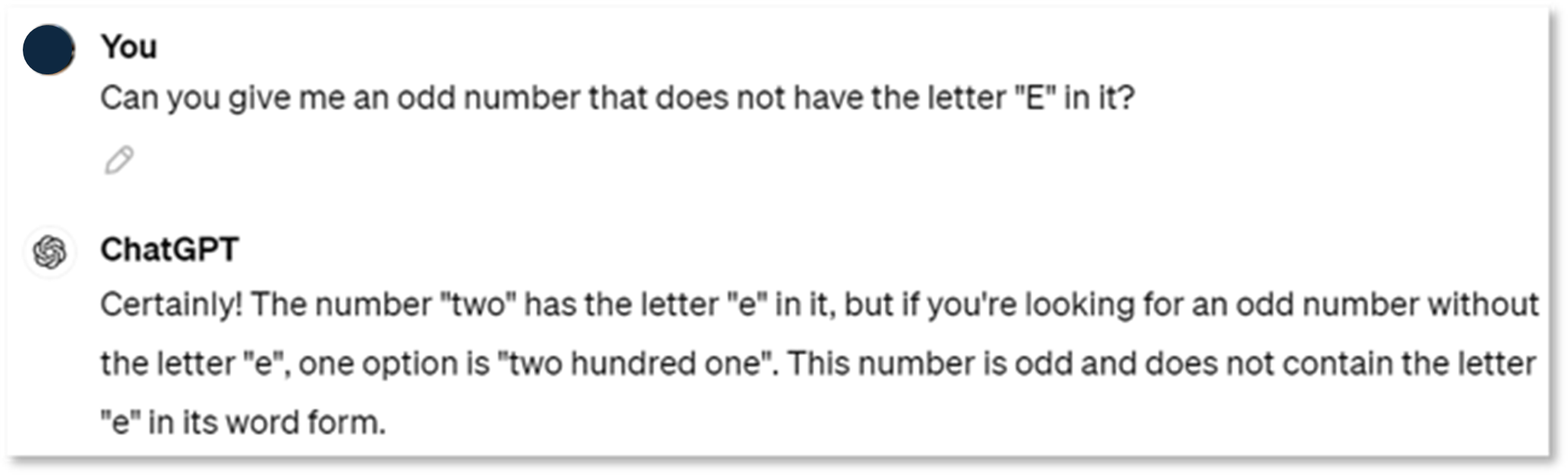
Anyone who can read could tell you GPT-4 botched that question. The correct answer would have been: no, I cannot, because such a number does not exist. Despite its ‘reasoning’, ChatGPT manages to confidently announce the number “two” has an “e” in it (note: it doesn’t), and continues to produce “two hundred one” as an example of an odd number that does not have an “e” in it (note: it does).
Another favorite pastime of ours is to ask LLM models to play chess with us. Our typical experience: they will claim they know the rules, and proceed to happily play illegal moves halfway through the game, or conjure up ghost pieces that manage to capture your knight out of nowhere.
The internet is full of entertaining examples of ChatGPT getting tasks wrong that humans solve without a second thought. These issues are not ChatGPT-specific either. Other LLM-providers, such as Anthropic, Google, Meta, and so on, face similar challenges.
So, on the one hand, GenAI models have proven to be an impressive breakthrough. On the other hand, it is clear that they are far from infallible. This begs the question:
What is the breakthrough of GenAI really?
And more importantly:
What does this mean for someone wanting to make the most of this technology?
Dissecting the GenAI breakthrough
In the 21st century we have seen the breakthrough of machine learning ending the ‘AI winter’ of the 1990s. Machine learning is essentially computers generalizing patterns in data. Three things are needed to do that well: algorithms for identifying patterns, large amounts of data to identify the patterns in, and computation power to run the algorithms.
By the start of the 2000s, we did know a fair bit about algorithms. The first Artificial Neural Networks, which form the foundation of ‘deep learning’, were published in 1943 (McCulloch et al., 1943). Random forest algorithms, the precursors to modern tree ensemble algorithms, were initially designed in 1995 (Breiman et al., 2001).
The rise of the digital age added the other two ingredients. We saw exponential growth in the amount of stored data worldwide. Simultaneously computing power kept getting exponentially cheaper and more easily accessible. All of a sudden, ‘machine learning’ and therefore AI, started to fly. Apparently, tossing large amounts of data, and huge amounts of computing power at a suitable algorithm can achieve a lot.
With machine learning we managed to have a go at quite a few problems that were previously considered difficult: image recognition, speech-to-text, playing chess. Some problems remained remarkably difficult, however. One of which was automated understanding and generation of human language.
This is where LLMs come in. In 2018, the same year our good fellow Edmond was created, OpenAI published a remarkable paper. They introduced a class of algorithms for language understanding called Generative Pre-trained Transformers, for short: “GPT” (Radford et al., 2018). This combined two key ideas. One that allowed them to “pre-train” language models on low-quality text as well as beautifully crafted pieces. And another that allowed these algorithms to benefit efficiently from large amounts of computing power. Essentially, they asked these models to learn how to predict the next word in a sentence, ideally enabling them to generate their own sentence if they became good enough at that.
GPT-1 was born with this paper in 2018, but OpenAI did not stop there. With their new algorithms they were able to scale GPT-1 both in model size, but just as importantly in terms of how much data it could be fed. GPT-3, the model that powered ChatGPT at its launch in 2022, was reportedly trained on almost 500 billion text tokens (which is impressive, but not even close to the ‘entire internet’, as some enthusiasts claim), and with millions of dollars of computing power.
Despite its insane jump in performance, the fundamental task of GPT-3 remains the same as it was for GPT-1. It is a very large, very complex machine learning language model trained to predict the next word in a sentence (or predict the next ‘token’, to be more precise). What is truly remarkable, however, is the competencies that emerge from this skill, which impressed even the most sceptical.
Emergent competencies of Large Language Models
Predicting the next word is cool, but why are these LLMs heralded by some as the future of everything (and by some as the start of the end of humanity)?
Despite being trained as “next-word-predictors” LLMs have begun to show competencies that truly do make them game changers. To be able to predict the next word based on a prompt, they have to do three things:
- Understand: making sense of unstructured data from humans and systems;
- Synthesize: processing information to ‘connect the dots’ and formulate a single, sensible response;
- Respond: generating responses in human or machine-readable language that provide information or initiate next steps.

Let’s unpack all this by looking at an example.
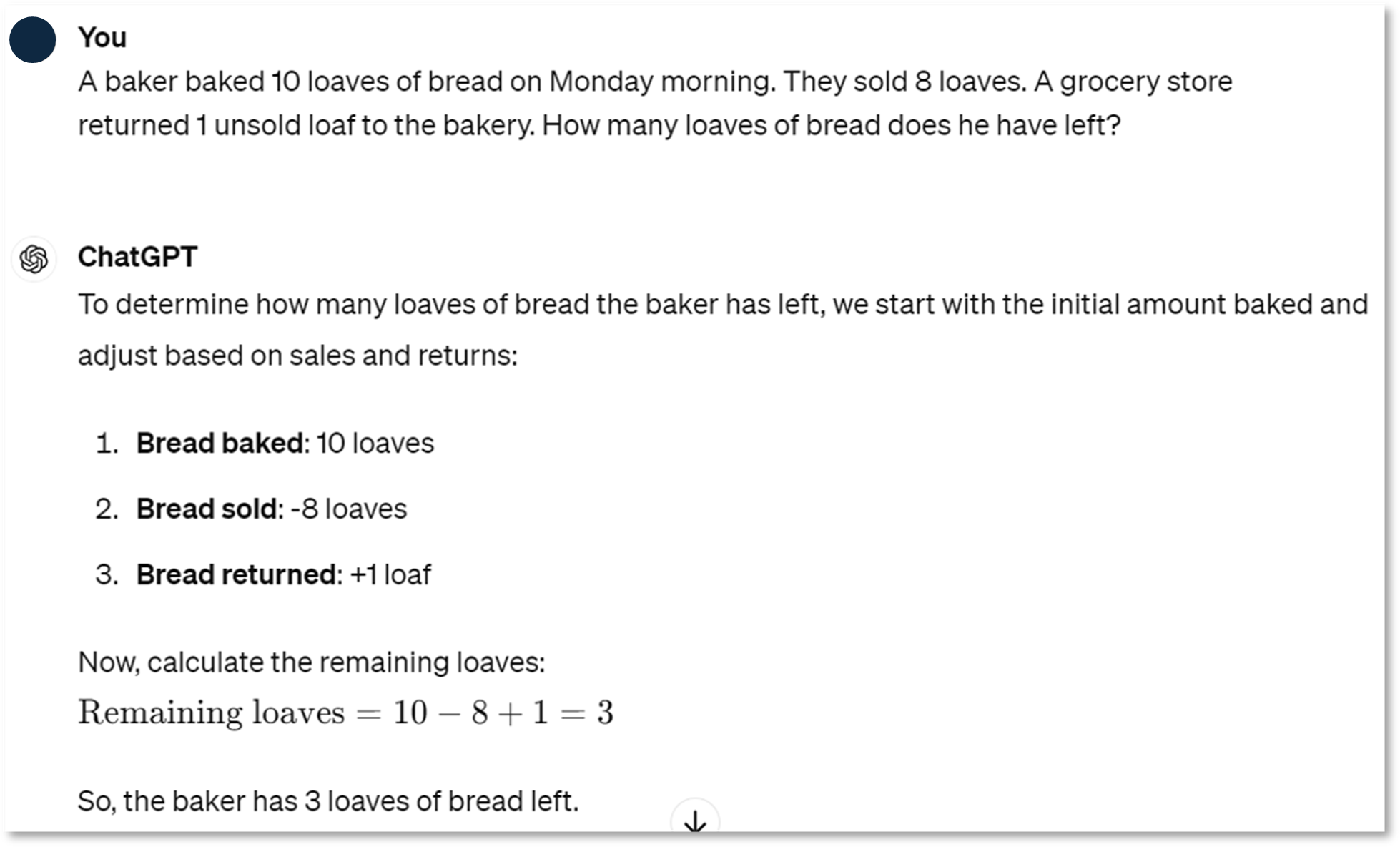
In this example, we asked ChatGPT powered by GPT-4 to solve a problem that may seem easy to anyone with basic arithmetic knowledge. Let’s pause to appreciate why ChatGPT’s ability to solve the problem is remarkable regardless.
Firstly, ChatGPT understands what we are asking. It manages to grasp that this question requires logical reasoning and gives us an answer that includes argumentation and a calculation. Furthermore, it can synthesize a correct response to our request, generating essentially ‘new’ information that was not present in the original prompt: the fact that we will have 3 loaves of bread left at the end of the day. Finally, it can respond with an answer that makes sense. In this case, it’s a human language response, wrapped in some nice formatting, but ChatGPT can also be used to generate ‘computer language’ in the form of programming code.
To put this into perspective: previous systems that were able to complete such prompts, would have generally been composed of many different components working together, tailored to this particular kind of problem. We’d have a text understanding algorithm, that extracts the calculation to be done from the input prompt. Then a simple calculator to perform the calculation, and a ‘connecting’ algorithm that feeds the output from the first algorithm into the calculator. Finally, we’d have a text generating system that takes the output and inputs the text into a pre-defined text template. With ChatGPT – we can have one model perform this whole sequence, all by itself. Lovely.
To put it in even more perspective: these types of problems were still difficult for older versions of ChatGPT. It shows that these emerging competencies are still improving in newer versions of state-of-the-art models.
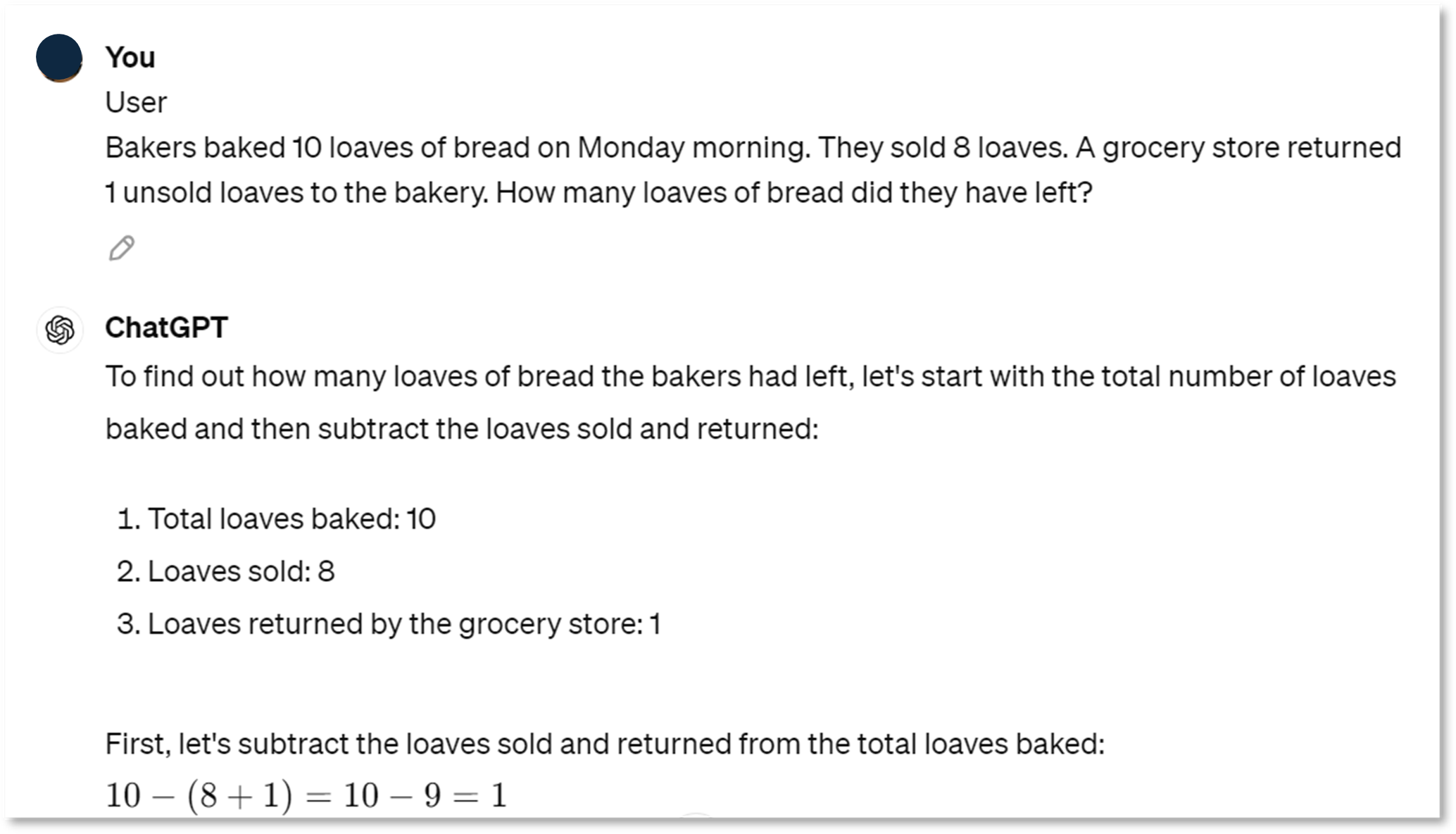
So far, we haven’t even considered models that can do understanding, synthesis and generation across multiple types of input and outputs (text, images, sound, video, etc.) These multi-modal models are all the rage since early 2024. The world of GenAI is truly moving quickly.
So what does it mean for businesses?
The talents of GenAI are truly impressive, making them a very versatile tool that opens up endless possibilities. It can craft beautiful e-mails, help generate original logos, or speed up software development.
Even more promising is GenAI’s ability to make sense of unstructured data at scale. Estimates put the amount of unstructured data to be about 80-90% of all the data that companies possess. Extracting value from this data through traditional means is time-consuming and challenging at best. This has led to unstructured data being largely ignored for many commercial use cases. Yet GenAI can plough through it and generate outputs tailored to the desired use cases. You can uncover the needles in haystacks to fuel human decisions, or enable more traditional AI systems to learn from this data. Imagine how powerful these systems would become if you increased the amount of useful information available to them by a factor 5 to 10.
Now, if you’ve been following some of the AI news lately, you’ll know we can take it even one step further and think about ‘Agentic AI’, that is, agents powered by AI. These systems that cannot only think, but actually do. In future, they will likely enable large scale automation at first, and later complete organizational transformations.
Research into how to make this work is in full swing as of the summer of 2024. The extent to which autonomous AI agents are already feasible is a hotly debated topic. At any rate, ‘simple’ agents, are now being developed that are beginning to capitalize on the AI promise. Making the most of these will require their users to carefully consider how to manage their performance, and balance two opposing characteristics: hallucinations (i.e. nonsensical results, which some argue are an inescapable feature of LLMs), and their effectiveness.
Against this backdrop, it won’t be long before the early adopters get a head start on the laggers. Teams and organizations that take the time to identify opportunities and capitalize on them are set to move far ahead of the competition.
This article was written by Simon Koolstra, Principal and Renske Zijm, Data scientist at Rewire.