A handful of principles will set you up for success when bringing GenAI to enterprise data.
As highlighted in our 2025 Data Management Trends report, the rapid evolution of LLMs is accelerating the shift of GenAI models and agentic systems from experimental pilots to mission-critical applications. Building a proof of concept that taps a single data source or uses a few prompts is relatively simple. But delivering real business value requires these models to access consistent, high-quality data—something often trapped in silos, buried in legacy systems, or updated at irregular intervals.
To scale from pilot to production, organizations need a more structured approach—one grounded in solid data management practices. That means integrating with enterprise data, enforcing strong governance, and ensuring continuous maintenance. Without this foundation, organizations risk stalling after just one or two operationalized GenAI use cases, unable to scale or accommodate new GenAI initiatives, due to persistent data bottlenecks. The good news is that those early GenAI implementation efforts are the ideal moment to confront your data challenges head-on and embed sustainable practices from the start.
How, then, can data management principles help you build GenAI solutions that scale with confidence?
In the sections that follow, we’ll look at the nature of GenAI data and the likely challenges it poses in your first value cases. We’ll then explore how the right data management practices can help you address these challenges—ensuring your GenAI efforts are both scalable and future-proof.
Understanding the nature of GenAI data
GenAI data introduces a new layer of complexity that sets it apart from traditional data pipelines, where raw data typically flows into analytics or reporting systems. Rather than dealing solely with structured, tabular records in a centralized data warehouse, GenAI applications must handle large volumes of both structured and unstructured content—think documents, transcripts, and other text-based assets—often spread across disparate systems.
To enable accurate retrieval and provide context to the model, unstructured data first be made searchable. This typically involves chunking the content and converting it into vector embeddings or exposing it via a Model Context Protocol (MCP) server, depending on the storage provider (more on that later). Additional context is increasingly delivered through metadata, knowledge graphs, and prompt engineering, all of which are becoming essential assets in GenAI architectures. Moreover, GenAI output often extends beyond plain text. Models may generate structured results—such as function calls, tool triggers, or formatted prompts—that require their own governance and tracking. Even subtle variations in how text is chunked or labeled can significantly influence model performance.
Because GenAI taps into a whole new class of data assets, organizations must treat it differently from standard BI or operational datasets. This introduces new data management requirements to ensure that models can reliably retrieve, generate, and act on timely information—without drifting out of sync with rapidly changing data. By addressing these nuances from the outset, organizations can avoid performance degradation, build trust, and ensure their GenAI solutions scale effectively while staying aligned with business goals.
Start smart: consider data representability
Selecting your first GenAI value case to operationalize is a critical step—one that sets the tone for everything that follows. While quick experiments are useful for validating a model’s capabilities, the real challenge lies in integrating that model into your enterprise architecture in a way that delivers sustained, scalable value.
Choosing the right value case requires more than just identifying an attractive opportunity. It involves a clear-eyed assessment of expected impact, feasibility, and data readiness. One often overlooked but highly valuable criterion is data representability: how well does the use case surface the kinds of data challenges and complexities you’re likely to encounter in future GenAI initiatives?
Your first operationalized value case is, in effect, a strategic lens into your data landscape. It should help you identify bottlenecks, highlight areas for improvement, and stress-test the foundations needed to support long-term success. Avoid the temptation to tackle an overly ambitious or complex use case out of the gate—that can easily derail early momentum. Instead, aim for a focused, representative scenario that delivers clear early wins while also informing the path to sustainable, scalable GenAI adoption.
Don’t get stuck: Answer these critical data questions
The targeted approach described above delivers the early tangible win needed to get stakeholder buy-in—and, more critically, it exposes underlying data issues that might otherwise go unnoticed. Here are typical data requirements for your first GenAI use case, along with the key challenge they create and core questions you’ll need to answer.
Requirement | Challenge | Key questions |
Integrated information | Previously untapped information (for example, pdf documents), is now a key data source and must be integrated with your systems. | * Where is all this data physically stored? Who maintains ownership of each source? * Do you have the necessary permissions to access them? * Does the data storage tool provide a MCP server to interact with the data? |
Searchable knowledge system | Searchable documents must be chunked, embedded, and updated in a vector database—or exposed via an MCP server—creating new data pipeline and infrastructure demands. | * Can you find a reliable embedding model for capturing the nuances in your business? * How frequently must you re-embed to handle updates? * Are the associated costs manageable at scale? |
Context on how data is interconnected | Reasoning capabilities require explicitly defined relationships—typically managed through a knowledge graph. | * Is your data described well enough to construct such a graph? * Who will ensure these relationships and definitions stay accurate over time? |
Retrieval from relational databases by an LLM | Models need rich, well-documented metadata to understand and accurately query relational data. | * Are vital metadata fields consistent and detailed? * Do you have the schemas and documentation required for the model to navigate these databases effectively? |
Defining prompts, output structures and tool interface | Prompts, interfaces, and outputs must be reusable, versioned, and traceable to scale reliably and maintain trust. | * How do you ensure these prompts and interfaces are reusable? * Can you track changes for auditability? * How do you log their usage to troubleshoot issues? |
Evaluate model outputs over time | User feedback loops are essential to monitor performance, but collecting, storing, and acting on feedback systematically is lacking. | * How do you store feedback? * How do you detect dips in accuracy or relevance? * Can you trace poor results back to specific data gaps? |
Access to multiple data sources for the LLM | GenAI creates data leakage risks (e.g. prompt injection), requiring access controls to secure model interactions. | * Which guardrails or role-based controls prevent unauthorized data exposure? * How do you ensure malicious prompts don’t compromise the agent? |
Transform potential into performance with GenAI
Whether you win or lose in your market may soon rely on having the best GenAI capability and the data foundations to support it. We can help you build this.
Discuss your needsLook beyond your first pilot: apply data product thinking -from day one
A common mistake is solving data challenges only within the narrow context of the initial pilot—resulting in quick fixes that don’t scale. As you tackle more GenAI projects, inconsistencies in data structures, metadata, or security can quickly undermine progress. Instead, adopt a reusable data strategy from day one—one that aligns with your broader data management framework.
Treat core assets like vector databases, knowledge graphs, relational metadata, and prompt libraries as data products. That means assigning ownership, defining quality standards, providing documentation, and identifying clear consumers—so each GenAI use case builds on a solid, scalable foundation.
Core data product ingredients for GenAI
Below, we outline the key principles for making that happen, along with examples, roles, and how to handle incremental growth.
1. Discoverability
Implement a comprehensive data catalogue that documents what data exists, how it's structured, who owns it, and when it's updated. This catalogue should work in conjunction with—but not necessarily be derived from—any knowledge graphs built for specific GenAI use cases. While knowledge graphs excel at modelling domain-specific relationships, your data catalogue needs broader enterprise-wide coverage. Include the metadata and examples needed for generating SQL queries, as well as references for your vector database. (This is something we find particularly useful, for example when developing AI agents that autonomously generate SQL queries to search through databases. Curated, detailed metadata, including table schemas, column definitions, and relationships allow the agents to understand the structure and semantics of the databases.) Define a scheduled process to keep these inventories current, to ensure that new GenAI projects can easily discover existing datasets or embeddings, while avoiding unnecessary re-parsing or re-embedding of data that is already available.
2. Quality standards
This includes a range of processes. From reviewing PDF documents to remove or clarify checkboxes and handwritten text to checking chunk-level completeness for embeddings, consistent naming conventions, or standardized templates for prompt outputs. Involve domain experts to clarify which fields or checks are critical, and align those standards in your data catalogue. By enforcing requirements at the source, you prevent silent inconsistencies (like missing metadata or mismatched chunk sizes) from undermining your GenAI solutions later on.
3. Accessibility
GenAI models require consistent, reliable ways to retrieve information across your enterprise landscape—yet building a custom integration for every data source quickly becomes unsustainable. As an example, at a client a separate data repository was created—with its own API and security—to pull data from siloed systems. This is where the MCP comes in handy: by specifying a standard interface for models to access external data and tools, it removes much of the overhead associated with custom connectors. Not all vendors support MCP but adoption is growing (OpenAI for example provides it). So keep that in mind and avoid rushing into building custom connectors for every system. In sum, focus on building a solid RAG foundation for your most critical, large-scale enterprise data—where the return on investment is clear. For simpler or lower-priority use cases, it may be better to wait for MCP-ready solutions with built-in search, rather than rushing into one-off integrations. This balanced approach lets you meet immediate needs while staying flexible as MCP adoption expands. (We’ll dive deeper into MCP in our next blog post.)
4. Auditability
Log every step—when doing at least make sure to include knowledge graph updates to prompts, reasoning, and final outputs—so you can pinpoint which data, transformations, or model versions led to a given response. This is especially vital in GenAI, where subtle drifts (like outdated embeddings or older prompt templates) often go unnoticed until user trust is already compromised. From a compliance perspective, this is critical when GenAI outputs influence business decisions. Organizations may need to demonstrate exactly which responses were generated, what processing logic was applied, and which data was used at specific points in time—particularly in regulated industries or during audits. Ensure each logged event links back to a clear version ID for both models and data, and store these audit trails securely with appropriate retention policies to meet regulatory, privacy, and governance requirements.
5. Security & access control
Ensure appropriate role- and attribute-based access is enforced on all data sources accessed by your model, rather than granting blanket privileges. Align access with the end-user's identity and context, preventing the model from inadvertently returning unauthorized information. Be particularly vigilant about prompt injection attacks—where carefully crafted inputs manipulate models into bypassing security controls or revealing sensitive information. This remains one of GenAI's most challenging security vulnerabilities, as traditional safeguards can be circumvented through indirect methods. Consider implementing real-time checks, especially in autonomous agent scenarios, alongside an "LLM-as-a-judge" layer that intercepts and sanitizes prompts that exceed a user's permissions or exhibit malicious patterns. While no solution offers perfect protection against these threats, understanding the risk vectors and implementing defence-in-depth strategies significantly reduces your exposure. Resources like academic papers and technical analyses on prompt injection techniques can help your team stay informed about this evolving threat landscape.
6. LLMOps
A shared, organization-wide LLMOps pipeline and toolkit gives every GenAI project a stable, reusable foundation—covering everything from LLM vendor switching and re-embedding to common connectors and tool interfaces. Centralizing these resources eliminates ad-hoc solutions, promotes collaboration, and ensures proper lifecycle management. The result: teams can launch GenAI initiatives faster, apply consistent best practices, and still stay flexible enough to experiment with different models and toolchains.
The takeaway: combine your GenAI implementation with proper data management
Implementing GenAI models and agentic systems isn’t just about prompting an LLM. It’s a data challenge and an architectural exercise. By starting with a high-impact use case, you secure immediate value and insights, while robust data management practices ensure you stay in control—maintaining quality and trust as AI spreads across your organization. By adopting these principles in your very first GenAI initiative, you avoid the trap of quick-win pilots that ultimately buckle under growing data complexity. Instead, each new use case taps into a cohesive, well-governed ecosystem—keeping your AI agents current, consistent, and secure as you scale.
Those who excel at both GenAI innovation and data management will be the ones to transform scattered, siloed information into a unified, GenAI-ready asset—yielding genuine enterprise value on a foundation built to last. If you haven’t already established data management standards and principles, don’t wait any longer—take a look at our blog posts on data management fundamentals part 1 and 2.
If you’d like to learn more about building a robust GenAI foundation, feel free to reach out to us.
This article was written by Daan Manneke, Data & AI Engineer at Rewire and Frejanne Ruoff, Principal at Rewire.
The race to building competitive advantage just took an interesting turn. Here is what you need to know.
What is model distillation?
In chemistry, distillation is a purification process where a liquid mixture is heated until components with different boiling points separate. The result is often a more concentrated, refined substance. This elegant concentration technique has been used for centuries to create everything from perfumes to whiskey, preserving essential qualities while removing unnecessary bulk.
Model distillation in AI follows a remarkably similar principle; knowledge is extracted from a large and complex AI model (the “teacher”) and transferred to a smaller, more efficient model (the “student”). At its core, model distillation is about teaching the student to act like the teacher. Instead of training a small model with traditionally labeled data, response-based knowledge distillation allows the student model to mimic the intelligence of the teacher (i.e., output token probability distributions, for the data scientists among us).
The result is a refined, lightweight model that captures a concentrated essence of its larger counterpart's capabilities within its domain of expertise.
Why model distillation is everywhere
While DeepSeek did not invent model distillation, their breakthrough in early 2025, which we discussed before, catapulted cost efficiency for GenAI models into the frontstage, proving that smaller teams with limited resources could compete at the cutting edge of AI development.
The latest generation Large Language Models (LLMs) like GPT-4.5, DeepSeek V3, and Claude 3.7 are incredibly powerful—but also incredibly expensive to run. Model distillation offers the process of training a smaller model to mimic a larger one, delivering near state-of-the-art performance in a narrow domain at a fraction of the cost.
DeepSeek was a game changer in the pricing of large scale models. Model distillation extends beyond that: it enables AI capabilities where even the cheapest full-scale LLMs are too expensive or impractical to run.
For example, researchers from the University of Washington created their own reasoning model in merely 26 minutes for less than $50. InHand Networks successfully distilled models on their edge AI computers to provide low-power real-time inference capabilities. This revolution has forced even industry giants like OpenAI to reconsider their closed-source approach.
Why it is a game-changer for businesses
The value of model distillation extends far beyond reducing expenses—it unlocks new possibilities for AI implementation that were not feasible previously. Here's how it's changing the game:
✅Better unit economics. Running small, distilled models requires significantly less computational resources, translating directly to lower operational costs for high-volume AI applications.
✅ Enhanced inference speed. Smaller models process data more quickly, leading to faster response times and improved user experience in time-sensitive applications.
✅ Near state-of-the-art performance. For well-defined use cases, a distilled model can perform remarkably close to full-size LLMs while being more efficient and cost-effective.
✅ Smaller bottleneck on data. The requirement on your data shifts from curating and labeling large datasets, to a smaller curated set of examples with teacher-generated answers, significantly reducing annotation costs and time.
Not a silver bullet: the downsides you should consider
Model distillation is not without hurdles. Here’s what you need to plan for:
❌ Upfront development cost. Building and training your own distilled model takes time and effort from experts in your team.
❌ Teacher performance limitation. The small model can only be as good as the large model it learns from. Furthermore, some large proprietary model providers like OpenAI and Anthropic put restrictions on the output of their models, hindering their use for model distillation.
❌ No automatic updates. When improved foundation models are released, you need to repeat the process of distillation to get access to these improvements in distilled format.
Model distillation in action: where it works best
Distillation works great when you need cost-effective, scalable AI in a controlled environment. Good use cases include:
🔹 Edge devices & on-device solutions. Frontier models do not fit on consumer hardware or smaller edge devices. (Edge devices—e.g., phones, sensors, or cameras—process data locally and on the spot instead of sending it to a distant data center.) If low-latency or portability is a requirement, you may want to embed the required intelligence in a smaller package.
🔹 Personalized content generation at scale. Think online learning platforms that generate exercises tailored to their students’ needs, or marketing tools that generate personalized e-mail content.
🔹 Domain-specific chatbots. Customer support bots trained for highly specific industries without relying on expensive full-scale models for all interactions.
🔹Specialized parts of agentic workflows. Agents use notoriously many tokens. Hence, splitting your solution up in specialized modules with distilled models can reduce costs and latency significantly, especially at scale.
However, don’t use model distillation for deep research or complex, open-ended reasoning tasks. If your AI needs to push the boundaries of intelligence or perform highly creative problem-solving, a distilled model won’t cut it; inherently, it will remain inferior to its teacher.
The bottom line: does distillation fit your business needs?
Model distillation is transforming from an academic idea into a competitive advantage for businesses. It democratizes advanced AI capabilities while dramatically reducing costs. For businesses facing the dual pressures of innovation and efficiency, distillation offers a middle ground: near state-of-the-art performance without the steep price tag.
Just be mindful that it’s not a silver bullet. Many use cases will still require full-scale LLMs, which offer the benefit of broader and more general knowledge and capabilities.
If your AI roadmap has well-defined use cases in a specific domain that can afford a short-term investment for long-term cost benefits, it may well be worth it to explore this approach.
This article was written by Jacco Broere, Data & AI Engineer at Rewire and Simon Koolstra, Principal at Rewire.
Unlocking true intelligence with memory, strategic planning, and transparent performance management
The rise of intelligent AI agents marks a shift from traditional task automation toward more adaptive, decision-making systems. While many AI-powered workflows today rely on predefined rules and deterministic processes, true agentic AI goes beyond fixed automation—it incorporates memory, reasoning, and self-improvement to adapt and autonomously achieve specific goals.
The previous agentic AI blog post explained the building blocks (memory, planning, tools and actions), use cases, and potential for autonomy. It positioned AI agents not just as tools for automating repetitive tasks but as systems capable of enhancing workflow efficiency and tackling complex problems with some degree of independent decision-making. In this blog post, we explore how these agents can be seamlessly integrated into real-world scenarios, striking a balance between cognitive science theory and the practical realities of human-agent interaction, with a particular focus on the memory and planning building blocks, alongside the addition of performance management.
Moving from models to real-world AI agents
Scaling AI agents presents challenges. While they can improve efficiency, their success depends on integration with existing systems. Use cases like personal assistants and content generators demonstrate clear value, whereas others struggle with reliability and adaptability.
Current LLM-based workflow automation relies on knowledge—whether through large reasoning models or knowledge bases. However, these agents often lack persistent memory, meaning they re-solve the same issues repeatedly. Without storing and leveraging past experiences, they remain reactive rather than truly intelligent. To bridge this gap, AI agents need:
- Memory – Retaining and applying past experiences.
- Ability to plan – Setting goals, adapting strategies, and managing complexity.
- Transparent performance management – Ensuring alignment, oversight, and trust.
These elements go beyond the building blocks of tools and actions, which have already been widely discussed in AI agent design. Here we focus on memory, planning, and performance management, as they represent critical design choices to move toward AI agents that are not just reactive task automators, but intelligent, adaptable decision-makers capable of handling more sophisticated tasks in real-world scenarios. Let’s start by exploring what intelligence in that sense even means.
Memory: the key to true intelligence
True intelligence goes beyond automation. An AI agent must not only process information but also learn from past experiences to improve over time. Without memory, an AI agent would remain static, unable to adapt or evolve. By integrating memory, reasoning, and learning, an intelligent AI agent moves beyond simply performing predefined tasks. In our previous blog post on AI agents, we explained that memory, planning, tools and actions are the building blocks of agents. Now, let's examine how memory plays a crucial role in enhancing an AI agent's capabilities. In cognitive science, memory is divided into:
- Semantic memory – A structured database of facts, rules, and language that provides foundational knowledge.
- Episodic memory – Past experiences that inform future decisions and allow for adaptation.
The interplay between semantic and episodic memory enables self-improvement: experiences enrich knowledge, while knowledge structures experiences. When agents lack episodic memory, they struggle with contextual awareness and must rely solely on predefined rules or external prompting to function effectively. To obtain intelligent agents, episodic memory is therefore crucial. By organizing past interactions into meaningful units (through chunking), agents can recall relevant solutions, compare them to new situations, and refine their approach. This form of memory actively supports an agent’s ability to reflect on past actions and outcomes.
To illustrate this, let's consider an example from supply chain management, as depicted in the image below. An AI agent that tracks delivery data, inventory, and demand can improve logistics by learning from past experiences. If the agent identifies patterns, such as delays during certain weather conditions or peak seasons, it can proactively adjust shipping schedules and notify relevant stakeholders. Without memory, the agent would simply repeat tasks without optimizing them, leading to inefficiencies and missed opportunities for improvement.
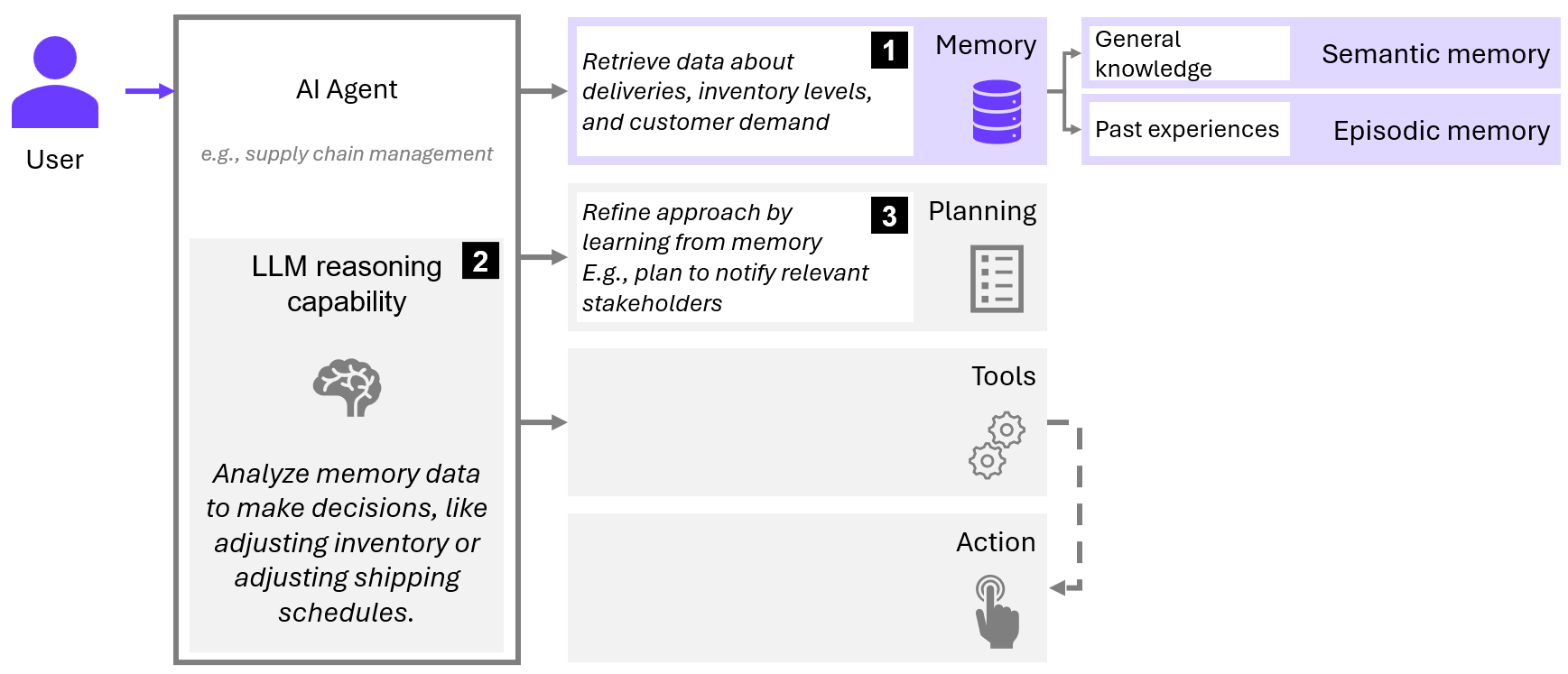
Figure 1 - Memory in AI Agents - An example from supply chain management
Ability to plan: the foundation for autonomous decision-making
Intelligent AI agents must dynamically plan and break complex problems into manageable tasks—mirroring the analytical nature of the human mind. Unlike rule-based automation, these agents should be able to assess different strategies, evaluate potential outcomes, and adjust their approach based on real-time feedback. Planning allows an agent to remain flexible, ensuring it can pivot when conditions change rather than blindly following predefined sequences.
LLMs serve as the reasoning engines of AI agents, showcasing increasingly advanced cognitive abilities. However, they struggle with long-term memory and sustained focus—much like the human mind under information overload. This limitation poses challenges in designing AI agents that must retain context across extended interactions or tackle complex problem-solving tasks.
A critical design question is whether an agent should retain plans internally or offload them to an external tool. Keeping plans within an LLM provides full information access but may be limited by context constraints. For example, an AI managing a real-time chat-based customer support system could benefit from internal memory to dynamically adapt to an ongoing conversation, keeping track of the customer's previous questions and preferences without relying on external systems. This allows the agent to provide personalized responses without the delay of querying an external database. On the other hand, external tools lighten the cognitive load but can introduce rigidity if not well-integrated. For instance, an AI-powered weather application might be better off using an external tool to retrieve up-to-date weather data rather than relying on its internal model, which could become outdated or too complex to manage. This allows the system to focus on processing and presenting the information without overloading its internal resources. A balanced approach ensures adaptability without overloading the agent’s working memory. Ultimately, the necessity of such a tool depends on the LLM's ability to retrieve, retain, and adjust information—an advanced reasoning model might even eliminate the need for external tools.
For example, an AI-powered financial advisor might need to balance long-term investment strategies with short-term market fluctuations. If it relies too heavily on immediate context, it might make impulsive decisions based on temporary trends. On the other hand, if it solely adheres to a rigid external planning framework, it might fail to adapt to new opportunities. The ideal approach blends both—leveraging structured knowledge while maintaining the ability to dynamically reassess and adjust strategies.
Transparent performance management: balancing efficiency and trust
Human-AI agent interaction is shaped by the trade-off between efficiency and trust: the more autonomous an AI agent becomes, the more it can streamline operations and reduce human workload—yet the less transparent its decision-making may feel. In scenarios where tasks are low-risk and repetitive, full automation makes sense as errors have minimal impact, and efficiency gains outweigh the downsides. However, in high-stakes environments like financial trading or medical diagnosis, the costs of a wrong decision are simply too high. Transparent performance management is thus essential.
The challenge is that AI agents, while improving, are still fallible, inheriting issues like hallucinations and biases from LLMs. AI must operate within defined trust thresholds—where automation is reliable enough to act independently yet remains accountable. Rather than requiring continuous human oversight, performance management should focus on designing mechanisms that allow AI agents to function autonomously while ensuring reliability. This involves self-monitoring, self-correction, and explainability.
Mechanisms like agent self-critique mitigate these issues by enabling agents to evaluate their own decisions before execution. Also known as LLM-as-a-judge, self-critique involves sending both input and output to a separate LLM entity that is unaware of the entire agentic workflow, assessing whether the response logically follows from the input. For instance, an LLM can check its output for consistency by sending both its input and response to a separate validation model, which then determines whether the response aligns with the provided information. This process helps catch hallucinations, biases, and inconsistencies before decisions are finalized, improving the reliability of autonomous AI agents.
In the early stages of AI agent deployment, human experts play a crucial role in shaping and refining performance management processes. However, as these agents evolve, the goal is to reduce direct human intervention while maintaining oversight through structured performance metrics. Instead of requiring constant check-ins, AI agents should be designed to self-monitor and adapt, ensuring alignment with objectives without excessive human involvement. By incorporating mechanisms for self-assessment, AI agents can achieve greater autonomy while maintaining accountability. The ultimate aim is to develop fully autonomous agents that balance efficiency with transparency—operating independently while ensuring performance remains reliable.
For example, consider an AI agent managing IT system maintenance in a large enterprise. Such an agent monitors server performance, security threats, and software updates. Instead of relying on human intervention for every decision, it can autonomously detect anomalies, apply minor patches, and optimize system configurations based on historical performance data. However, major decisions—such as deploying a company-wide software update—may still require validation through transparent reporting mechanisms. If the AI agent consistently demonstrates accuracy in its assessments and risk predictions, human involvement can gradually decrease, ensuring both operational efficiency and system integrity.
Transform potential into performance with GenAI
Whether you win or lose in your market may soon rely on having the best GenAI capability and the data foundations to support it. We can help you build this.
Discuss your needsWhat’s next for AI agents?
AI agents are evolving beyond simple automation. To become truly intelligent, they must adapt, plan, and learn from experience. Without memory, an agent is static. Without planning, it lacks direction. Without transparent performance management, it risks unreliability.
By integrating memory, planning, and performance management, AI agents can move beyond task execution toward strategic problem-solving. Future AI will not merely automate processes but will actively contribute to decision-making, helping organizations navigate complexity with greater precision and efficiency.
The future belongs to AI that doesn’t just execute tasks but remembers, adapts, and improves. An agent without intelligence is merely automation with an attitude.
Sources
Greenberg DL, Verfaellie M. "Interdependence of episodic and semantic memory: evidence from neuropsychology." J Int Neuropsychol Soc. 2010;16(5):748-753. doi:10.1017/S1355617710000676. Link.
"Does AI Remember? The Role of Memory in Agentic Workflows." (2025) Link.
"RULER: What's the Real Context Size of Your Long-Context Language Models?" (2024). arXiv:2404.06654
This article was written by Gijs Smeets, Data Scientist at Rewire and Mirte Pruppers, Data Scientist at Rewire.
An introduction to the world of LLM output quality evaluation: the challenges and how to overcome them in a structured manner
Perhaps you’ve been experimenting with GenAI for some time now, but how do you determine when the output quality of your Large Language Model (LLM) is sufficient for deployment? Of course, your solution needs to meet its objectives and deliver reliable results. But how can you evaluate this effectively?
In contrast to LLMs, assessing machine learning models is often a relatively straightforward process: metrics like Area Under the Curve for classification or Mean Absolute Percentage Error for regression give you valuable insights in the performance of your model. On the other hand, evaluating LLMs is another ball game, since GenAI generates unstructured, subjective outputs – in the form of texts, images, or videos - that often lack a definitive "correct" answer. This means that you’re not just assessing whether the model produces accurate outputs; you also need to consider, for example, relevance and writing style.
For many LLM-based solutions (except those with very specific tasks, like text translation), the LLM is just one piece of the puzzle. LLM-based systems are typically complex, since they often involve multi-step pipelines, such as retrieval-augmented generation (RAG) or agent-based decision systems, where each component has its own dependencies and performance considerations.
In addition, system performance (latency, cost, scalability) and responsible GenAI (bias, fairness, safety) add more layers of complexity. LLMs operate in ever-changing contexts, interacting with evolving data, APIs, and user queries. Maintaining consistent performance requires constant monitoring and adaptation.
With so many moving parts, figuring out where to start can feel overwhelming. In this article, we’ll purposefully over-simplify things by answering the question: “How can you evaluate the quality of your LLM output?”. First, we explain what areas you should consider in the evaluation of the output. Then, we’ll discuss the methods needed to evaluate output. Finally, to make it concrete, we bring everything together in an example.
What are the evaluation criteria of LLM output quality?
High-quality outputs build trust and improve user experience, while poor-quality responses can mislead users and foster misinformation. The start of building an evaluation (eval) is to start with the end-goal of the model. The next step is to define the quality criteria to be evaluated. Typically these are:
- Correctness: Are the claims generated by the model factually accurate?
- Relevance: Is the information relevant to the given prompt? Is all required information provided -by the end user, or in the training data- to adequately offer an answer to the given prompt?
- Robustness: Does the model consistently handle variations and challenges in input, such as typos, unfamiliar question formulations, or types of prompts that the model was not specifically instructed for?
- Instruction and restriction adherence: Does the model comply with predefined restrictions or is it easily manipulated to jailbreak the rules?
- Writing style: Does the tone, grammar, and phrasing align with the intended audience and use case?
How to test the quality of LLM outputs?
Now that we’ve identified what to test, let’s explore how to test. A structured approach involves defining clear requirements for each evaluation criterion listed in the previous section. There are two aspects to this: references to steer your LLM towards the desired output and the methods to test LLM output quality.
1. References for evaluation
In LLM-based solutions, the desired output is referred to as the golden standard, which contain reference answers for a set of input prompts. Moreover, you can provide task-specific guidelines such as model restrictions and evaluate how well the solution adheres to those guidelines.
While using a golden standard and task-specific guidelines can effectively guide your model towards the desired direction, it often requires a significant time investment and may not always be feasible. Alternatively, performance can also be assessed through open-ended evaluation. For example, you can use another LLM to assess relevance, execute generated code to verify its validity, or test the model on an intelligence benchmark.
2. Methods for assessing output quality
Selecting the right method depends on factors like scalability, interpretability, and the evaluation requirement being measured. In this section we explore several methods, and assess their strengths and limitations.
2.1. LLM-as-a-judge
An LLM isn’t just a text generator—it can also assess the outputs of another LLM. By assessing outputs against predefined criteria, LLMs provide an automated and scalable evaluation method.
Let’s demonstrate this with an example. For example, ask the famous question, "How many r's are in strawberry?" to ChatGPT's 4o mini model. It responds with, "The word 'strawberry' contains 1 'r'.", which is obviously incorrect. With the LLM-as-a-judge method, we would like the evaluating LLM (in this case, also 4o mini) to recognize and flag this mistake. In this example, there is a golden reference answer “There are three 'r’s' in 'strawberry'.”, which can be used to evaluate the correctness of the answer.
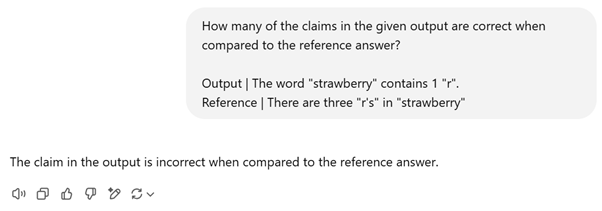
Indeed, the evaluating LLM appropriately recognizes that the answer is incorrect.
The example shows that LLMs can evaluate outputs consistently and at scale due to their ability to quickly assess several criteria. On the other hand, LLMs may struggle to understand complex, context-dependent nuances or subjective cases. Moreover, LLMs may strengthen biases within the training data and can be costly to use as an evaluation tool.
2.2. Similarity metrics for texts
When a golden reference answer is available, similarity metrics provide scalable and objective assessments of LLM performance. Famous examples are NLP metrics like BLEU and ROUGE, or more advanced embedding-based metrics like cosine similarity and BERTScore. These methods provide quantitative insights in measuring the overlap in words and sentence structure without the computational burden of running full-scale LLMs. This can be beneficial when outcomes must closely align with provided references – for example in the case of summarization or translation.
While automated metrics provide fast, repeatable, and scalable evaluations, they can fall short on interpretability and often fail to capture deeper semantic meaning and factual accuracy. As a result, they are best used in combination with human evaluation or other evaluation methods.
2.3. Human evaluation
Human evaluation provides a strong evaluation method due to its flexibility. In early stages of model development, it is used to thoroughly evaluate errors such as hallucinations, reasoning flaws, and grammar mistakes to provide insights into model limitations. As the model improves through iterative development, groups of evaluators can systematically score outputs on correctness, coherence, fluency, and relevance. To reduce workload and enable real-time human evaluation after deployment, pairwise comparison can be used. Here, two outputs are compared to determine which performs better for the same prompt. This is in fact implemented in ChatGPT.
It is recommended to use both experts as non-experts in human evaluation of your LLM. Experts can validate the model’s approach based on their expertise. On the other hand, non-experts play a crucial role in identifying unexpected behaviors and offering fresh perspectives on real-world system usage.
While human evaluation offers deep, context-aware insights and flexibility, it is resource- and time-intensive. Moreover, comparing different examiners can lead to inconsistent evaluations when they are not aligned.
2.4. Benchmarks
Lastly, there are standardized benchmarks that offer an approach to assess the general intelligence of LLMs. These benchmarks evaluate models on various capabilities, such as general knowledge (SQuAD), natural language understanding (SuperGLUE), and factual consistency (TruthfulQA). To maximize their relevance, it’s important to select benchmarks that closely align with your domain or use case. Since these benchmarks test broad abilities, they are often used to identify an initial model for prototyping. However, standardized benchmarks can provide a skewed perspective due to their lack of alignment with your specific use case.
2.5. Task specific evaluation
Depending on the task, other evaluation methods are appropriate. For instance, when testing a categorization LLM, accuracy can be measured using a predefined test set alongside a simple equality check (of the predicted category vs. actual category). Similarly, the structure of outputs can be tested by counting line-breaks; certain headers and/or the presence of certain keywords can also be checked. Although these technique are not easily generalizable across different use cases, they offer a precise and efficient way to verify model performance.
Putting things together: writing an eval to measure LLM summarization performance
Consider a scenario where you're developing an LLM-powered summarization feature designed to condense large volumes of information into three structured sections. To ensure high-quality performance, we evaluate the model for each of our five evaluation criteria. For each criterion, we identify a key question that guides the evaluation. This question helps define the precise metric needed and determines the appropriate method for calculating it.
Criterium | Key question | Metric | How |
Correctness | Is the summary free from hallucinations? | Number of statements in summary that can be verified based on source text | * Use an LLM-as-a-judge to check if each statement can be answered based on the source texts * Use human evaluation to verify correctness of outputs |
Relevance | Is the summary complete? | Number of key elements present with respect to a reference guideline or golden standard summary | Cross-reference statements in summaries with LLM-as-a-judge and measure the overlap |
Is the summary concise? | Number of irrelevant statements with respect to golden standard Length of summary | * Cross-reference statements in summaries with LLM-as-a-judge and measure the overlap * Count the number of words of generated summaries | |
Robustness | Is the model prone to noise in the input text? | Similarity of summary generated for original text with respect to summary generated for text with noise such as typo’s and inserted irrelevant information | Compare statements with LLM-as-a-judge, or compare textual similarity with ROUGE or BERTscore |
Instruction & restriction adherence | Does the summary comply with required structure? | Presence of three structured sections | Count number of line breaks and check presence of headers |
Writing style | Is the writing style professional, fluent and free of grammatical errors? | Rating of tone-of-voice, fluency and grammar | * Ask LLM-as-a-judge to rate fluency and professionality and mark grammatical errors * Rate writing style with human evaluation |
Overarching: alignment with golden standard | Do generated summaries align with golden standard summaries? | Textual similarity with respect to golden standard summary | Calculate similarity with ROUGE or BERTscore |
The table shows that the proposed evaluation strategy leverages multiple tools and combines reference-based and reference-free assessments to ensure a well-rounded analysis. And so we ensure that our summarization model is accurate, robust, and aligned with real-world needs. This multi-layered approach provides a scalable and flexible way to evaluate LLM performance in diverse applications.
Final thoughts
Managing LLM output quality is challenging, yet crucial to build robust and reliable applications. To ensure success, here are a few tips:
- Proactively define the evaluation criteria. Establish clear quality standards before model deployment to ensure a consistent assessment framework.
- Automate when feasible. While human evaluation is essential for subjective aspects, automate structured tests for efficiency and consistency.
- Leverage GenAI to broaden your evaluation. Use LLMs to generate diverse test prompts, simulate user queries, and assess robustness against variations like typos or multi-language inputs.
- Avoid reinventing the wheel. There are already various evaluation frameworks available on the internet (for instance, DeepEval). These frameworks provide structured methodologies that combine multiple evaluation techniques.
Achieving high-quality output is only the beginning. Generative AI systems require continuous oversight to address challenges that arise after deployment. User interactions can introduce unpredictable edge cases, exposing the gap between simulated scenarios and real-world usage. In addition, updates to models and datasets can impact performance, making continuous evaluation crucial to ensure long-term success. At Rewire, we specialize in helping organizations navigate the complexities of GenAI, offering expert guidance to achieve robust performance management and deployment success. Ready to take your GenAI solution to the next level? Let’s make it happen!
This article was written by Gerben Rijpkema, Data Scientist at Rewire, and Renske Zijm, Data Scientist at Rewire.
Steeped in history, built for the future, the new Rewire office in Amsterdam is a hub for innovation, collaboration, and impact
Rewire has taken a new step forward: a new home in Amsterdam! But this isn’t just an office. It’s a reflection of our growth, vision, and commitment to driving business impact through data & AI. With this new office, we aim to create an inspiring home away from home for our employees, and a great place to connect and collaborate with our clients and partners.
Steeped in history, built for the future
Amsterdam is a city where history and innovation coexist. Our new office embodies this spirit. Nestled in scenic Oosterpark, which dates back to 1891, and set in the beautifully restored Amstel Brewery stables from 1912, our location is a blend of tradition and modernity.
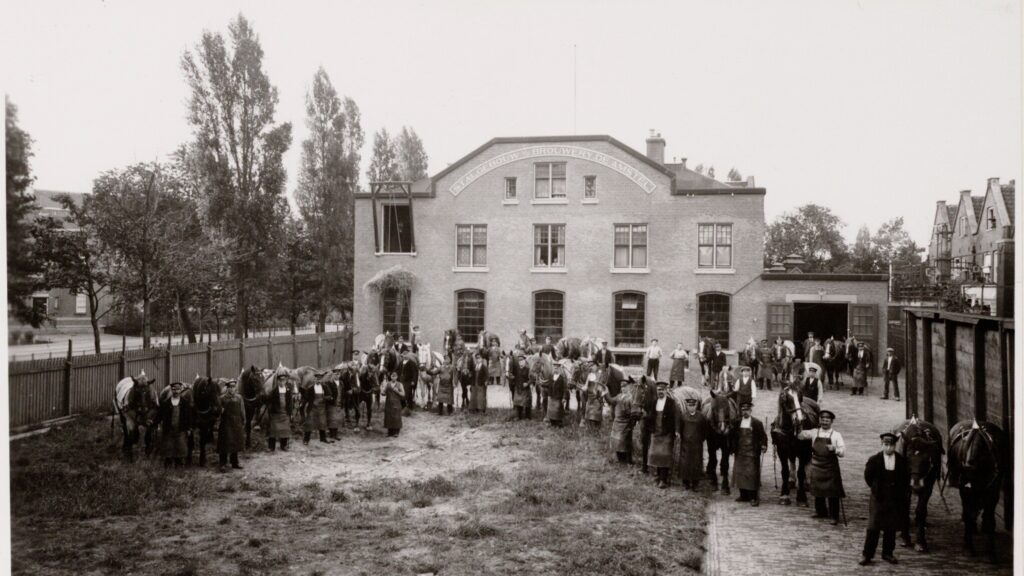
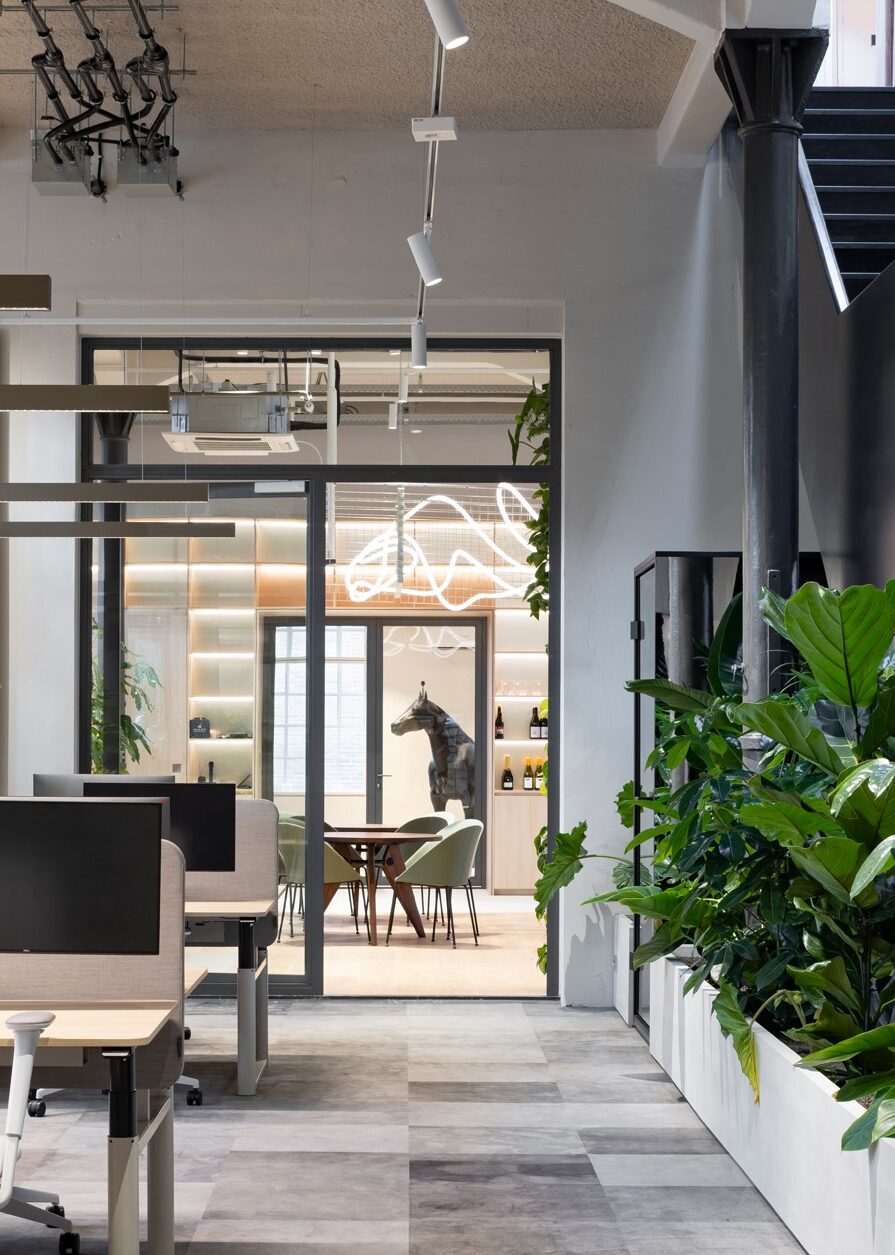
The fully refurbished Rewire office in Amsterdam once served as the stables of the Amstel brewery.
But this isn’t just about aesthetics—our presence within one of Amsterdam’s most vibrant areas is strategic. Surrounded by universities, research institutions, cultural and entertainment landmarks, we’re positioned at the crossroads of academia, industry, and creativity. Moreover, the city has established itself as a global hub for Data & AI, attracting global talent, startups, research labs, and multinational companies. Thus, we’re embedding ourselves in an environment that fosters cross-disciplinary collaboration and promotes impact-driven solutions. All in all, our new location ensures that we stay at the forefront of AI while providing an inspiring setting to work, learn, and imagine the future.
A space for collaboration & growth
At Rewire, we believe that knowledge-sharing and hands-on learning are at the core of meaningful AI adoption. That’s why our new Amsterdam office is more than just a workspace—it’s a hub for collaboration, education, and innovation.
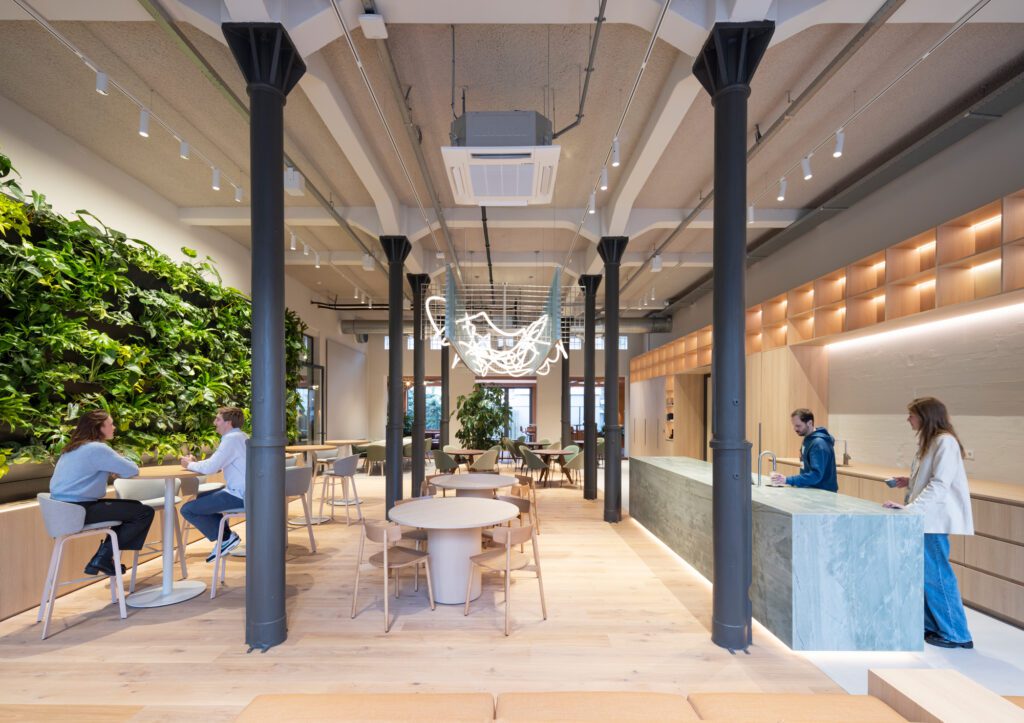
More than just an office, it's a hub for collaboration, education, and innovation.
We’ve created dedicated spaces for GAIN’s in-person training, workshops, and AI bootcamps, reinforcing our commitment to upskilling talent and supporting the next generation of AI professionals. Whether through hands-on coding sessions, strategic AI leadership discussions, or knowledge-sharing events, this space is designed to develop the Data & AI community of tomorrow.
Beyond structured learning, we’ve designed our office to be an environment where teams can engage in deep problem-solving, collaborate on projects, and push the boundaries of what can be achieved. Our goal is to bridge the gap between research and real-world application, thus helping clients leverage AI’s full potential.
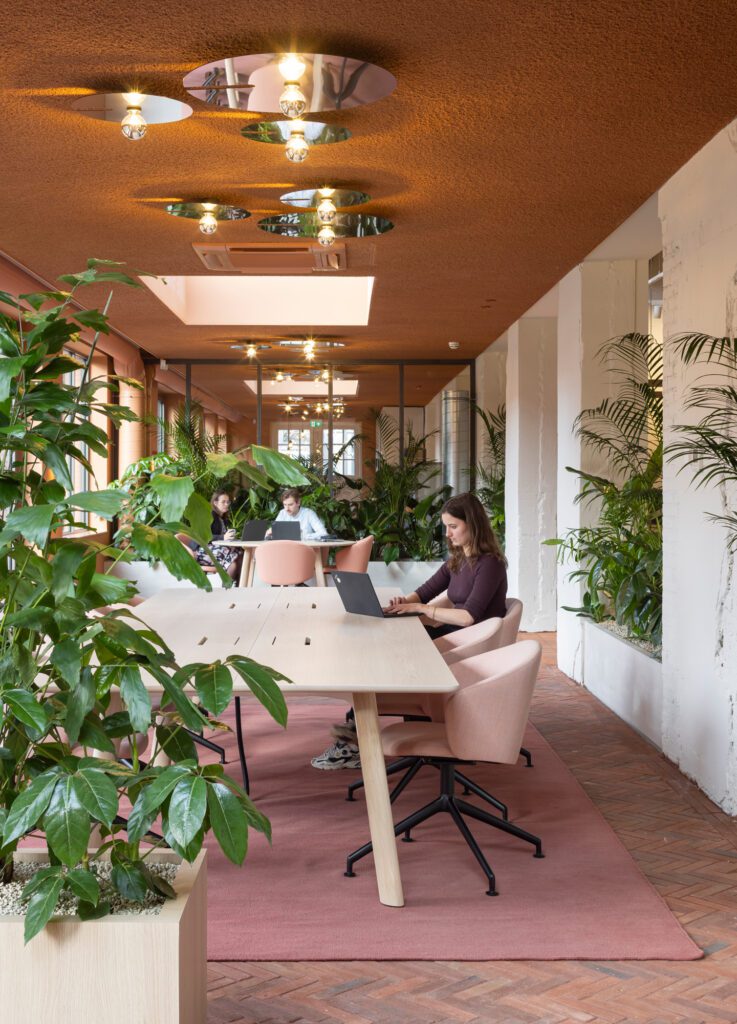
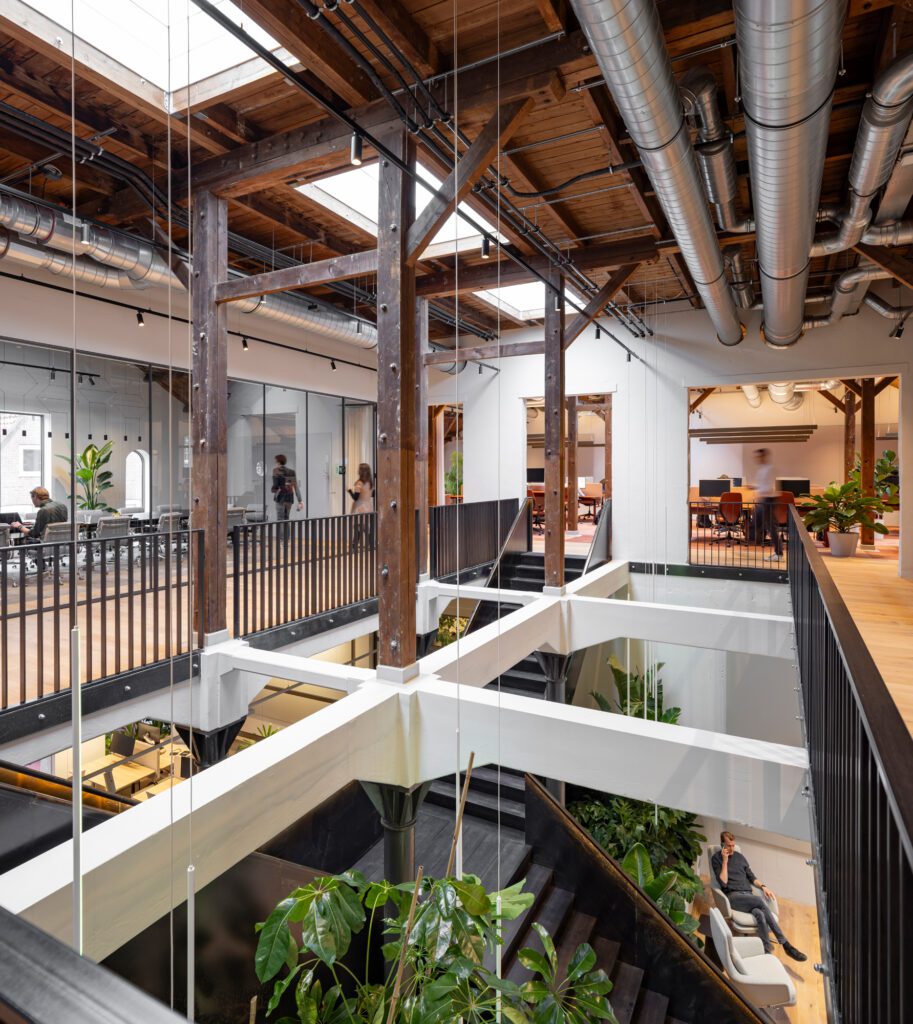
The new office includes a variety of spaces, from small quiet spaces for deep thinking to large open spaces for group work, and socializing.
Sustainability & excellence at the core
Our new office is built to the highest standards of quality and sustainability, incorporating modern energy-efficient design, eco-friendly materials, and thoughtfully designed workspaces.
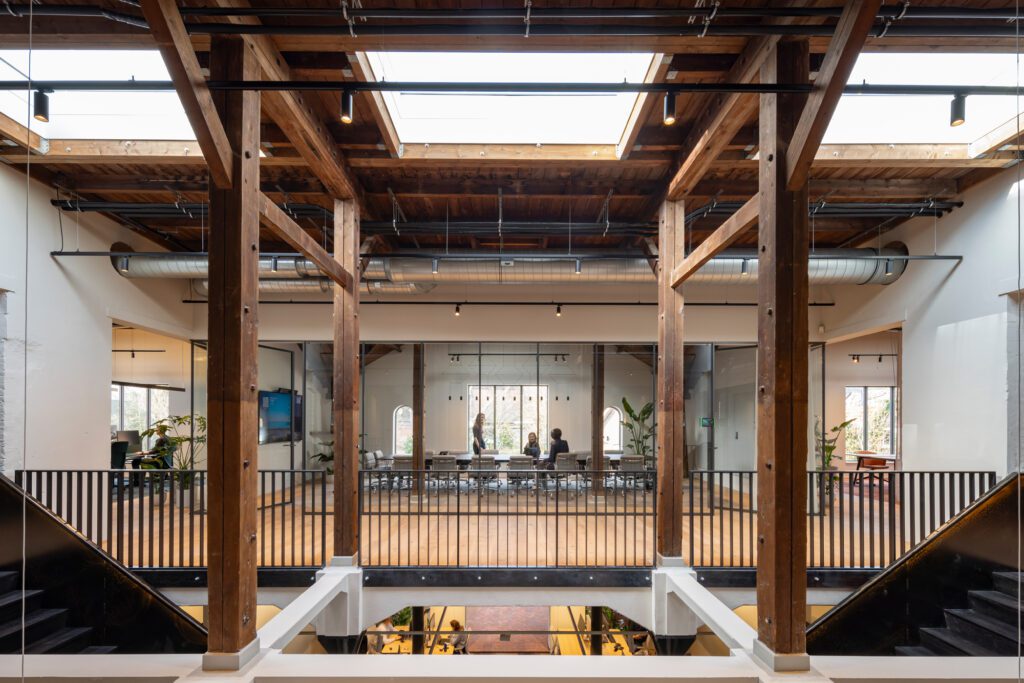
Eco-friendly and energy efficient, the new office retain the fixtures of the original Amstel stables.
We’ve curated an office that balances dynamic meeting spaces, collaborative areas, and quiet zones for deep thinking—all designed to support flexibility, focus, and innovation. Whether brainstorming the next AI breakthrough or engaging in strategic discussions, our employees have a space that fosters creativity, problem-solving, and impactful decision-making.
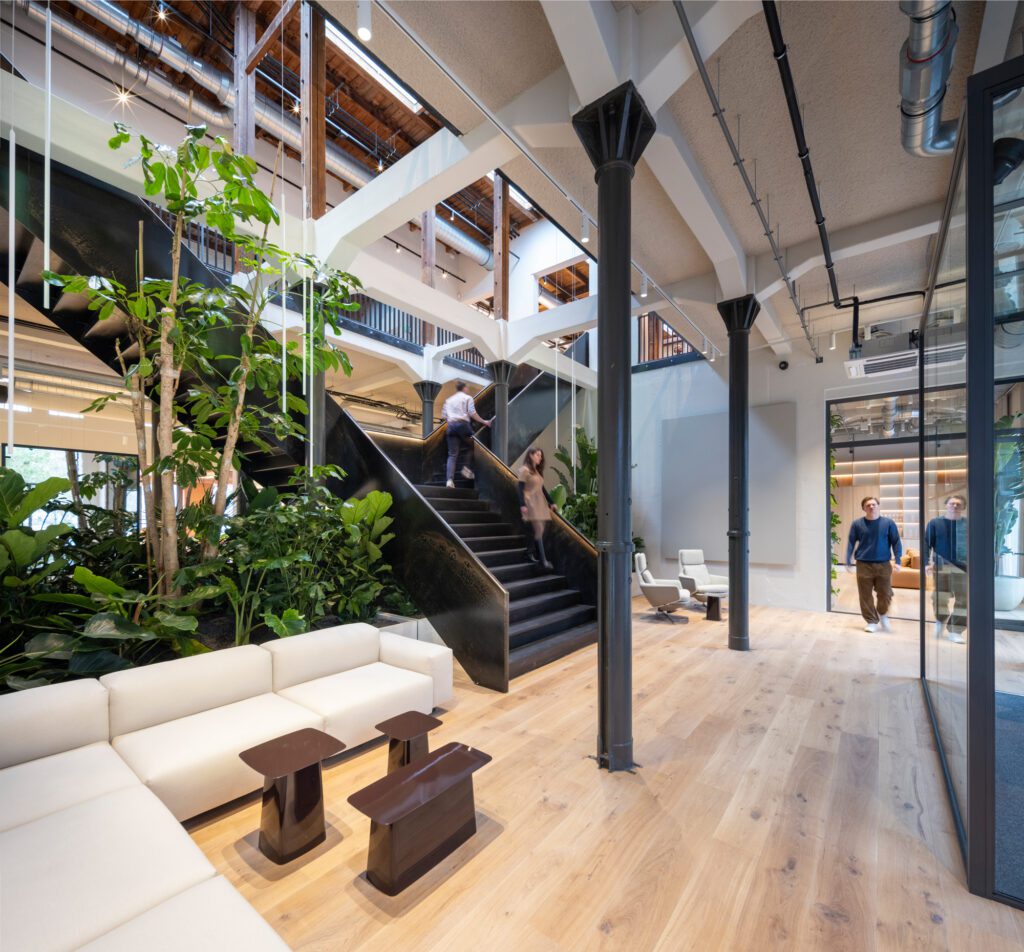
The office is not just next to Oosterpark, one of Amsterdam's most beautiful parks. It is also home to thousands of plants.
We’re Just getting started
Our move to a new office in Amsterdam represents a new chapter in Rewire’s journey, but this is only the beginning. As we continue to expand, build, and collaborate, we look forward to engaging with the broader Data & AI community, fostering innovation, and shaping the future of AI-driven impact.
We’re excited for what’s ahead. If you’re interested in working with us, partnering on AI initiatives, or visiting our new space—contact us!
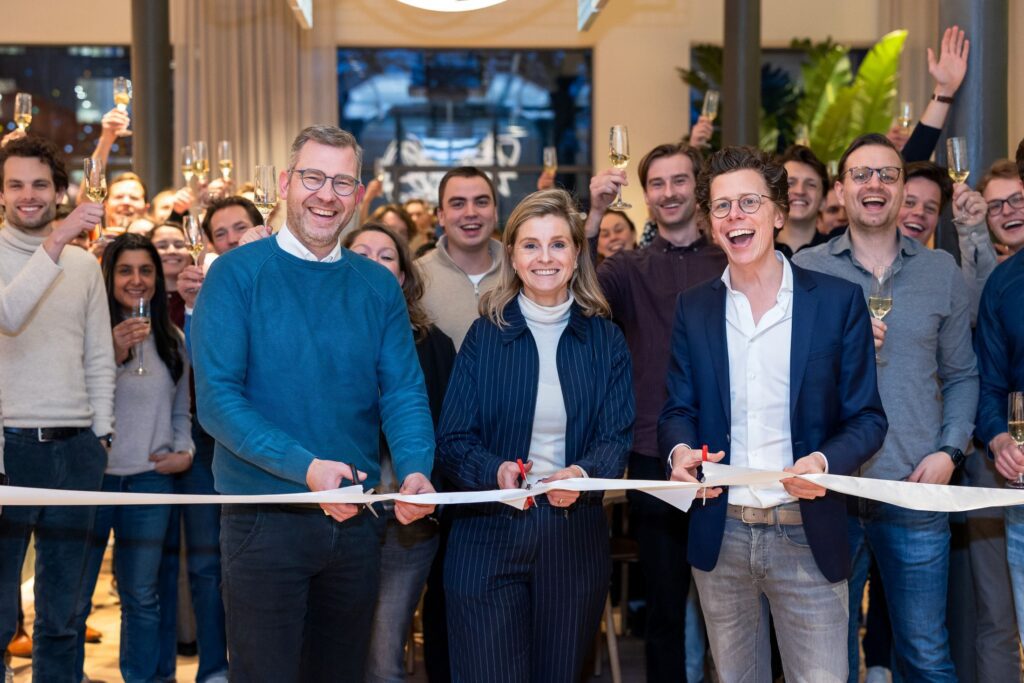
The team that made it happen.
How a low-cost, open-source approach is redefining the AI value chain and changing the reality for corporate end-users
At this point, you’ve likely heard about it: DeepSeek. Founded in 2023 by Liang Wenfeng, co-founder of the quantitative hedge fund High-Flyer, this Hangzhou-based startup is rewriting the rules of AI development with its low-cost model development, and open-source approach.
Quick recap first.
From small steps to giant leaps
There are actually two model families launched by the startup: DeepSeek-V3 and DeepSeek R1.
V3 is a Mixture-of-Experts (MoE) large language model (LLM) with 671 billion parameters. Thanks to a number of optimizations it can provide similar or better performance than other large foundational models, such as GPT-4o and Claude-3.5-Sonnet. What’s even more remarkable is that V3 was trained in around 55 days at a fraction of the cost for similar models developed in the U.S. —less than US$6 million for DeepSeek V3, compared with tens of millions, or even billions, of dollars in investments for its Western counterparts.
R1, released on January 20, 2025, is a reasoning LLM that uses innovations applied to the V3 base model to greatly improve its performance in reasoning. DeepSeek-R1 achieves performance comparable to OpenAI-o1 across math, code, and reasoning tasks. The kicker is that R1 is published under the permissive MIT license: this license allows developers worldwide to modify the model for proprietary or commercial use, which paves the way for accelerated innovation and adoption.
Less is more: reshaping the AI value chain
As other companies emulate DeepSeek’s achievements, its cost-effective approach and open-sourcing of its technology signals three upcoming shifts:
1. Lower fixed costs and increased competition. DeepSeek shows that cutting-edge LLMs no longer require sky-high investments. Users have reported running the V3 model on consumer Mac hardware and even foresee its use on devices as lightweight as a Raspberry Pi. This opens the door for more smaller players to develop their own models.
2. Higher variable costs driven by higher query costs. As reasoning models become widely available and more sophisticated, their per-query costs rise due to the energy-intensive reasoning processes. This dynamic creates new opportunities for purpose-built models – as opposed to general-purpose models like OpenAI’s ChatGPT.
3. Greater value creation down the AI value chain. As LLM infrastructure becomes a commodity (much like electricity) and companies develop competing models that dent the monopolistic power of big tech (although the latter will continue to push the boundaries with new paradigm breakthroughs), the development of solutions in the application layer – where most of the value for end-users resides – becomes much more accessible and cost-effective. Hence, we expect accelerated innovations in the application layer and the AI market to move away from “winner-takes-all” dynamics, with the emergence of diverse applications tailored to specific industries, domains, and use cases.
Transform potential into performance with GenAI
Whether you win or lose in your market may soon rely on having the best GenAI capability and the data foundations to support it. We can help you build this.
Discuss your needsSo what’s next for corporate end-users?
Most corporate end-users will find that having a reliable and “good enough” model matters more than having the absolute best model. Advances in reasoning such as R1 could be a big step for AI agents that deal with customers and perform tasks in the workplace. If those are available more cheaply, corporate adoption and bottom lines will increase.
Taking a step back, a direct consequence of the proliferation of industry and function-specific AI solutions built in the application layer is that AI tools and capabilities will become a necessary component for any company seeking to build competitive advantage.
At Rewire, we help clients prepare themselves for this new reality by building solutions and capabilities that leverage this new “AI commodity” and turning AI potential into real-world value. To explore how we can help you harness the next wave of AI, contact us.
From customer support to complex problem-solving: exploring the core components and real-world potential of Agentic AI systems
For decades, AI has captured our imaginations with visions of autonomous systems like R2-D2 or Skynet, capable of independently navigating and solving complex challenges. While those pictures remain firmly rooted in science fiction, the emergence of Agentic AI signals an exciting step in that direction. Powered by GenAI models, these systems would improve adaptability and decision-making beyond passive interactions or narrow tasks.
Consider the example of e-commerce, where customer support is critical. Traditional chatbots handle basic inquiries, but when a question becomes more complex—such as tracking a delayed shipment or providing tailored product recommendations—the need for human intervention quickly becomes apparent. This is where GenAI agents can step in, bridging the gap between basic automation and human-level problem solving.
The promise of GenAI-driven agents isn’t about overnight industry transformation but lies in their ability to augment workflows, enhance creative processes, and tackle complex challenges with a degree of autonomy. Yet, alongside this potential come significant technological, ethical, and practical challenges that demand thoughtful exploration and development.
In this blog, we’ll delve into what sets these systems apart, examine their core components, and explore their transformative potential through real-world examples. Let’s start by listing a few examples of possible use cases for GenAI agents:
- Personal assistants that schedule meetings based on availability and send out invitations.
- Content creators that generate blog posts, social media copy, and product descriptions with speed and precision.
- Code assistants that assist developers in writing, debugging, and optimizing code.
- Healthcare assistants that analyze medical records and provide diagnostic insights.
- AI tutors that personalize learning experiences, offering quizzes and tailored feedback to students.
GenAI agents handle tasks that previously required significant human effort, freeing up valuable time and resources for strategic thinking and innovation. But what exactly are these GenAI agents, how do they work and how to design them?
Understanding the core components of GenAI agents
Unlike traditional chatbots, GenAI agents transcend simple text generation, captured in three core principles:
- They act autonomously. These agents are capable of taking goal-driven actions, such as querying a database or generating a report, without explicit human intervention.
- They plan and reason. Leveraging advanced reasoning capabilities, they can break down complex tasks into actionable steps.
- They integrate with tools. While LLMs are great for answering questions, GenAI agents use tools and external systems to access real-time data, perform calculations, or retrieve historical information.
What makes these capabilities possible? At the heart of every GenAI agent lies the power of GenAI models, specifically large language models (LLMs). LLMs are the engine behind the agent's ability to understand natural language, adapt to diverse tasks, and simulate reasoning. Without them, these agents couldn’t achieve the nuanced communication or versatility required for autonomy or to handle complex inputs.
But how do all the pieces come together to create such a system? To understand the full picture, we need to look beyond LLMs and examine the other key components that make GenAI agents work. Together, these components (Interface, Memory, Planning, Tools, and Action) enable the agent to process information, make decisions, and execute tasks. Let’s explore each of these building blocks in detail.
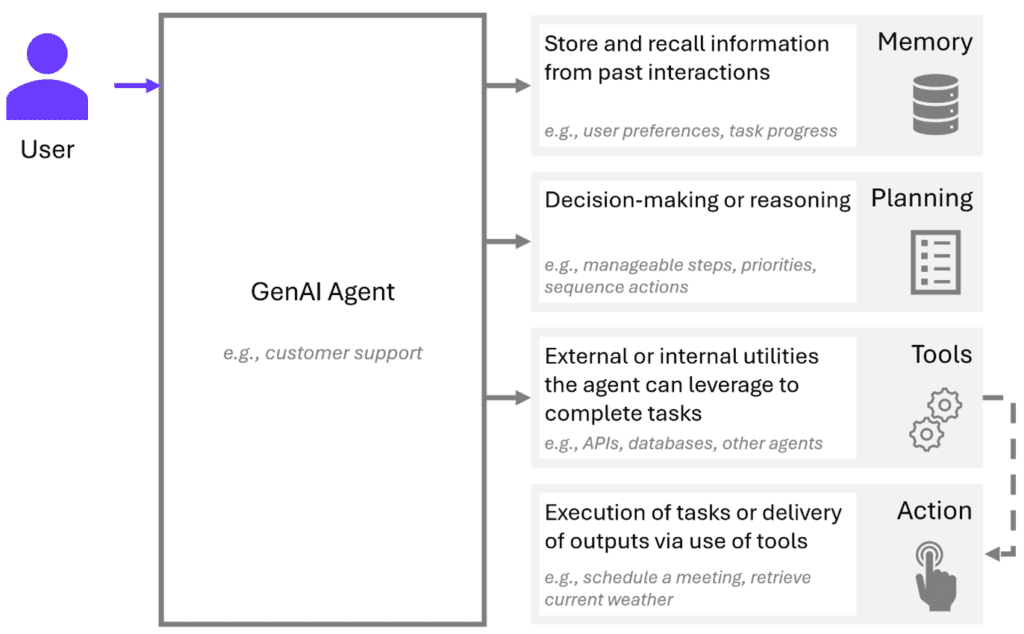
1. Interface: the bridge between you and the AI
The interface is the gateway through which users communicate with the GenAI agent. It serves as the medium for input and output, allowing users to ask questions, give commands, or provide data. Whether it’s a text-based chat, a voice command, or a more complex graphical user interface (GUI), the interface ensures the agent can understand human input and convert it into actionable data.
2. Memory: remembering what matters
Memory is what allows a GenAI agent to learn from past experiences and adapt over time. It stores both short-term and long-term information, helping the agent maintain context across interactions and deliver personalized experiences, based on past conversations and preferences.
3. Planning: charting the path to success
The planning component is the brain behind the agent’s decision-making process. This is essentially using the reasoning of an LLM to break down the problem into smaller tasks. When faced with a task or problem, the agent doesn’t just act blindly. Instead, it analyses the situation, sets goals and priorities, and devises a strategy to accomplish them. This ability to plan ensures that the agent doesn’t simply react in predefined ways, but adapts its actions for both simple and complex scenarios.
4. Tools: extending the agent’s capabilities
No GenAI agent is an island— it needs to access additional resources to solve more specialized problems. Tools can be external resources, APIs, databases, or even other specialized (GenAI) agents that the agent can use to extend its functionality. By activating tools as prompted by the GenAI agent when needed, the agent can perform tasks that go beyond its core abilities, making it more powerful and versatile.
5. Action: bringing plans to life
Once the agent has formulated a plan, it’s time to take action. The action component is where the agent moves from theory to practice, executing tasks, sending responses, or interacting with tools and external systems. It’s the moment where the GenAI agent delivers value by fulfilling its purpose, completing a task, or responding to a user request.
The core components of a GenAI agent in action
Now that we’ve broken down the core components of a GenAI agent, let’s see how they come together in a real-world scenario. Let’s get back to our customer support example and imagine a customer who is inquiring about the status of their order. Here’s how the agent seamlessly provides a thoughtful, efficient response:
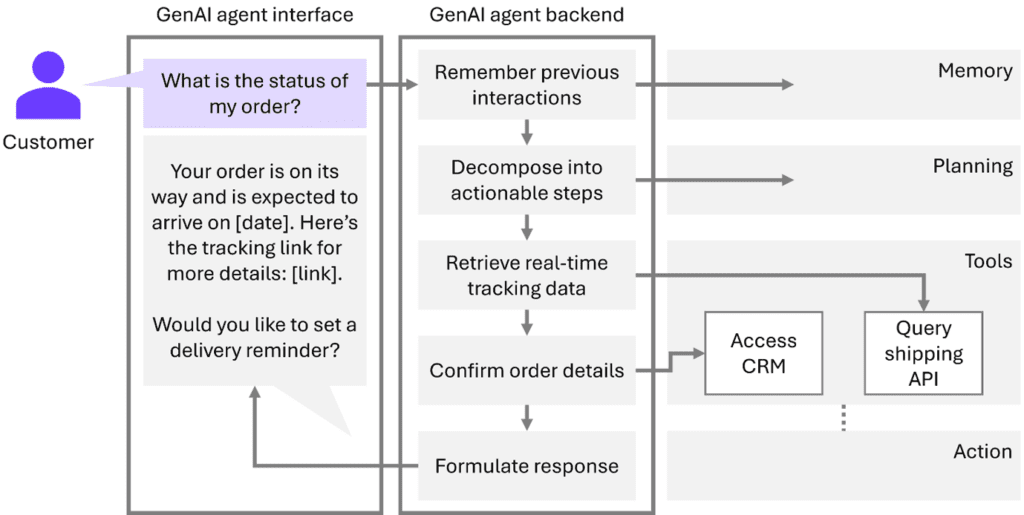
- Interface: The agent receives the customer query, "What is the status of my order?"
- Memory: The agent remembers the customer placed an order on January 15th 2025 with order ID #56789 from a previous interaction.
- Planning: The agent breaks down the task into steps: retrieve order details, check shipment status, and confirm delivery date.
- Tools: The agent accesses the DHL API with the customer’s order reference to get the most recent status update and additionally accesses CRM to confirm order details.
- Action: The agent retrieves the shipping status, namely “Out for delivery” as of January 22nd, 2025.
- Interface: The agent sends the response back to the customer: "Your order is on its way and is expected to arrive on January 22nd, 2025. Here’s the tracking link for more details: [link]. Would you like to set a delivery reminder?"
If the customer opts to set a reminder, the agent can handle this request as well by using its available tools to schedule the reminder accordingly, something that basic automation would not be able to do.
Unlocking efficiency with multi-agent systems for complex queries
A single GenAI agent can efficiently handle straightforward queries, but real-world customer interactions often involve complex, multifaceted issues. For instance, a customer may inquire about order status while also addressing delayed shipments, refunds, and promotions—all in one conversation. Managing such tasks sequentially with one agent increases the risk of delays and errors.
This is where multi-agent systems come in. By breaking down tasks into smaller, specialized subtasks, each handled by a dedicated agent, it’s easier to ensure and track efficiency and accuracy. For example, one agent handles order tracking, another manages refunds, and a third addresses promotions.
Splitting tasks this way unlocks several benefits: simpler models can tackle reduced complexity, agents can be given specific instructions to specialize in certain areas, and parallel processing allows for approaches like majority voting to build confidence in outputs.
With specialized GenAI agents working together, businesses can scale support for complex queries while maintaining speed and precision. This multi-agent approach outperforms traditional customer service, offering a more efficient and accurate solution than escalating issues to human agents.
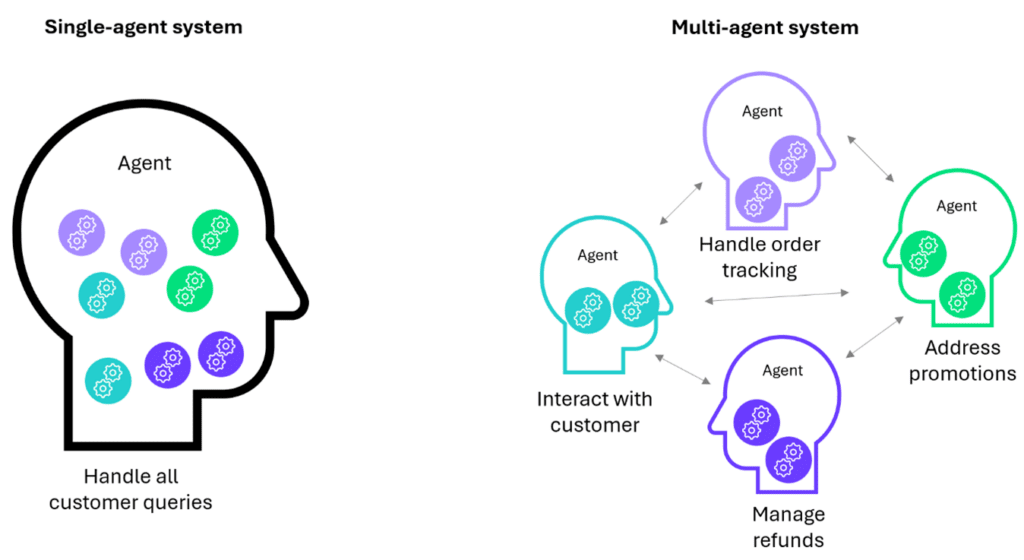
What is needed to design GenAI agents?
Whether you’re automating customer support, enhancing business processes, or revolutionizing healthcare, building effective GenAI agents is a strategic endeavor that requires expertise across multiple disciplines.
At the core of building a GenAI agent is the integration of key components that enable its functionality and adaptability:
- Data engineering. The foundation of any GenAI agent is its ability to access and process data from multiple sources. This includes integrating APIs, databases, and real-time data streams, ensuring the agent can interact with the world outside its own environment. Effective data engineering enables an agent to access up-to-date information, making it dynamic and capable of handling a variety of tasks.
- Prompt engineering. For a large language model to perform effectively in a specific domain, it must be tailored through prompt engineering. This involves crafting the right inputs to guide the agent’s responses and behavior. Whether it’s automating customer inquiries or analyzing medical data, domain-specific prompts ensure that the agent’s actions are relevant, accurate, and efficient.
- Experimentation. Designing workflows for GenAI agents requires balancing autonomy with accuracy. During experimentation, developers must refine the agent’s decision-making process, ensuring it can handle complex tasks autonomously while maintaining the precision needed to deliver valuable results. This iterative process of testing and optimizing is key to developing agents that operate efficiently in real-world scenarios.
- Ethics and governance. With the immense potential of GenAI agents comes the responsibility to ensure they are used ethically. This means designing systems that protect sensitive data, comply with regulations, and operate transparently. Ensuring ethical behavior isn’t just about compliance—it’s about building trust with users and maintaining accountability in AI-driven processes.
Transform potential into performance with GenAI
Whether you win or lose in your market may soon rely on having the best GenAI capability and the data foundations to support it. We can help you build this.
Discuss your needsChallenges and pitfalls with GenAI agents
While GenAI agents hold tremendous potential, there are challenges that need careful consideration:
- Complexity in multi-agent systems. Coordinating multiple specialized agents can introduce complexity, especially in ensuring seamless communication and task execution.
- Cost vs. benefit. With resource-intensive models, balancing the sophistication of GenAI agents with the cost of deployment is crucial.
- Data privacy and security. As GenAI agents require access to vast data and tools, ensuring the protection of sensitive information becomes a top priority.
- Ethical and regulatory considerations. Bias in training data, dilemmas around autonomous decision-making, and challenges in adhering to legal and industry-specific standards create significant risks. Deploying these agents responsibly requires addressing ethical concerns while navigating complex regulatory frameworks to ensure compliance.
- Performance management. Implementing agent systems effectively requires overcoming the complexity of monitoring and optimizing their performance. From breaking down reasoning steps to preparing systems and data for smooth access, managing the performance of these agents as they scale remains a significant hurdle.
Unlocking the future of GenAI agents with insights and discoveries ahead - stay tuned!
GenAI agents represent an exciting shift in how we approach problem-solving and human-computer interaction. With their promise to reason, plan, and execute tasks autonomously, these agents have the potential to tackle even the most complex workflows. As we continue our exploration of these systems, our focus will remain on understanding both their immense promise and the challenges they present.
In the coming weeks, we will delve deeper into the areas where GenAI agents excel, where they struggle, and what can be done to enhance their real-world effectiveness. We invite you to follow along with us as we share our experiments, findings, and practical insights from this ongoing journey. Stay tuned for our next blog post, where we will explore the evolving landscape of GenAI agents, along with the valuable lessons we have learned through experimentation.
This article was written by Mirte Pruppers, Data Scientist, Phebe Langens, Data Scientist, and Simon Koolstra, Principal at Rewire.
Jonathan Dijkslag on growing and maintaining the company's edge in biotechnology and life sciences
In the latest episode of our podcast, Jonathan Dijkslag, Global Manager of Data Insights & Data Innovation at Enza Zaden, one of the world’s leading vegetable breeding companies sits down with Ties Carbo, Principal at Rewire. With over 2,000 employees and operations in 30 countries, Enza Zaden has built its success on innovation, from pioneering biotechnology in the 1980s to embracing the transformative power of Data & AI today.
Jonathan shares insights into the company’s data-driven journey, the challenges of integrating traditional expertise with modern technology, and how cultivating a culture of trust, speed, and adaptability is shaping Enza Zaden’s future. Tune in to discover how this Dutch leader is using data to revolutionize vegetable breeding and stay ahead in a competitive, innovation-driven industry.
Watch the full interview
The transcript below has been edited for clarity and length.
Ties Carbo: Thank you very much for joining this interview. Can you please introduce yourself?
Jonathan Dijkslag: I work at Enza Zaden as the global manager of Data Insights and Innovation. My mission is to make Enza Zaden the most powerful vegetable-breeding company using data.
"It's about how to bring new skills, new knowledge to people, and combine it all together to deliver real impact."
Jonathan Dijkslag
Ties Carbo: Can you tell us a little bit about your experience on working with Data & AI?
Jonathan Dijkslag: What made Enza very successful is its breeding expertise. We are working in a product-driven market and we are really good at it. Enza grew over time thanks to the expertise of our people. In the 80s, biotech came with it. And I think we were quite successful in adapting to new technologies.
Now we are running into an era where data is crucial. So I think that data is at the heart of our journey. We are a company that values our expertise. But also we are really aware of the fact that expertise comes with knowledge, and with having the right people able to leverage that knowledge in such a way that you make impact. And that's also how we look at this journey. So it's about how to bring new skills, new knowledge to people, and combine it all together to deliver real impact.
Ties Carbo: It sounds like you're in a new step of a broader journey that started decades ago. And now, of course, Data & AI is a bit more prevalent. Can you tell us a little bit more about some successes that you're having on the Data & AI front?
Jonathan Dijkslag: There are so many, but I think the biggest success of Enza is that with everything we do, we are a conscious of our choices. Over the past three to five years, we have spent quite some money on the foundation for success. So where some companies are quite stressed out because of legacy systems, we do very well in starting up the right initiatives to create solid foundations and then take the time to finalize them. And I think that is very powerful within Enza. So over the past years we have built new skills based on scalable platforms in such a way that we can bring real concrete value in almost all fields of expertise in the company.
Ties Carbo: Can you maybe give a few examples of some challenges you encountered along the way?
Jonathan Dijkslag: I think the biggest challenge is that the devil is in the details. That's not only from a data journey perspective, but also in our [functional] expertise. So the real success of Enza with data depends on really high, mature [functional] expertise combined with very high, mature expertise in data. And bridging functional experts with data experts is the biggest challenge. It has always been the biggest challenge. But for a company like Enza, it's particularly complex.
Another complexity is we have quite some people who for decades have used data heavily in their daily work. Yet the way we use data nowadays is different than compared to decades ago. So the challenge is how to value the contributions made today and at the same time challenge people to take the next step with all the opportunities we see nowadays.
"We learned the hard way that if you don't understand – or don't want to understand what our purpose is as a company, then you will probably not be successful."
Jonathan Dijkslag
Ties Carbo: I was a bit intrigued by your first response – bridging the functional expertise with the Data & AI expertise. What's your secret to doing that?
Jonathan Dijkslag: I think it really comes back to understanding the culture of your company. When I joined the company, I knew that it would take time to understand how things work today. I needed to show my respect to everything that has brought so much success to the company in order to add something to the formula.
Every company has a different culture. Within Enza, the culture is pretty much about being Dutch. Being very direct, clear, result-driven, don't complain. Just show that you have something to add. And that's what we try to do. And learn, learn and adapt. For instance, in the war on talent, we made some mistakes. We thought maybe business expertise is not always that important. But we learned the hard way that if you don't understand – or don't want to understand what our purpose is as a company, then you will probably not be successful. And that means something to the pace and the people you can attract to the company. So it’s all about understanding the existing culture, and acting on it. And sometimes that means that you need more time than you’d like to have the right people or have the right projects finalized and create the impact you want.
"We have to create a mechanism whereby we're not too busy. We have to create a change model where you can again and again adapt to new opportunities."
Jonathan Dijkslag
Ties Carbo: What advice would you give to other companies that embark on a journey like this?
Jonathan Dijkslag: This is the new normal. The complexity is the new normal. So we have to think about how we can bring every day, every week, every month, every year more and more change. And people tend to say “I'm busy, we’re busy, wait, we have to prioritize.” I think we have to rethink that model. We have to create a mechanism whereby we're not too busy. We have to create a change model where you can again and again adapt to new opportunities. And I think this is all about creating great examples. So sometimes it’s better to make fast decisions that afterwards you would rate with a seven or an eight, but you did it fast. You were very clear and you made sure that the teams work with this decision that’s rated seven, rather than thinking for a long period about the best business case or the best ROI. So I think the speed of decision-making close to the area impact. I think that that's the secret.
People talk a lot about agility. I think for me, the most important part of agility is the autonomy to operate. Combined with very focused teams and super fast decision-making and the obligation to show what you did.
Ties Carbo: How is it to work with Rewire?
Jonathan Dijkslag: For me, it's very important to work with people who are committed. I have a high level of responsibility. I like to have some autonomy as well. And to balance those things, I think it's very important to be result-driven and also to show commitment in everything you do. And what we try to do in our collaboration with Rewire is to create a commitment to results. Not only on paper, but instead both parties taking ownership of success. And we measure it concretely. That's the magic. We're really in it together and there's equality in the partnership. That's how it feels for me. So I think that that's the difference.
We work a lot with genetic data. So our challenges are very specific. Life science brings some complexity with it. And we were looking for a partner who can to help us develop the maturity to understand and work with confidence with the corresponding data. And thanks to their experience in the field of life science, they showed that they understand this as well. And that's very important because trust is not easily created. And if you show that you brought some successes in the world of life science - including genetic data - that helps to get the trust, and step into realistic cases.
Ties Carbo: Thank you.
About the authors
Jonathan Dijkslag is the Global Manager of Data Insights & Data Innovation at Enza Zaden, where he drives impactful data strategies and innovation in one of the world's leading vegetable breeding companies. With over 15 years of experience spanning data-driven transformation, business insights, and organizational change, Jon has held leadership roles at Pon Automotive, where he spearheaded transitions to data-driven decision-making and centralized business analytics functions. Passionate about aligning technology, culture, and strategic goals, Jon is dedicated to creating tangible business impact through data.
Ties Carbo is Principal at Rewire.
Allard de Boer on scaling data literacy, overcoming challenges, and building strong partnerships at Adevinta - owner of Marktplaats, Leboncoin, Infojobs and more.
In this podcast, Allard de Boer, Director of Analytics at Adevinta (a leading online classifieds which includes brands like Marktplaats, Leboncoin, mobile.de, and many more) sits down with Rewire Partner Arje Sanders to explore how the company transformed its decision-making process from intuition-based to data-driven. Allard shares insights on the challenges of scaling data and analytics across Adevinta’s diverse portfolio of brands.
Watch the full interview
The transcript below has been edited for clarity and length.
Arje Sanders: Can you please introduce yourself and tell us something about your role?
Allard de Boer: I am the director of analytics at Adevinta. Adevinta is a holding company that owns multiple classifieds brands like Marktplaats in the Netherlands, Mobile.de in Germany, Leboncoin in France, etc. My role is to scale the data and analytics across the different portfolio businesses.
Arje Sanders: How did you first hear about Rewire?
Allard de Boer: I got introduced to Rewire [then called MIcompany] six, seven years ago. We started working on the Analytics academy for Marktplaats and have worked together since.
We were talking about things like statistical significance, but actually only a few people knew what that actually meant. So we saw that there are limits to our capabilities within the organization.
Allard de Boer
Arje Sanders: Can you tell me a little about the challenges that you were facing and wanted to resolve in that collaboration? What were your team challenges?
Allard de Boer: Marktplaats is a technology company. We have enormous technology, we have a lot of data. Every solution that employees want to create has a foundation in technology. We had a lot of questions around data and analytics and every time people threw more technology at it. That ran into limitations because the people operating the technology were scarce. So we needed to scale in a different way.
What we needed to do is get more people involved in our data and analytics efforts and make sure that it was a foundational capability throughout the organization. This is when we started thinking about how to scale specific use cases further. See how we can take what we have, but then make it common throughout the whole organization and then scale specific use cases further.
For example, one problem is that we did a lot of A/B testing and experimentation. Any new feature on Marktplaats was tested through A/B testing. It was evaluated on how it performed on customer journeys, and how it performed on company revenue. This was so successful that we wanted to do more experiments. But then we ran into the limitations of how many people can look at the experimentation, and how many people understand what they're actually looking at.
We were talking about things like statistical significance, but actually only a few people knew what that actually meant. So we saw that there are limits to our capabilities within the organization. This is where we started looking for a partner that can help us to scale employee education to raise the level of literacy within our organization. That's how we came up with Rewire.
We were an organization where a lot of gut feeling decision-making was done. We then shifted to data-driven decision-making and instantly saw acceleration in our performance.
Allard de Boer
Arje Sanders: That sounds quite complex because I assume you're talking about different groups of people with different capability levels, but also over different countries. How did you approach the challenge?
Allard de Boer: Growth came very naturally to us because we had a very good model. Marktplaats was growing very quickly in the Netherlands. Then after a while, growth started to flatten out a bit. We needed to rethink how we run the organization. We moved towards customer journeys and understanding customer needs.
Understanding those needs is difficult because we're a virtual company. Seeing what customers do, understanding what they need is something we need to track digitally. This is why the data and analytics is so vital for us as a company. If you have a physical store, you can see how people move around. If your store is only online, the data and analytics is your only corridor to understanding what the customer needs are.
When we did this change at Marktplaats, people understood instantly that the data should be leading when we make decisions. We were an organization where a lot of gut feeling decision-making was done. We then shifted to data-driven decision-making and instantly saw acceleration in our performance.
Arje Sanders: I like that move from gut feeling-based decision-making to data-driven decision-making. Can you think of a moment when you thought that this is really a decision made based on data, and it's really different than what was done before?
Allard de Boer: One of the concepts we introduced was holistic testing. Any problem we solve on Marktplaats is like a Rubik's Cube. You solve one side, but then all the other sides get messed up. For example we introduced a new advertising position on our platform, and it performed really well on revenue. However, the customers really hated it. When only looking at revenue, we thought this is going well. However, if you looked at how customers liked it, you saw that over time the uptake would diminish because customer satisfaction would go down. This is an example of where we looked at performance from all angles and were able to scale this further.
After training people to know where to find the data, what the KPIs mean, how to use them, what are good practices, what are bad practices, the consumption of the data really went up, and so did the quality of the decisions.
Allard de Boer
Creating a blueprint for AI Transformation
Our strategies elevate the performance of your AI applications from marginal or tactical results to unequivocal, transformational successes.
Discuss your needsArje Sanders: You mentioned that in this entire transformation to become more data-driven, you needed to do something about people's capabilities. How did you approach that?
Allard de Boer: We had great sponsorship from the top. We had a visionary leader in the leadership team. Because data is so important to us as a company, everybody from the receptionist to the CEO, needs to have at least a profound understanding of the data. Within specific areas, of course, we want to go deeper and much more specific. But everybody needs to have access to the data, understand what they're looking at, and understand how to interpret the data.
Because we have so many data points and so many KPIs, you could always find a KPI that would go up. If you only focus on that one but not look at the other KPIs, you would not have the full view of what is actually happening. After training people to know where to find the data, what the KPIs mean, how to use them, what are good practices, what are bad practices, the consumption of the data really went up, and so did the quality of the decisions.
Arje Sanders: You said that you had support from the top. How important is that component?
Allard de Boer: For me, it’s vital. There's a lot of things you can do bottom up. This is where much of the innovation or the early starting of new ideas happen. However, when the leadership starts leaning in, that’s when things accelerate. Just to give you an example, I was responsible for implementing certain data governance topics a few years back. It took me months to get things on the roadmap, and up to a year to get things solved. Then we shifted the company to focus on impact and everybody had to measure their impact. Leadership started leaning in and I could get things solved within months or even weeks.
Arje Sanders: You've been collaborating with Rewire on the educational program. What do you like most about that collaboration, about this analytics university that we've developed together?
Allard de Boer: There are multiple things that really stand out for me. One is the quality of the people. The Rewire people are the best talent in the market. Also, when developing this in-house analytics academy, I was able to work with the best people within Rewire. It really helped to set up a high-quality program that instantly went well. It's also a company that oozes energy when you come in. When I go into your office, I'm always welcome. The drinks are always available at the end of the week. I think this also shows that it is a company of people working well together.
Arje Sanders: What I like a lot in those partnerships is that it comes from two sides. Not every partnership flourishes, but those that flourish, those are the ones that also open up to a real partnership. Once you have that, then you really see an accelerated collaboration. I like that a lot about that, working together.
Allard de Boer: Yes. Rewire is not just a vendor for us. You guys have been a partner for us from the start. I've always shared this as a collaborative effort. We could have never done this without Rewire. I think this is also why it's such a strong partnership over the many years.
For information about how to build Data & AI capabilities, visit GAIN - Rewire's Data & AI training unit.
About the authors
Allard de Boer is the Director of Global Data & Analytics at Adevinta, where he drives the scaling of data and analytics across a diverse portfolio of leading classifieds brands such as Marktplaats, Mobile.de, and Leboncoin. With over a decade of experience in data strategy, business transformation, and analytics leadership, Allard has held prominent roles, including Global Head of Analytics at eBay Classifieds Group. He is passionate about fostering data literacy, building scalable data solutions, and enabling data-driven decision-making to support business growth and innovation.
Arje Sanders is Partner at Rewire.
Joris Lindenhovius from Rabobank discusses the strategies, challenges, and successes in building data governance at the bank
In a recent interview, Joris Lindenhovius, Head of Data Management at Rabobank, and Rewire Principal Ties Carbo discuss Rabobank’s journey to establish robust data governance and foster a data-driven culture across its workforce, highlighting the technical and human elements essential for success. The discussion sheds light on the power of shared responsibility, strategic governance, and hands-on training to embed data as a core asset within a company.
Watch the full interview
The transcript below has been edited for clarity and length.
Ties Carbo: Joris, thanks for joining us on this interview. Can you please introduce yourself?
Joris Lindenhovius: I work at Rabobank and I'm the head of the data management department. I'm responsible for data management standards, having oversight of the implementation of those standards and making sure that our data is of the highest quality and always available and accessible to everyone within the bank.
We have data management as a strategic topic within Rabobank. So we report back to the managing board on its progress. Our vision is of taking stock of our most business critical reports and models, making sure that we implement our data management standards, and reporting to the managing board because it's a strategic topic – not only for us, but also for the supervisor. So scoping, focusing and monitoring our progress, because it's part of our vision.
Ties Carbo: Can you elaborate on a data-driven application or AI use case that had a significant impact?
Joris Lindenhovius: My team is a policy team. We draw up data management standards and we have the oversight bank-wide on the implementation but we're not responsible for the implementation itself. So if you look at success, it's about drawing up a master plan where you have a five-year commitment from the managing board, and a five-year budget to make that impact. So making sure that data is a strategic asset is one of our biggest successes.
Secondly, we have three levels of data governance: we have a data governance board at a strategic level, with participation of the managing board. We have a tactical committee. We also have an operational board that steers our data management program. And we are continuously refining and improving our governance. That can be seen as a success.
Data management is not only about technology, it's not only about plans, it's also about people.
Joris Lindenhovius
Data management is not only about technology, it's not only about plans, it's also about people. With the literacy team, the [GAIN-enabled] academy in place, my people deliver data training one day per week to the 42 thousand employees of the bank. We have a mandatory training, e-learning trainings. That's a huge success because it makes people aware of the fact that they're part of our data challenges.
Then we have a sponsor program that the managing board and the people under it must take to understand their position vis-à-vis our data challenges. This is also a big success. Finally, we just have to make sure that we keep on doing this and we keep on being successful in implementing our data management standards.
The biggest lesson is that you cannot do it alone. You have to share the responsibilities. Appoint data owners.
Joris Lindenhovius
Ties Carbo: What lessons could you share with others, whether banks or other companies?
Joris Lindenhovius: The biggest lesson is that you cannot do it alone. You have to share the responsibilities. Appoint data owners: make report owners or model owners responsible for the quality of the data, even though they have a limited amount of influence. But they have to know that they're responsible for it and they have to organize things. So sharing the ownership, sharing the responsibilities, I think is one of the biggest learnings. It's not just about training them, but it's also about putting them into the position – with the help of governance layers – to do their work. All of them are really busy and we're just adding new roles and responsibilities. So they have to understand what's in it for them. You teach that in the beginning and afterwards you hold them responsible for doing what they should be doing. You also help them by giving them insights, data and information to see whether we are moving in the right direction. But the biggest learning is sharing their responsibilities throughout the organization and putting people in a position to transform the organization into a data-driven organization.
Ties Carbo: How is it to work with Rewire? What stands out for you?
Joris Lindenhovius: I've been directly involved with Rewire at two companies, as part of creating a data-driven culture – or a data analytics-driven culture. I think the most important thing is that you take people away from their day-to-day job and have them focus during a full day or multi-day training on what is data, what is data analytics, what it means for you, and engage them through exercises to create that energy on this specific topic. Because before that it's mostly an opinion that they have because of the articles that they've read. By engaging them through a [data training] program you can tap into what it data is and what it means for them. That's what I was involved with at KPN and later at Rabobank – creating that culture using these training sessions. So taking employees out of their normal work – not an hour, not two hours – but for a whole day or two, put them through a program and letting them understand what data is and what it can be. That's what I think of when I think of Rewire.
About the authors
Joris Lindenhovius is an expert in data management and strategy, currently serving as Head of Global Data Management at Rabobank. With over 20 years in the field, Joris has held key leadership roles across sectors, including as Chief Data Officer at KPN, where he led the transformation of KPN’s data program and centralized data organization. He’s known for his deep expertise in data governance, data quality, and building data-driven cultures within organizations.
Ties Carbo is Principal at Rewire.