It takes a few principles to turn data into a valuable, trustworthy, and scalable asset.
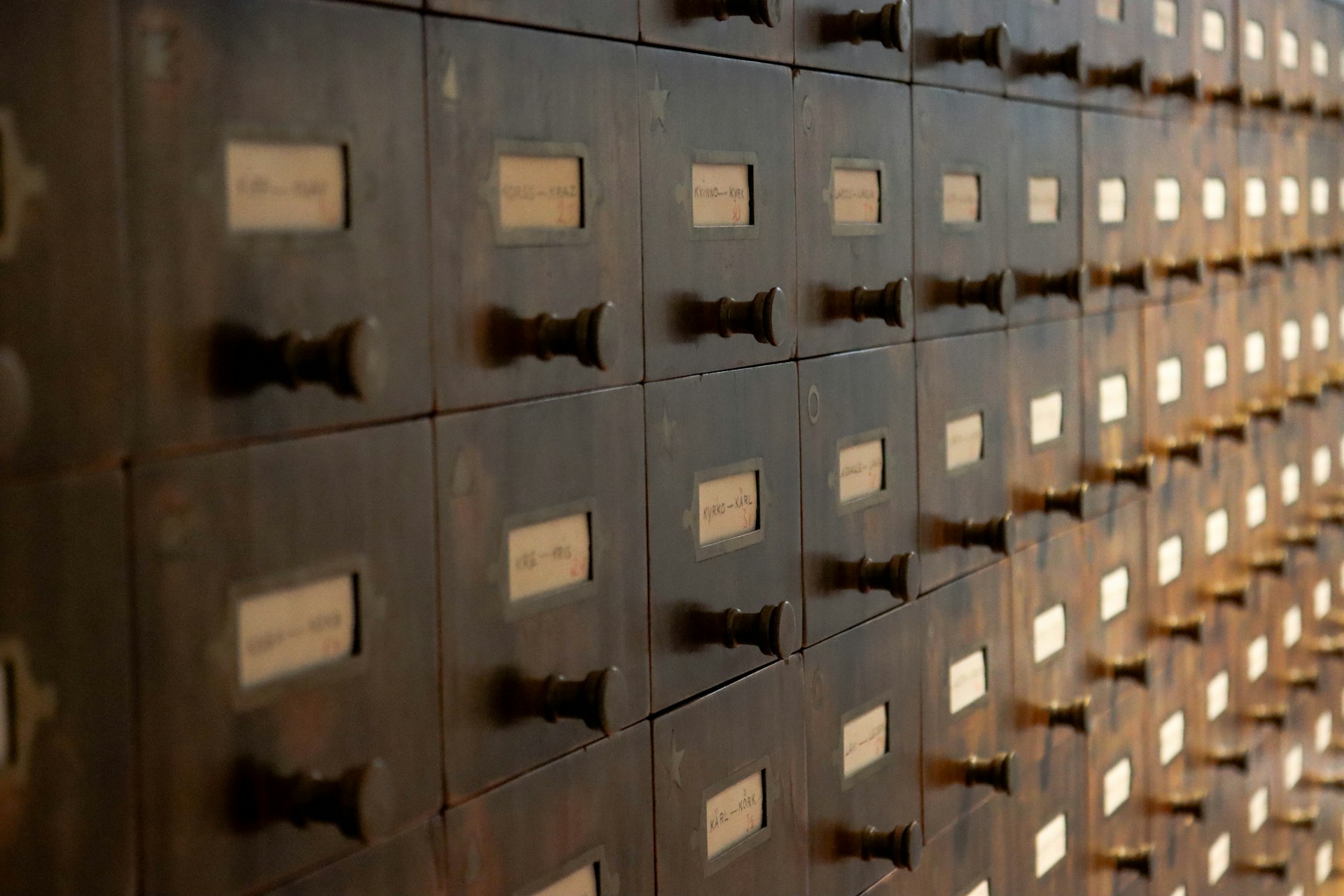
Imagine a runner, Amelia, who finishes her run every morning eager to pick up a nutritious drink from her favorite smoothie bar, "Running Smoothie”, at the corner of the street. But there’s a problem: Running Smoothie has no menu, no information about ingredients or allergens, and no standards for cleanliness or freshness. Even worse, some drinks should be restricted based on age — like a post-run mimosa — but there is no way to identify the drinks for adults only. For long time customers like Amelia, who know all products by heart, this isn’t much of an issue. But new customers, like Bessie, find the experience confusing and unpleasant, often deciding not to return.
Sounds strange, right? Yet, this is exactly how many organizations treat their data.
This scenario parallels the typical struggles organizations face in data management. Data pipelines can successfully transfer information from one system to another, but this alone doesn’t make the data findable, usable, or reliable for decision-making. Take a Sales team processing orders, for instance. That same data could be highly valuable for Finance, but it won’t deliver any value if the Finance team isn’t even aware the pipeline exists. This highlights a broader issue: simply moving data from point A to B falls short of a successful data exchange strategy.
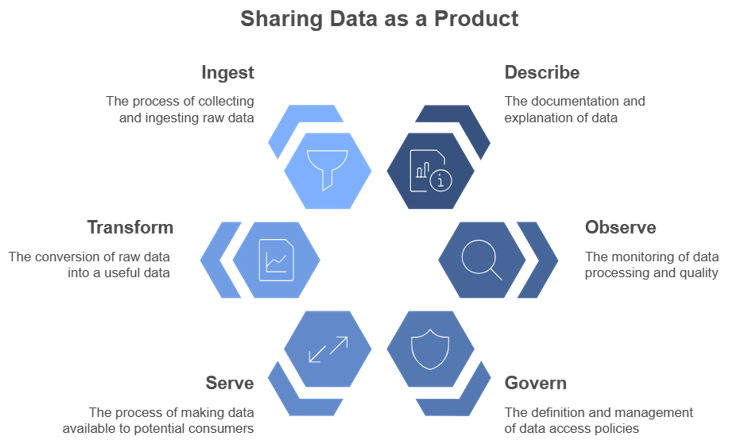
Effectively sharing Data as a Product requires sharing described, observable and governed data to ensure a smooth and scalable data exchange.
In response to this, leading organizations are embracing data as a product — a shift in mindset from viewing data as an output, to treating data as a strategic asset for value creation. Transforming data into a strategic asset requires attention to three core principles:
- Describe: ensure users can quickly find and understand the data they need, just as a well-labeled menu listing ingredients and allergens helps Bessie know what she can order.
- Observe: share data quality and reliability over time, both expected standards and unexpected deviations — like information on produce freshness and flagging when customers must wait longer than usual for their drink.
- Govern: manage who can access specific data so only authorized individuals can interact with sensitive information, similar to restricting alcoholic menu items based on an age threshold.
By embedding these foundational principles, data is not just accessible but is transformed into a dependable asset to create value organization-wide. This involves carefully designing data products with transparency and usability in mind, much like one would expect from a reputable restaurant's menu.
In this blog, we will explore why each principle is essential for an effective data exchange.
Describe: ensure data is discoverable and well-described
For data to create value, consumers need to be able to find, understand, and use it. If your team produces a dataset that’s crucial to multiple departments but remains tucked away on a platform no one knows about or is not well described and remains ambiguous, your crucial dataset might as well be invisible to potential consumers.
Findable data requires a systematic approach to metadata: think of it as the digital “menu listing” of data that helps others locate and understand it. Key metadata elements include the data schema, ownership details, data models, and business definitions. By embedding these in, for example, a data catalog, data producers help their consumers not only discover data but also interpret it accurately for their specific needs.
Observe: monitor data quality and performance
The next step is to share data quality—consumers need to know that what they are accessing is reliable. Data lacking quality standards leave users guessing whether the data is recent, consistent, and complete. Without transparency, consumers might hesitate to rely on data or worse, make flawed decisions based on outdated or erroneous information.
By defining and sharing clear standards around data quality and availability —such as timeliness, completeness, and accuracy— you enable consumers to determine if the data meets their needs. Providing observability into performance metrics, such as publishing data update frequency or tracking issues over time, allows users to trust the data and promotes data quality accountability.
Govern: manage data access and security
Finally, a successful data product strategy is built on well-managed data access. While data should ideally be accessible to any team or individual who can create value from it, data sensitivity and compliance requirements must be taken into account. Yet, locking all data behind rigid policies slows down collaboration and might lead teams to take risky shortcuts.
A well-considered access policy strikes the right balance between accessibility and security. This involves categorizing data access levels based on potential use cases, and establishing clear guidelines on who can view, modify, or distribute data. Effectively managed access not only safeguards sensitive information but also builds trust among data producers, who can rest assured their data is treated confidentially. Meanwhile, consumers can access and use data confidently, without friction or fear of misuse.
Sounds easy, but the devil is in the details
For many, these foundational principles may seem straightforward. Yet, we often see companies fall into the trap of relying solely on technology to solve their Data & AI challenges, neglecting to apply these principles holistically. This tech-first approach often results in poor adoption and missed opportunities due to a lack of focus on organizational context and value delivery.
Take data catalogs, for example — essential tools for data discoverability. While it may seem like a simple matter of choosing the right tool, driving real change requires a comprehensive approach that incorporates best practices from the Playbook to Scalable Data Management. Without them, companies face long-term risks where:
- Due to a lack of standards the catalog features data duplication, inconsistent definitions, no clear or recursively looping data lineage, and so on. For consumers of the data, this makes it difficult to navigate eroding its usefulness.
- Due to a lack of requirements the catalog is helpful for some teams, but useless for others, inviting proliferation of alternative tools further complicating data access and reducing overall adoption.
This illustrates the fact that something as fundamental as a data catalog isn’t just a technological fix. Instead, it requires a coordinated, cross-functional effort that aligns with business priorities and data strategy: it is not about implementing the right tool, but about implementing the tool the right way.
Conclusion: Data as a Product, not just Data
In the end, successfully sharing data across an organization is about more than just setting up access points and handing over datasets. It demands a holistic approach to data discoverability, observability, and governance suited to your organization. By embedding these principles, organizations can overcome common pitfalls in data sharing and set up a robust foundation that turns data into a true organizational asset. It’s not only a strategic shift in data management but also a cultural one that lays the foundation for scalable, data-driven growth.
This article was written by Femke van Engen, Data Scientist, Simon Beets, Data & AI engineer, and Freek Gulden, Lead Data Engineer at Rewire.