Home
Data & AI strategy
It takes a few principles to turn data into a valuable, trustworthy, and scalable asset.
Imagine a runner, Amelia, who finishes her run every morning eager to pick up a nutritious drink from her favorite smoothie bar, "Running Smoothie”, at the corner of the street. But there’s a problem: Running Smoothie has no menu, no information about ingredients or allergens, and no standards for cleanliness or freshness. Even worse, some drinks should be restricted based on age — like a post-run mimosa — but there is no way to identify the drinks for adults only. For long time customers like Amelia, who know all products by heart, this isn’t much of an issue. But new customers, like Bessie, find the experience confusing and unpleasant, often deciding not to return.
Sounds strange, right? Yet, this is exactly how many organizations treat their data.
This scenario parallels the typical struggles organizations face in data management. Data pipelines can successfully transfer information from one system to another, but this alone doesn’t make the data findable, usable, or reliable for decision-making. Take a Sales team processing orders, for instance. That same data could be highly valuable for Finance, but it won’t deliver any value if the Finance team isn’t even aware the pipeline exists. This highlights a broader issue: simply moving data from point A to B falls short of a successful data exchange strategy.
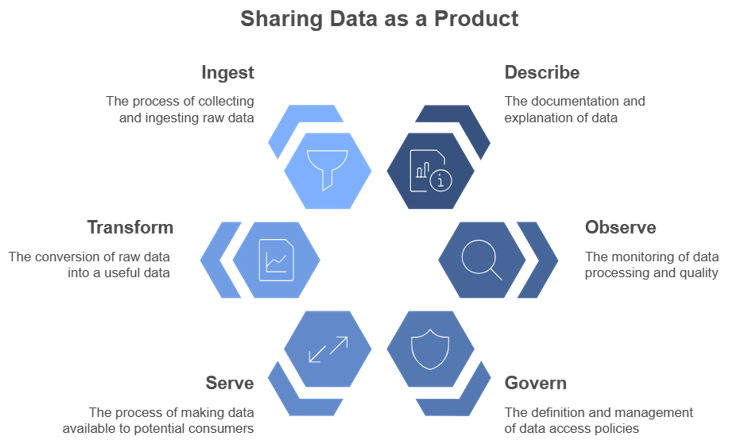
Effectively sharing Data as a Product requires sharing described, observable and governed data to ensure a smooth and scalable data exchange.
In response to this, leading organizations are embracing data as a product — a shift in mindset from viewing data as an output, to treating data as a strategic asset for value creation. Transforming data into a strategic asset requires attention to three core principles:
- Describe: ensure users can quickly find and understand the data they need, just as a well-labeled menu listing ingredients and allergens helps Bessie know what she can order.
- Observe: share data quality and reliability over time, both expected standards and unexpected deviations — like information on produce freshness and flagging when customers must wait longer than usual for their drink.
- Govern: manage who can access specific data so only authorized individuals can interact with sensitive information, similar to restricting alcoholic menu items based on an age threshold.
By embedding these foundational principles, data is not just accessible but is transformed into a dependable asset to create value organization-wide. This involves carefully designing data products with transparency and usability in mind, much like one would expect from a reputable restaurant's menu.
In this blog, we will explore why each principle is essential for an effective data exchange.
Describe: ensure data is discoverable and well-described
For data to create value, consumers need to be able to find, understand, and use it. If your team produces a dataset that’s crucial to multiple departments but remains tucked away on a platform no one knows about or is not well described and remains ambiguous, your crucial dataset might as well be invisible to potential consumers.
Findable data requires a systematic approach to metadata: think of it as the digital “menu listing” of data that helps others locate and understand it. Key metadata elements include the data schema, ownership details, data models, and business definitions. By embedding these in, for example, a data catalog, data producers help their consumers not only discover data but also interpret it accurately for their specific needs.
Observe: monitor data quality and performance
The next step is to share data quality—consumers need to know that what they are accessing is reliable. Data lacking quality standards leave users guessing whether the data is recent, consistent, and complete. Without transparency, consumers might hesitate to rely on data or worse, make flawed decisions based on outdated or erroneous information.
By defining and sharing clear standards around data quality and availability —such as timeliness, completeness, and accuracy— you enable consumers to determine if the data meets their needs. Providing observability into performance metrics, such as publishing data update frequency or tracking issues over time, allows users to trust the data and promotes data quality accountability.
Govern: manage data access and security
Finally, a successful data product strategy is built on well-managed data access. While data should ideally be accessible to any team or individual who can create value from it, data sensitivity and compliance requirements must be taken into account. Yet, locking all data behind rigid policies slows down collaboration and might lead teams to take risky shortcuts.
A well-considered access policy strikes the right balance between accessibility and security. This involves categorizing data access levels based on potential use cases, and establishing clear guidelines on who can view, modify, or distribute data. Effectively managed access not only safeguards sensitive information but also builds trust among data producers, who can rest assured their data is treated confidentially. Meanwhile, consumers can access and use data confidently, without friction or fear of misuse.
Sounds easy, but the devil is in the details
For many, these foundational principles may seem straightforward. Yet, we often see companies fall into the trap of relying solely on technology to solve their Data & AI challenges, neglecting to apply these principles holistically. This tech-first approach often results in poor adoption and missed opportunities due to a lack of focus on organizational context and value delivery.
Take data catalogs, for example — essential tools for data discoverability. While it may seem like a simple matter of choosing the right tool, driving real change requires a comprehensive approach that incorporates best practices from the Playbook to Scalable Data Management. Without them, companies face long-term risks where:
- Due to a lack of standards the catalog features data duplication, inconsistent definitions, no clear or recursively looping data lineage, and so on. For consumers of the data, this makes it difficult to navigate eroding its usefulness.
- Due to a lack of requirements the catalog is helpful for some teams, but useless for others, inviting proliferation of alternative tools further complicating data access and reducing overall adoption.
This illustrates the fact that something as fundamental as a data catalog isn’t just a technological fix. Instead, it requires a coordinated, cross-functional effort that aligns with business priorities and data strategy: it is not about implementing the right tool, but about implementing the tool the right way.
Conclusion: Data as a Product, not just Data
In the end, successfully sharing data across an organization is about more than just setting up access points and handing over datasets. It demands a holistic approach to data discoverability, observability, and governance suited to your organization. By embedding these principles, organizations can overcome common pitfalls in data sharing and set up a robust foundation that turns data into a true organizational asset. It’s not only a strategic shift in data management but also a cultural one that lays the foundation for scalable, data-driven growth.
This article was written by Femke van Engen, Data Scientist, Simon Beets, Data & AI engineer, and Freek Gulden, Lead Data Engineer at Rewire.
Jonathan Dijkslag on growing and maintaining the company's edge in biotechnology and life sciences
In the latest episode of our podcast, Jonathan Dijkslag, Global Manager of Data Insights & Data Innovation at Enza Zaden, one of the world’s leading vegetable breeding companies sits down with Ties Carbo, Principal at Rewire. With over 2,000 employees and operations in 30 countries, Enza Zaden has built its success on innovation, from pioneering biotechnology in the 1980s to embracing the transformative power of Data & AI today.
Jonathan shares insights into the company’s data-driven journey, the challenges of integrating traditional expertise with modern technology, and how cultivating a culture of trust, speed, and adaptability is shaping Enza Zaden’s future. Tune in to discover how this Dutch leader is using data to revolutionize vegetable breeding and stay ahead in a competitive, innovation-driven industry.
Watch the full interview
The transcript below has been edited for clarity and length.
Ties Carbo: Thank you very much for joining this interview. Can you please introduce yourself?
Jonathan Dijkslag: I work at Enza Zaden as the global manager of Data Insights and Innovation. My mission is to make Enza Zaden the most powerful vegetable-breeding company using data.
"It's about how to bring new skills, new knowledge to people, and combine it all together to deliver real impact."
Jonathan Dijkslag
Ties Carbo: Can you tell us a little bit about your experience on working with Data & AI?
Jonathan Dijkslag: What made Enza very successful is its breeding expertise. We are working in a product-driven market and we are really good at it. Enza grew over time thanks to the expertise of our people. In the 80s, biotech came with it. And I think we were quite successful in adapting to new technologies.
Now we are running into an era where data is crucial. So I think that data is at the heart of our journey. We are a company that values our expertise. But also we are really aware of the fact that expertise comes with knowledge, and with having the right people able to leverage that knowledge in such a way that you make impact. And that's also how we look at this journey. So it's about how to bring new skills, new knowledge to people, and combine it all together to deliver real impact.
Ties Carbo: It sounds like you're in a new step of a broader journey that started decades ago. And now, of course, Data & AI is a bit more prevalent. Can you tell us a little bit more about some successes that you're having on the Data & AI front?
Jonathan Dijkslag: There are so many, but I think the biggest success of Enza is that with everything we do, we are a conscious of our choices. Over the past three to five years, we have spent quite some money on the foundation for success. So where some companies are quite stressed out because of legacy systems, we do very well in starting up the right initiatives to create solid foundations and then take the time to finalize them. And I think that is very powerful within Enza. So over the past years we have built new skills based on scalable platforms in such a way that we can bring real concrete value in almost all fields of expertise in the company.
Ties Carbo: Can you maybe give a few examples of some challenges you encountered along the way?
Jonathan Dijkslag: I think the biggest challenge is that the devil is in the details. That's not only from a data journey perspective, but also in our [functional] expertise. So the real success of Enza with data depends on really high, mature [functional] expertise combined with very high, mature expertise in data. And bridging functional experts with data experts is the biggest challenge. It has always been the biggest challenge. But for a company like Enza, it's particularly complex.
Another complexity is we have quite some people who for decades have used data heavily in their daily work. Yet the way we use data nowadays is different than compared to decades ago. So the challenge is how to value the contributions made today and at the same time challenge people to take the next step with all the opportunities we see nowadays.
"We learned the hard way that if you don't understand – or don't want to understand what our purpose is as a company, then you will probably not be successful."
Jonathan Dijkslag
Ties Carbo: I was a bit intrigued by your first response – bridging the functional expertise with the Data & AI expertise. What's your secret to doing that?
Jonathan Dijkslag: I think it really comes back to understanding the culture of your company. When I joined the company, I knew that it would take time to understand how things work today. I needed to show my respect to everything that has brought so much success to the company in order to add something to the formula.
Every company has a different culture. Within Enza, the culture is pretty much about being Dutch. Being very direct, clear, result-driven, don't complain. Just show that you have something to add. And that's what we try to do. And learn, learn and adapt. For instance, in the war on talent, we made some mistakes. We thought maybe business expertise is not always that important. But we learned the hard way that if you don't understand – or don't want to understand what our purpose is as a company, then you will probably not be successful. And that means something to the pace and the people you can attract to the company. So it’s all about understanding the existing culture, and acting on it. And sometimes that means that you need more time than you’d like to have the right people or have the right projects finalized and create the impact you want.
"We have to create a mechanism whereby we're not too busy. We have to create a change model where you can again and again adapt to new opportunities."
Jonathan Dijkslag
Ties Carbo: What advice would you give to other companies that embark on a journey like this?
Jonathan Dijkslag: This is the new normal. The complexity is the new normal. So we have to think about how we can bring every day, every week, every month, every year more and more change. And people tend to say “I'm busy, we’re busy, wait, we have to prioritize.” I think we have to rethink that model. We have to create a mechanism whereby we're not too busy. We have to create a change model where you can again and again adapt to new opportunities. And I think this is all about creating great examples. So sometimes it’s better to make fast decisions that afterwards you would rate with a seven or an eight, but you did it fast. You were very clear and you made sure that the teams work with this decision that’s rated seven, rather than thinking for a long period about the best business case or the best ROI. So I think the speed of decision-making close to the area impact. I think that that's the secret.
People talk a lot about agility. I think for me, the most important part of agility is the autonomy to operate. Combined with very focused teams and super fast decision-making and the obligation to show what you did.
Ties Carbo: How is it to work with Rewire?
Jonathan Dijkslag: For me, it's very important to work with people who are committed. I have a high level of responsibility. I like to have some autonomy as well. And to balance those things, I think it's very important to be result-driven and also to show commitment in everything you do. And what we try to do in our collaboration with Rewire is to create a commitment to results. Not only on paper, but instead both parties taking ownership of success. And we measure it concretely. That's the magic. We're really in it together and there's equality in the partnership. That's how it feels for me. So I think that that's the difference.
We work a lot with genetic data. So our challenges are very specific. Life science brings some complexity with it. And we were looking for a partner who can to help us develop the maturity to understand and work with confidence with the corresponding data. And thanks to their experience in the field of life science, they showed that they understand this as well. And that's very important because trust is not easily created. And if you show that you brought some successes in the world of life science - including genetic data - that helps to get the trust, and step into realistic cases.
Ties Carbo: Thank you.
About the authors
Jonathan Dijkslag is the Global Manager of Data Insights & Data Innovation at Enza Zaden, where he drives impactful data strategies and innovation in one of the world's leading vegetable breeding companies. With over 15 years of experience spanning data-driven transformation, business insights, and organizational change, Jon has held leadership roles at Pon Automotive, where he spearheaded transitions to data-driven decision-making and centralized business analytics functions. Passionate about aligning technology, culture, and strategic goals, Jon is dedicated to creating tangible business impact through data.
Ties Carbo is Principal at Rewire.
Allard de Boer on scaling data literacy, overcoming challenges, and building strong partnerships at Adevinta - owner of Marktplaats, Leboncoin, Infojobs and more.
In this podcast, Allard de Boer, Director of Analytics at Adevinta (a leading online classifieds which includes brands like Marktplaats, Leboncoin, mobile.de, and many more) sits down with Rewire Partner Arje Sanders to explore how the company transformed its decision-making process from intuition-based to data-driven. Allard shares insights on the challenges of scaling data and analytics across Adevinta’s diverse portfolio of brands.
Watch the full interview
The transcript below has been edited for clarity and length.
Arje Sanders: Can you please introduce yourself and tell us something about your role?
Allard de Boer: I am the director of analytics at Adevinta. Adevinta is a holding company that owns multiple classifieds brands like Marktplaats in the Netherlands, Mobile.de in Germany, Leboncoin in France, etc. My role is to scale the data and analytics across the different portfolio businesses.
Arje Sanders: How did you first hear about Rewire?
Allard de Boer: I got introduced to Rewire [then called MIcompany] six, seven years ago. We started working on the Analytics academy for Marktplaats and have worked together since.
We were talking about things like statistical significance, but actually only a few people knew what that actually meant. So we saw that there are limits to our capabilities within the organization.
Allard de Boer
Arje Sanders: Can you tell me a little about the challenges that you were facing and wanted to resolve in that collaboration? What were your team challenges?
Allard de Boer: Marktplaats is a technology company. We have enormous technology, we have a lot of data. Every solution that employees want to create has a foundation in technology. We had a lot of questions around data and analytics and every time people threw more technology at it. That ran into limitations because the people operating the technology were scarce. So we needed to scale in a different way.
What we needed to do is get more people involved in our data and analytics efforts and make sure that it was a foundational capability throughout the organization. This is when we started thinking about how to scale specific use cases further. See how we can take what we have, but then make it common throughout the whole organization and then scale specific use cases further.
For example, one problem is that we did a lot of A/B testing and experimentation. Any new feature on Marktplaats was tested through A/B testing. It was evaluated on how it performed on customer journeys, and how it performed on company revenue. This was so successful that we wanted to do more experiments. But then we ran into the limitations of how many people can look at the experimentation, and how many people understand what they're actually looking at.
We were talking about things like statistical significance, but actually only a few people knew what that actually meant. So we saw that there are limits to our capabilities within the organization. This is where we started looking for a partner that can help us to scale employee education to raise the level of literacy within our organization. That's how we came up with Rewire.
We were an organization where a lot of gut feeling decision-making was done. We then shifted to data-driven decision-making and instantly saw acceleration in our performance.
Allard de Boer
Arje Sanders: That sounds quite complex because I assume you're talking about different groups of people with different capability levels, but also over different countries. How did you approach the challenge?
Allard de Boer: Growth came very naturally to us because we had a very good model. Marktplaats was growing very quickly in the Netherlands. Then after a while, growth started to flatten out a bit. We needed to rethink how we run the organization. We moved towards customer journeys and understanding customer needs.
Understanding those needs is difficult because we're a virtual company. Seeing what customers do, understanding what they need is something we need to track digitally. This is why the data and analytics is so vital for us as a company. If you have a physical store, you can see how people move around. If your store is only online, the data and analytics is your only corridor to understanding what the customer needs are.
When we did this change at Marktplaats, people understood instantly that the data should be leading when we make decisions. We were an organization where a lot of gut feeling decision-making was done. We then shifted to data-driven decision-making and instantly saw acceleration in our performance.
Arje Sanders: I like that move from gut feeling-based decision-making to data-driven decision-making. Can you think of a moment when you thought that this is really a decision made based on data, and it's really different than what was done before?
Allard de Boer: One of the concepts we introduced was holistic testing. Any problem we solve on Marktplaats is like a Rubik's Cube. You solve one side, but then all the other sides get messed up. For example we introduced a new advertising position on our platform, and it performed really well on revenue. However, the customers really hated it. When only looking at revenue, we thought this is going well. However, if you looked at how customers liked it, you saw that over time the uptake would diminish because customer satisfaction would go down. This is an example of where we looked at performance from all angles and were able to scale this further.
After training people to know where to find the data, what the KPIs mean, how to use them, what are good practices, what are bad practices, the consumption of the data really went up, and so did the quality of the decisions.
Allard de Boer
Arje Sanders: You mentioned that in this entire transformation to become more data-driven, you needed to do something about people's capabilities. How did you approach that?
Allard de Boer: We had great sponsorship from the top. We had a visionary leader in the leadership team. Because data is so important to us as a company, everybody from the receptionist to the CEO, needs to have at least a profound understanding of the data. Within specific areas, of course, we want to go deeper and much more specific. But everybody needs to have access to the data, understand what they're looking at, and understand how to interpret the data.
Because we have so many data points and so many KPIs, you could always find a KPI that would go up. If you only focus on that one but not look at the other KPIs, you would not have the full view of what is actually happening. After training people to know where to find the data, what the KPIs mean, how to use them, what are good practices, what are bad practices, the consumption of the data really went up, and so did the quality of the decisions.
Arje Sanders: You said that you had support from the top. How important is that component?
Allard de Boer: For me, it’s vital. There's a lot of things you can do bottom up. This is where much of the innovation or the early starting of new ideas happen. However, when the leadership starts leaning in, that’s when things accelerate. Just to give you an example, I was responsible for implementing certain data governance topics a few years back. It took me months to get things on the roadmap, and up to a year to get things solved. Then we shifted the company to focus on impact and everybody had to measure their impact. Leadership started leaning in and I could get things solved within months or even weeks.
Arje Sanders: You've been collaborating with Rewire on the educational program. What do you like most about that collaboration, about this analytics university that we've developed together?
Allard de Boer: There are multiple things that really stand out for me. One is the quality of the people. The Rewire people are the best talent in the market. Also, when developing this in-house analytics academy, I was able to work with the best people within Rewire. It really helped to set up a high-quality program that instantly went well. It's also a company that oozes energy when you come in. When I go into your office, I'm always welcome. The drinks are always available at the end of the week. I think this also shows that it is a company of people working well together.
Arje Sanders: What I like a lot in those partnerships is that it comes from two sides. Not every partnership flourishes, but those that flourish, those are the ones that also open up to a real partnership. Once you have that, then you really see an accelerated collaboration. I like that a lot about that, working together.
Allard de Boer: Yes. Rewire is not just a vendor for us. You guys have been a partner for us from the start. I've always shared this as a collaborative effort. We could have never done this without Rewire. I think this is also why it's such a strong partnership over the many years.
About the authors
Allard de Boer is the Director of Global Data & Analytics at Adevinta, where he drives the scaling of data and analytics across a diverse portfolio of leading classifieds brands such as Marktplaats, Mobile.de, and Leboncoin. With over a decade of experience in data strategy, business transformation, and analytics leadership, Allard has held prominent roles, including Global Head of Analytics at eBay Classifieds Group. He is passionate about fostering data literacy, building scalable data solutions, and enabling data-driven decision-making to support business growth and innovation.
Arje Sanders is Partner at Rewire.
From chess-playing robots to a future beyond our control: Prof Stefan Leijnen discusses the challenges of AI and its evolution
Stefan’s Leijnen experience spans cutting-edge AI research and public policy. As professor in applied AI, he focuses on machine learning, generative AI, and the emergence of artificial sentience. As the lead for EU affairs for the Dutch National Growth Fund project AiNed, Stefan plays a pivotal role in defining public policy that promotes innovation while protecting citizens’ rights.
In this captivating presentation, Stefan takes us on a journey through 250 years of artificial intelligence, from a chess-playing robot in 1770 to the modern complexities of machine learning. With thought-provoking anecdotes he draws parallels between the past and the ethical challenges we face today. As the lead in EU AI policy, Stefan unpacks how AI is reshaping industries, from Netflix’s algorithms to self-driving cars, and why we need to prepare for its profound societal impacts.
Below we summarize his insights as a series of lessons and share the full video presentation and its transcript.
Prepare to rethink what you know about AI and its future.
Lesson 1. AI behaviour is underpinned by models that cannot be understood by humans
10 years ago, Netflix asked the following question: “How do we know that the categories that we designed with our limited capacity as humans are the best predictors for your viewing preferences? We categorize people according to those labels, but those might not be the best labels. So let’s reverse engineer things: now that we have all this data, let’s decide what categories are the best predictors for our viewership.” And they did that. And so they come up with 50 dimensions or 50 labels, all generated by the computer, by AI. And 20 of them made a lot of sense: gender, age, etc. But for 30 of those 50 labels, you could not identify the category. That means that the machine uncovered a quality among people that we don’t have a word for. For Netflix this was great because it meant they now had 30 more predictors. But on the other hand, it’s a huge problem. Because now if you want to change something in those labels or you want to change something in the way that you use the model, you no longer understand what you’re dealing with.
Watch the video clip.
Lesson 2. AI’s versatility can lead to hidden – and very hard – ethical problems
Let’s say the camera of a self-driving car spots something and there’s a 99% chance that it is just a leaf blowing by, and a 1% chance that it’s a child crossing the street. Do you break? Of course, you would break in a 1% chance. But now let’s lower the chance to 0.1% or 0.01%. At what point do you decide to break?
The point, of course, is that we never make that decision as humans. But with rule-based programs, you have to make that decision. So it becomes an ethical problem. And these kind of ethical problems are much more difficult to solve than technological problems. Because who’s going to answer that? Who’s going to give you this number? It’s not the programmer. The programmer will go to the manager or to the CEO and they will go to the legal division or to the insurer or to the legislator. And nobody’s willing to provide an answer. For moral reasons (and for insurance reasons), it’s very difficult to solve this problem. Now, of course, nowadays there’s a different approach: just gather the data and calculate the probability of breaking that humans have. But in doing so, you have moved the ethical challenge under the rug. But it’s still there. So don’t get fooled by those strategies.
Watch the video clip.
Lesson 3. AI impact is unpredictable. But its impact won’t be just technological. It will be societal, economical, and likely political
There are other systems technologies like AI. We have the computer, we have the internet, we have the steam engine and electricity. And if you think about the steam engine, when it was first discovered, nobody had a clue of the implications of this technology 10, 20 or 30 years down the line. The first steam engines were used to automate factories. So instead of people working on benches close to each other, the whole workforce was designed along the axis of this steam engine so everything would be mechanically automated. This meant a lot of changes to the workforce. It meant that work could go for hours on end, even in the evenings and in the weekends. That led to a lot of societal changes. So labor forces emerged, you had unions, you had new ideologies popping up. The steam engine also became a lot smaller. You got the steam engine on railways. Railways meant completely different ways of warfare, economy, diplomacy. The world got a lot smaller. This all happened in the time span of several decades. We will see similar effects that are completely unpredictable as AI gets rolled out in the next couple of decades. Most of these effects of the steam engine were not technological. They were societal, economical, sometimes political. So it’s also good to be aware of this when it comes to AI.
Watch the video clip.
Lesson 4. The interfaces with AI will evolve in ways we do not yet anticipate.
The AI that we know now is very primitive. Because what we see today in AI is a very old interface. With ChatGPT, it’s a text command prompt. When the first car was invented, it was a horseless carriage. When the first TV was invented, it was essentially radio programming with an image glued on top of it. Now, for most of you who have been following the news, you already see that the interfaces are developing very rapidly. So you’ll get voice interfaces, you’ll get a lot more personalization with AI. This is a clear trend.
Watch the video clip.
Watch the full video.
(Full transcript below.)
Full transcript.
The transcript has been edited for brevity and clarity.
Prof Leijnen: Does anybody recognize this robot that I have behind me? Not many people. Well, that’s not surprising because it’s a very old robot. This robot was built in the year 1770, so over 250 years ago. And this robot can play chess.
And it was not just any chess-playing robot. It was actually an excellent chess-playing robot. He won most games. And as you can imagine at that time, it was a celebrity. This robot played against Benjamin Franklin. It played against emperors and kings. It also played a game against Napoleon Bonaparte. We know what happened because there were witnesses there. In fact, Napoleon being the smart man that he is, he decided to play a move in chess that’s not allowed just to see the robot’s reaction. What the robot did is it took the piece that Napoleon moved and put it back in its original position.
Napoleon being inventive, did the same illegal move again. Then the robot took the piece and put it beside the board as though it’s no longer in the game. Napoleon tried a third time and then the robot wiped all the pieces off the board and decided that the game was over, to the amusement of the spectators. Then they set up the pieces again, played another game and Napoleon lost. Well, to me, this is really intelligence.
You might think or not think that this is artificial intelligence. If you think this is not artificial, you’re right. Because there’s this little cabinet which has like magnets and strings and there was a very small person that could fit inside this cabinet and who was very good at playing chess. And of course, he is the person who played the game. People only found out about 80 years after the facts when the plans were revealed by the son of von Kempelen.
Now, there was another person who played against this robot and his name was Charles Babbage. And Charles Babbage is the inventor of this machine, the analytical engine. And it’s considered by many to be the first computer in the world. It was able to calculate logarithms. Interestingly, Babbage played against the robot that you just saw. He also lost in 18 turns. But I like to imagine that Babbage must have been thinking how does this robot work, what’s going on inside.
As some of you may know, a computer actually beat the world champion in chess, Gary Kasparov in 1997. So you could say in this story spanning 250 years is a nice story arc. Because now we do have AI that can play and win chess, which was the original point of the chess-playing robots. So we’re done. We’re actually done with AI. We have AI now. The future is here. But at the same time, we’re not done at all. Because now we have this AI and we don’t know how to use it. And we don’t know how to develop it further.
There is a nice example from Netflix, the streaming company. They collect a lot of data. They have your age, gender, postal code, maybe your income. They know things about you. And then based on those categories, they try to predict with machine learning what type of series and movies you like to watch. And this is essentially their business model. Now, 10 years ago they asked the following question: “How do we know that the categories that we designed with our limited capacity as humans are the best predictors for your viewing preferences? We categorize people according to those labels, but those might not be the best labels. So let’s reverse engineer this machine learning algorithm. And now that we have all this data, let’s decide what categories are the best predictors for your viewership.”
And they did that. And they came up with 50 dimensions or 50 labels that you could attach to a viewer, all generated by the computer, by AI. And 20 of them made a lot of sense. So you would see men here, women there. You would see an age distribution. And there was very clear preferences in viewership. Of course, not completely uniform, but you could identify the categories and you could attach a label to them.
Now for 30 of those 50 labels, you could not identify the category. For example, for one of the 30 categories, you would see people on the left side, people on the right side. On the left side, they had a strong preference for the movie “American Beauty.” And on the right side, they had a strong preference for X on the beach. And nobody had any clue what discerned the group on the left from the group on the right. So that means that there was a quality in those groups of people that we don’t have a word for. We don’t know how to understand that. Which for Netflix was great because it means they had now 30 more predictors they could use to do good predictions. But on the other hand, it’s a huge problem. Because now if you want to change something in those labels or you want to change something in the way that you use the model, you no longer understand what you’re dealing with.
And this is essentially the topic of what I am talking about today. How do you manage something you can’t comprehend? Because essentially that’s what AI is. And this is not just a problem for companies implementing AI. We all know plenty of examples of AI going wrong. And when it goes wrong, it tends to go wrong quite deeply. Like in this case, if you ask AI to provide you with an image of a salmon, the AI is not wrong. Statistically speaking, it is the most likely image of a salmon you’ll find on the internet. But of course we know that this is not what was expected. And this is not just a bug. It’s a feature of AI.
I teach AI, I teach students how to program AI systems and machine learning systems. If I ask my students to come up with a program, without using machine learning or AI, that filters out the dogs from the cakes in these kind of images. It will be very difficult because it’s very hard to come up with a rule set that can discern A from B. At the same time, we know that for AI, machine learning, this is a very easy task. And that’s because the AI programs itself. Or in other words, AI can come up with a model that is so complex that we don’t understand how it works anymore, but it still produces the outcome that we’re looking for. In this case, a category classifier.
And that’s great because those very complex models allow us to build systems of infinite complexity. There’s no boundary to the complexity of the model. Just the data that you use, the computing power that you use, the fitness function that you use, but those things we can collect. But it’s also terrible because we don’t know how to deal with this complexity anymore. It’s beyond our human comprehension.
Now, about 12, 13 years ago, I was in Mountain View at Google. They had a self-driving car division. The head of their self-driving car division explains the following problem to us. He said: “We have to deal with all kinds of technical challenges. But what do you think our most difficult challenge is?” Now, this was a room full of engineers. So they said, “Well, the steering or locating yourself on the street or how do I do image segmentation?” He said, “No, you’re all wrong. Those are all technical problems that can be solved. There’s a much deeper underlying problem here. And that’s the problem of when do I break?”
Let’s say the camera spots something and there’s a 99% chance that it’s a leaf blowing by. And it’s a 1% chance that it’s a child crossing the street. Do you break? Well, of course, you would break in a 1% chance. But now we lower the chance to 0.1 or 0.01. At what point do you decide to break? The point, of course, is that we never make that decision as humans. But when you program a system like that, you have to make a decision because it’s rule-based. So you have to say if the probability is below this and that, then I break. So it becomes an ethical problem. And these kinds of ethical problems are much more difficult to solve than technological problems. Because who’s going to answer that? Who’s going to give you this number? It’s not the programmer. The programmer will go to the manager or to the CEO and they will go to the legal division or to the insurer or to the legislator. And nobody’s willing to provide an answer. For moral reasons, also for insurance reasons, it’s very difficult to solve this problem, he said. Now, nowadays they have a different approach. They just gather the data and they say based on this data, this is the probability of breaking that humans have. And so they moved the ethical challenge under the rug. But it’s still there. Don’t get fooled by those strategies.
The examples I showed, of Netflix and Google, are from tech companies. But you see it everywhere. We also know that AI is going to play a major role in healthcare in the future. Not just in medicine, but also in caring for the elderly, for monitoring, for prevention, etc. This raises lots of ethical questions. Is this desirable? Here we see a woman who needs care. There’s no care for her. This is from the documentary “Still Alice”. And there’s this robot companion taking care of her, mental care. Is this what we want or is this not what we want? Again, it’s not a technical question. It’s a moral question.
In the last 10, 15 years and in the foreseeable future, AI has moved from the lab to society. ChatGPT is adopted at a much higher rate than most companies know. A large percentage of employees use ChatGPT. But if you ask company CEOs, they probably mention a lower number because many employees use these kind of tools without the company knowing it. We know that a majority of jobs in the Netherlands will be affected by AI, either by full of partial displacement, or AI complementing their work. And we also know that there are enormous opportunities in terms of automation. So on the one hand, it’s very difficult to manage such technology, not just its bugs, but its intrinsic properties. On the other hand, it provides enormous promises for problems that we don’t know how else to solve.
So it’s wise to take a step back and think more deeply and more long-term about the effects of this technology – before we start thinking about how to innovate and how to regulate the technology. What helps us is looking back a little bit. There are other systems technologies like AI. We have the computer, we have the internet, we have the steam engine and electricity. And if you think about the steam engine, when it was first discovered, nobody had a clue of the implications of this technology 10, 20 or 30 years down the line. The first steam engines were used to automate factories. Instead of people working on benches close to each other so they could talk, the whole workforce was designed along the axis of this steam engine so everything would be mechanically automated. This meant a lot of changes to the workforce. It meant that work could go on hour after hour, even in the evenings and in the weekends because now you have this machine, and you want to keep using it. That led to societal changes. You had labor forces, you had unions, you had new ideologies popping up. The steam engine also became a lot smaller. You got the steam engine on railways. Railways meant completely different ways of warfare, economy, diplomacy. The world got a lot smaller. This all happened in the time span of several decades but we will see similar effects that are completely unpredictable as AI gets rolled out in the next couple of decades. Most of these effects of the steam engine were not technological. They were societal, economical, sometimes political. So it’s also good to be aware of this when it comes to AI.
A second element of AI is the speed at which it develops. I’ve been giving talks about artificial creativity for about 10 years now and 10, 8 years ago, it was very easy for me to create a talk. I could just show people this image and then I would say, this cat does not exist and people would be taken aback. This was the highlight of my presentation. Now I show you this image and nobody raises an eyebrow. And then two years later, I had to show this image. Again, I see no reaction from you. I don’t expect any reaction, by the way. But it shows just how fast it goes and how quickly we adopt and get used to these kind of tools. And it also raises the question: given what was achieved in the past 8 years, where will we be in 25 years from now? You can, of course, apply AI in completely new different fields, similar as was done with the steam engine: creating new materials, coming up with new inventions, new types of engineering. We already know that AI has a major role to play in creativity and in coding.
We also know that the AI that we know now is very primitive. I’ll be giving another speech in 10 years and the audience won’t be taken aback by anything. Because what we see today is AI with a very old interface. At ChatGPT, it’s the interface of the internet [i.e. a text command prompt], of the previous system technology. And that’s always been the case. When the first car was invented, it was a horseless carriage. When the first TV was invented, it was essentially radio programming with an image added to it. And now what we have with AI is an internet browser with an AI model behind it. And for most of you who have been following the news, you see that the interfaces are developing very rapidly. You’ll get voice interfaces, you’ll get a lot more personalization with AI. This is also a trend that’s very clear.
So we talked a bit about the history, we talked a bit about the pace of development, about the complexity being a feature, not a bug. We can also dive a little bit more into the technology itself. This is a graph I designed five years ago with a student of mine. It’s called the Neural Network Zoo. And what you see is from the top left, all the way to the bottom right, is the evolution of neural network architectures. Interestingly, at the bottom right, this is called the transformer architecture. Essentially, the evolution stopped and most AI that you hear about nowadays, most AI developed at Microsoft and Google and OpenAI and others are based on this transformer architecture. So there was this Cambrian explosion of architectures, and then suddenly it converged.
Until five years or so AI models were proliferating. Now they’re also converging. Nowadays, we talk about OpenAI’s GPT, we talk about Google’s Gemini, we talk about Meta’s LAMA, Mistral. There aren’t that many models. So not just the technology has been locking in, but the models themselves as well. So you see huge conversions into only a very limited set of players and models. And this is of course due to the scaling laws. It becomes very difficult to play in this game. But it’s very interesting that on the one hand you have a convergence to a limited set of models in a limited set of companies. And on the other hand, you have this emergence of new functionalities coming out of these large scale models. So they surprise us all the time, but they’re only a very limited set of models that are able to surprise us. And these developments, these trends, all inform the way that we regulate this technology.
This is currently how the European Union thinks about regulating this technology. You have four categories. (1) A minimal risk category where there’s not much or hardly any legislation. (2) A limited risk. For example, if I interact with a chatbot, I have to know I’m interacting with a chatbot and not a human. The AI has to be transparent. (3) A high risk category, where there will be all kinds of ethical checks around, let’s say toys or healthcare or anything that has a real risk for consumers or citizens or society. (4) Unacceptable risk, which is AI systems that can subconsciously influence you, do social scoring, etc. Those will all be forbidden under new legislation (the EU AI Act).
I’ll end the presentation with this final quote, because I think this is essentially where we are right now: “The real problem of humanity is the following: we have paleolithic emotions, medieval institutions and with AI, god-like technology.” (E.O. Wilson).
The 2024 Nobel Prizes in Physics and Chemistry put the spotlight on AI. While the Physics laureates, John Hopfield and Geoffrey Hinton, contributed to its theoretical foundations, two of the three Chemistry laureates – specifically, Demis Hassabis and John Jumper – were rewarded for putting it into use.
John Hopfield developed the Hopfield network in 1982, a form of recurrent artificial neural network that can store and retrieve patterns, mimicking how human memory works. It operates by processing and recognizing patterns even when presented with incomplete or distorted data. His work was significant because it helped bridge the gap between biology and computer science, showing how computational systems could simulate the way the human brain stores and retrieves information.
Geoffrey Hinton co-invented the Boltzmann Machines, a type of neural network that played an important role in understanding how networks can be trained to discover patterns in data. He also popularized the use of backpropagation, an algorithm for training multi-layer neural networks, which considerably improved their capacity to learn complex patterns. Hinton’s contributions ultimately led to AI systems like GPT (Generative Pre-trained Transformers), which underpins ChatGPT, and AlphaFold the AI program that earned Demis Hassabis and John Jumper their Nobel prize in Chemistry.
AlphaFold solved one of biology’s greatest challenges: accurately predicting the 3D structure of proteins from their amino acid sequences. This problem had stumped scientists for decades, as protein folding is essential to understanding how proteins function, which is crucial for drug discovery, disease research, and biotechnology. AlphaFold’s predictions were so accurate that they matched experimental results with near-perfect precision, revolutionizing the field of biology. This breakthrough has wide-ranging implications for medicine and has already begun to accelerate research into diseases, drug discovery, and bioengineering.
Towards AI-driven disruption of traditional business models
Beyond the world of academia and frontier research, the AI techniques developed by the 2024 laureates are permeating the business world too. For one, the capabilities to analyse, identify patterns, and make sense of vast datasets, particularly unstructured data, rely at least partially on them.
From supply chain optimization to consumer behaviour analysis, AI holds the promise of making data-driven decisions faster, and automating a growing range of tasks. Large companies have already launched initiatives to capitalize on this, with some notable successes. Witness the case of a telecom company that generated an ROI 2.5x higher than average thanks to the judicious use of AI; or the case of an energy provider that delivered savings for consumers while increasing its own revenues; or this Supply Chain example that minimized waste and lost sales, while reducing the need for manual intervention at store level. These cases are no exceptions. Increasingly, the deployment of advanced algorithms and data management techniques play a central role in gaining competitive advantage.
Ultimately, AI ability to make sense of vast quantities of data will accelerate innovation and paves the way for new business models that will disrupt existing ones. From biotech to finance and manufacturing, the possibilities are endless, and all industries will eventually be impacted. More prosaically, the breakthroughs of the 2024 Nobel laureates herald an era when AI is not just a futuristic concept, but a key driver of competitiveness right now.
Technology and innovation expert Tom Goodwin on the merits of GenAI and how to leverage its potential.
During Rewire LIVE, we had the pleasure of hosting Tom Goodwin, a friend of Rewire and pragmatic futurist and transformation expert who advises Fortune 500 companies on emerging technologies such as GenAI. Over the past 20 years, he has studied the impact of new technology, new consumer behaviors and the changing rules of business, which makes him uniquely suited to understand the significance of GenAI today.
At the core of Tom’s thinking lies a question that all leaders should ponder: if, knowing everything you know now, were to build your company from scratch, what would it look like? At times counter-intuitive, Tom’s insights, steeped in history, provide valuable clues to answer this question. In this article, we share a handful of them.
INSIGHT 1: Technology revolution happens in two stages. In the first stage we add to what was done before. In the second stage we rethink. That’s when the revolution really happens.
Tom’s insight is derived from the Perez Framework, developed by Carlota Perez, a scholar specialized in technology and socio-economic development. The framework – based on the analysis of all the major technological revolutions since the industrial revolution – stipulates that technological revolutions first go through an installation phase, then a deployment stage. In the installation phase, the technology comes to market and the supporting infrastructure is built. In the deployment phase, society fully adopts the technology. (The transition between the two phases is typically marked by a financial crash and a recovery.)
During the first phase, there’s a frenzy – not dissimilar to the hype that currently surrounds GenAI. Everyone jumps on the technology, everyone talks about it. However, nothing profound really changes. For the most part, the technology only adds to the existing ways of doing things. In contrast, during the second stage, people finally make sense of the technology and use it to rethink the way things are done. That’s when the value is unleashed.
Take electricity as an example. In the first stage, electricity brought the electric iron, the light, the fan, the oven. These were all things that existed before. In the second stage, truly revolutionary innovations emerged: the radio, the TV, the telephone, the microwave, the microwave dinner, factories that operate 24/7, and so on. The second stage required a completely different mindset vis-à-vis what could do be done and how people would behave.
This begs the question: what will be the second stage of GenAI – and more broadly AI – be? What will be the telephone, radio, microwave for AI? Tom’s assertion here is that the degree of transformation is less about how exciting that technology is, and it’s much more about how deeply you change. Better AI will be about systems that are completely rethought and deep integrations, rather than UI patches.
Watch the video clip.
INSIGHT 2: Having category expertise, knowing how to make money, having relationships, and having staff who really know what they’re doing is probably more important than technology expertise.
Across many industries, battle lines are drawn between large traditional companies that have been around for a long time and the digitally-enabled, tech first, mobile-centric startup types. Think Airbnb vs Marriott, Tesla vs. BMW, SpaceX vs NASA, and so on.
The question is who’s going to win. Is it the digitally native companies who have created themselves for the modern era? Or is it the traditional companies that have been around for a long time? Put another way, is it easiest to be a tech company and learn how to make money in your target industry? Or be a big company who already knows how to make money but must now understand what a technology means and adapt accordingly?
Up until recently, the assumption was that the tech companies would win the battle. This proved true for a while: Netflix vs. Blockbusters, Apple vs. Nokia, etc. The assumption was that this would carry on. Understanding the technology was more important than understanding the category.
Tom’s observation is that in the past four years, these assumptions have been challenged. For example, traditional banks have got really good at understanding technology. Neobanks might be good at getting millennials to share the cost of a pizza, but they’re not that good at making money. So there’s this slow realisation that maybe digital-first tech companies are not going to win – because big companies are getting pretty good at change.
Taking a step back, it seems that the narrative of disrupt or die isn’t always true: a lot of the rules of business have not changed; incumbents just need to get a bit more excited about technology. Ultimately, having category expertise, knowing how to make money, having relationships, and having staff who really know what they’re doing is probably more important than tech expertise.
Watch the video clip.
INSIGHT 3: The AI craze is enabling a more flexible investment climate. This is an incentive for leaders to be bold.
Generative AI has spurn heated debates about the evolution of AI and divided experts and observers into two opposing groups: the AI cheerleaders and the sceptics. The former believe that AI is going to change everything immediately. The latter think that it’s a bubble.
History is littered with innovations that went nowhere. A handful of them however proved to be transformational – if in the long run. Only time will tell which group GenAI will join. In the meantime, there’s a growing realization that significant investment may be required to make meaningful steps with AI, hence a more flexible climate for capex – which is an incentive for leaders to be bold.
Tom’s insight reflects this situation: change is hard and expensive, and so regardless of one’s position in the debate, GenAI provides a unique window of opportunity to get the investor that you wouldn’t normally get. It is an amazing time to have an audience who normally wouldn’t listen to you.
Conclusion
These were but a handful of the many insights that Tom shared with us during Rewire LIVE. Taking a step back, it is clear that we are far from having realized the full value of GenAI – and, more broadly, AI. In the words of Tom, AI is a chance to dream really big and leave your mark on the world. It is yours for grab.
About Tom Goodwin
Tom Goodwin is the four time #1 in “Voice in Marketing” on LinkedIn with over 725,000 followers on the platform. He currently heads up “All We Have Is Now”, a digital business transformation consultancy, working with Clients as varied as Stellantis, Merck, Bayer, and EY to rethink how they use technology.
Tom hosts “The Edge” a TV series focusing on technology and innovation, and “My Wildest Prediction”, a podcast produced and distributed by Euronews. He has published the book “Digital Darwinism” with Kogan Page, and has spoken in over 100 cities across 45 countries.
With a 23 year career that spans creative, PR, digital and media agencies, Tom is an industry provocateur as a columnist for the Guardian, TechCrunch and Forbes and frequent contributor to GQ, The World Economic Forum, Ad Age, Wired, Ad Week, Inc, MediaPost and Digiday.
To find out more about Tom, visit www.tomgoodwin.co
Rewire CEO Wouter Huygen reviews the arguments for and against GenAI heralding the next industrial revolution, and how business leaders should prepare.
Is generative AI under- or overhyped? Is it all smoke and mirrors, or is it the beginning of a new industrial revolution? How should business leaders respond? Should they rush to adopt it or should they adopt a wait-and-see approach?
Finding clear-cut answers to these questions is a challenge for most. Experts in the field are equally divided between the cheerleaders and the skeptics, which adds to the apparent subjectivity of the debate.
The GenAI cheerleaders can point to the fact that performance benchmarks keep being beaten. Here the underlying assumption is the “AI Scaling Hypothesis”. That is, as long as we throw in more data and computing power, we’ll make progress. Moreover, the infrastructure required for GenAI at scale is already there: an abundance of cloud-based data and software; the ability to interact with the technology using natural language. Thus, innovation cycles have become shorter and faster.
On the other hand, GenAI skeptics make the following points: first, the limitations of GenAI are not bugs, they’re features. They’re inherent to the way the technology works. Second, GenAI lacks real world understanding. Third, LLMs demonstrate diminishing returns. In short, there are hard limits to the capabilities of GenAI.
The lessons of History indicate that while there might be some overhype around GenAI, the impact could be profound – in the long run. Leaders should therefore develop their own understanding of GenAI and use it to define their vision. Shaping the future is a long-term game that starts today.
Watch the video (full transcript below).
The transcript has been edited for clarity and length.
Generative AI: the new magic lantern?
Anyone recognizes this? If you look closely, not much has changed since. Because this is a basic slide projector. It’s the Magic Lantern, invented around 1600. But it was not only used as a slide projector. It was also used by charlatans, magicians, people entertaining audiences to create illusions. This is the origin of the saying “smoke and mirrors”. Because they used smoke and mirrors with the Magic Lantern to create live projections in the air, in the smoke. So the Magic Lantern became much more than a slide projector – actually a way of creating illusions that were by definition not real.
You could say that Artificial Intelligence is today’s Magic Lantern. We’ve all seen images of Sora, OpenAI’s video production tool. And if you look at OpenAI’s website, they claim that they’re not working on video production. They actually intend to model the physical world. That’s a very big deal if that is true. Obviously it’s not true. At least I think I’m one of the more sceptical ones. But those are the claims being made. If we can actually use these models to model the physical world, that’s a big step towards artificial general intelligence.
Is GenAI overhyped? Reviewing the arguments for and against
If AI is today’s Magic Lantern, it begs the question, where are the smoke and where are the mirrors? And people who lead organizations should ponder a few questions: How good are AI capabilities today? Is AI overhyped? What is the trajectory? Will it continue to go at this pace? Will it slow down? Re-accelerate? How should I respond? Do we need to jump on it? Do we need to wait and see? Let everybody else do the first experience, experience the pains, and then we will adopt whatever works? What are the threats and what are the risks? These are common questions, but given the pace of things, they are crucial.
To answer these questions, one could look to the people who develop all this new technology. But the question is whether we can trust them. Sam Altman is looking for $7 trillion. I think the GDP of Germany is what? $4 trillion or $5 trillion. Last week Eric Schmidt, ex-Google CEO, stated on TV that AI is underhyped. He said the arrival of a non-human intelligence is a very, very big deal. Then the interviewer asked: is it here? And his answer was: it’s here, it’s coming, it’s almost here. Okay, so what is it? Is it here or is it coming? Anyway, he thinks it’s underhyped.
We need to look at the data, but even that isn’t trivial. Because if you look at generative AI, Large Language Models and how to measure their performance, it’s not easy. Because how do you determine if a response is actually accurate or not? You can’t measure it easily. In any case, we see the field progressing, and we’ve all seen the news around models beating bar exams and so on.
The key thing here is that all this progress is based on the AI scaling hypothesis, which states that as long as we throw more data and compute at it, we’ll advance. We’ll get ahead. This is the secret hypothesis that people are basing their claims on. And there are incentives for the industry to make the world believe that we’re close to artificial general intelligence. So we can’t fully trust them in my opinion, and we have to keep looking at the data. But the data tells us we’re still advancing. So what does that mean? Because current systems are anything but perfect. You must have seen ample examples. One is from Air Canada. They deployed a chatbot for their customer service, and the chatbot gave away free flights. It was a bug in the system.
That brings us to the skeptical view. What are the arguments? One is about large language modelling or generative AI in general: the flaws that we’re seeing are not bugs to be fixed. The way this technology works, by definition, has these flaws. These flaws are features, they’re not bugs. And part of that is that the models do not represent how the world works. They don’t have an understanding of the world. They just produce text in the case of a Large Language Model.
On top of that, they claim that there are diminishing returns. If you analyze the performance, for instance, of the OpenAI stuff that’s coming out, they claim that if you look at the benchmarks, it’s not really progressing that much anymore. And OpenAI hasn’t launched GPT-5, so they’re probably struggling. And all the claims are based on these scaling laws, and those scaling laws can’t go on forever. We’ve used all the data in the world, all the internet by now. So we’re probably hitting a plateau. This is the skeptical view. So on the one hand we hear all the progress and all the promises, but there are also people saying, “Look, that’s actually not the case if you really look under the hood of these systems.”
As for questions asked by organization leaders: “What do I need to do?” “How fast is this going?” Here, the predictions vary. In the Dutch Financial Times, here’s an economist saying it’s overhyped, it’s the same as always, all past technology revolutions took time and it will be the same this time. On the other hand, a recent report that came out saying this time is different: generative AI is a different type of technology and this is going to go much faster. The implication being that if you don’t stay ahead, if you don’t participate as an organization, you will be left behind soon.
The argument for generative AI is that the infrastructure is already there. It’s not like electricity, where we had to build power lines. For generative AI, the infrastructure is there. The cloud is rolled out. Software has become modular. And the technology itself is very intuitive. It’s very easy for people to interact with it because it’s based on natural language. All of those arguments are the basis for saying that this is going to go much faster. And I think some of us recognize that.
Looking ahead: how leaders should prepare
There’s a difference between adopting new tools and really changing your organization. When we think about the implications, at Rewire we try to make sense of these polarized views and form our own view of what is really happening and what it means for our clients, for our partners, and the people we work with. We have three key takeaways.
The first one is that we firmly believe that everybody needs to develop their own intuition and understanding of AI. Especially because we’re living in the smoke and mirror phase. It means that it’s important for people who have the role of shaping their organization to understand the technology and develop their own compass of what it can do, to navigate change.
The second is that you need to rethink the fundamentals. You need to think about redesigning things, re-engineering things, re-imagining your organization, asking what if, rather than adopting a tool or a point solution. You must think how your organization is going to evolve, what will it look like in five years’ time and how do we get there?
The third, is that yes, I agree with the fact of this Andrew McAfee, the economist that says generative AI is different because it goes faster. To a certain extent that’s true. But not to the point where full business models and full organizations and end-to-end processes change. Because that’s still hard work, it’s transformational work that doesn’t happen overnight. So the answers are nuanced. It’s not one extreme or the other. It is a long-term game to reap the benefits of this new technology.
Demystifying the enablers and principles of scalable data management.
In the first instalment of our series of articles on scalable data management, we saw that companies that master the art of data management consider three enablers: (1) data products, (2) organizations, and (3) platforms. In addition, throughout the entire data management transformation, they follow three principles: value-driven, reusable, and iterative. The process is shown in the chart below.
Exhibit 1. The playbook for successful scalable data management.
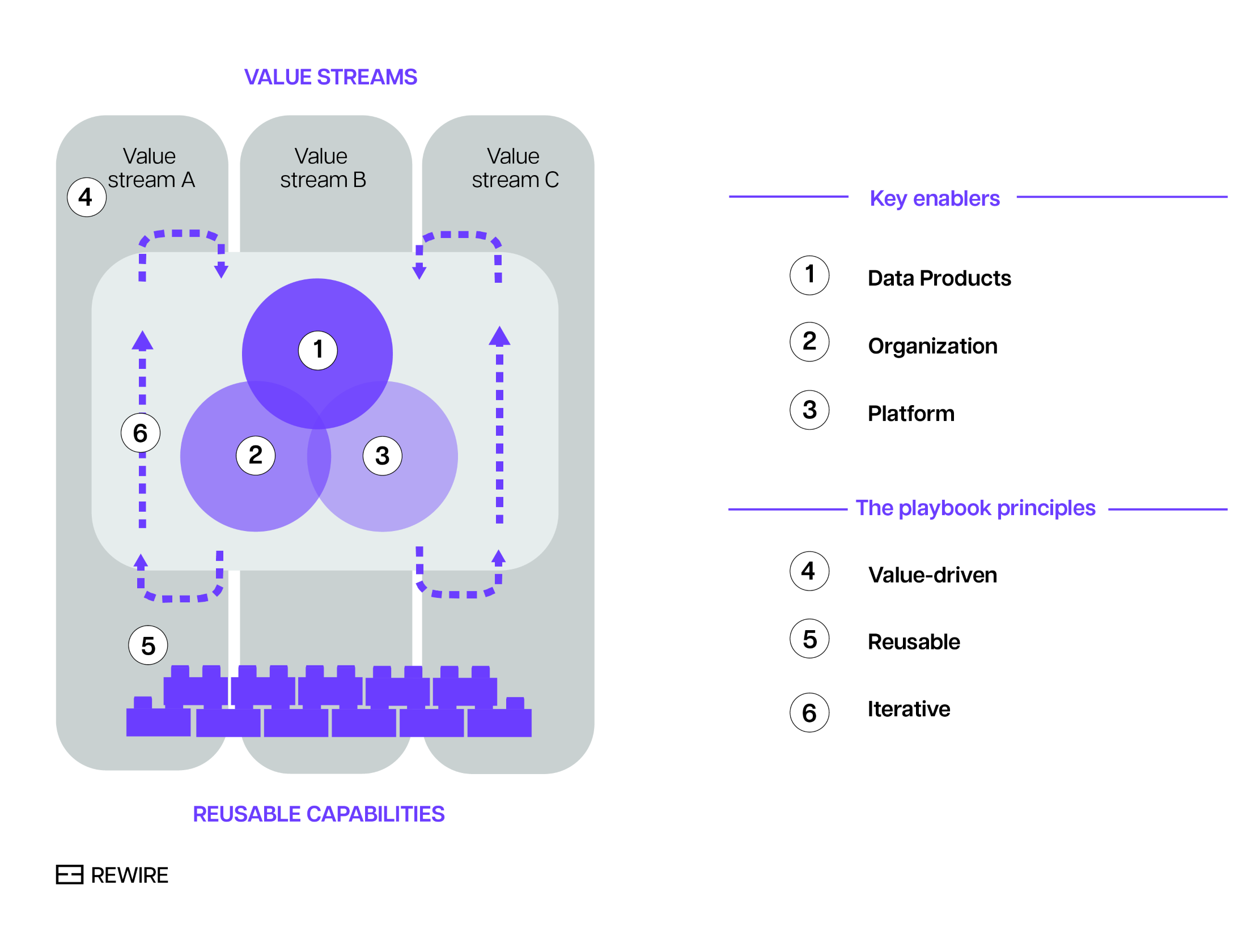
Now let’s dive deeper into the enablers and principles of scalable data management.
Enabler #1: data products
Best practice dictates that data should be treated not just as an output, but as a strategic asset for value creation with a comprehensive suite of components: metadata, contract, quality specs, and so on. This means approaching data as a product, and focusing on quality and the needs of customers.
There are many things to consider, but the most important questions concern the characteristics of the data sources and consumption patterns. Specifically:
- What is the structure of the data? Is there a high degree of commonality in data types, formats, schemas, velocities? How could these commonalities be exploited to create scalability?
- How is the data consumed? Is there a pattern? Is it possible to standardize the format of output ports?
- How do data structure and data consumption considerations translate into reusable code components to create and use data products faster over time?
Enabler #2: organization
This mainly concerns the structure of data domains and clarifying the scope of their ownership (more below). This translates into organizational choices such as whether data experts are deployed centrally or decentrally. Determining factors include data and AI ambitions, use case complexity, data management maturity, and the ability to attract, develop, and retain data talent. To that end, leading companies consider the following:
- What is the right granularity and topology of the data domains?
- What is the scope of ownership in these domains? Does the ownership merely cover definitions, and does it (still) rely on a central team for implementation or have domains real end-to-end ownership over data products?
- Given choices on these points, what does it mean for how to distribute data experts (e.g. data engineers, data platform engineers)? Is that realistic given the size or ability to attract and develop talent or should choices be reconsidered?
Enabler #3: platforms
This enabler covers technology platforms - specifically the required (data) infrastructure and services that support the creation and distribution of data products within and between domains. Organizations need to consider:
- How best to select services and building blocks to construct a platform? Should one opt for off-the-shelf solutions, proprietary (cloud-based) services, or open-source building blocks?
- How much focus on self-service is required? For instance, a high degree of decentralization typically means a greater focus on self-service within the platform and the ability of building blocks to work in a federated ways.
- What are the main privacy and security concerns and what does that mean for how security-by-design principles are incorporated into the platform?
Bringing things together: the principles of scalable data management
Although all three enablers are important on their own, the full value of AI can only be unlocked by leaders who prudently balance them throughout the whole data management transformation. For example, too much focus on platform development typically leads to organizations that struggle to create value as data (or rather, its value to the business) has been overlooked. On the other hand, too data-centric companies often struggle with scaling as they haven’t arranged the required governance, people, skills and platforms to remain in control of large scale data organizations.
In short, how the key enablers are combined is as important as the enablers on their own. Hence the importance of developing a playbook that spells out how to bring things together. It begins with value, and balances the demands on data, organization and platform to create reusable capabilities that drive scalability in iterative, incremental steps. This emphasis on (1) value, (2) reusability and (3) iterative approach lies at the heart of what companies who lead in the field of scalable data management do.
Let’s review each of these principles.
Principle #1: value, from the start
The aim is to avoid two common pitfalls: the first is starting a data management transformation without a clear perspective on value. The second is failing to demonstrate value early in the transformation. (Data management transformation projects can last for years, and failing to demonstrate value early in the process erodes the momentum and political capital.) Instead of focusing on many small initiatives, it is essential to prioritize the most valuable use cases. The crucial – and arguably the hard bit – is to consider not only the impact and feasibility of individual use cases but also the synergies between them.
Principle #2: reusable capabilities
Here the emphasis is on collecting, formalizing and standardizing the capabilities from core use cases. Then, re-use them for other use cases, thereby achieving scalability. Reusable capabilities encompass people capabilities, methodologies, standards and blueprints. Think about data product blueprints that include standards for data contracts, minimum requirements on meta data and data quality, standards on outputs and inputs, as well as methods on how to organize ownership, development, and deployment.
Principle #3: building iteratively
Successful data transformation progress iteratively towards their ultimate objectives, with each step being the optimal iteration in light of future iterations. Usually this requires (1) assessing the data needs of the highest-value use cases and developing data products that address these needs. Then, (2) considering where it impacts the organization and taking steps towards the new operating model. The key here is to identify the most essential platform components. Since they typically have long lead times, it's important to mitigate gaps through pragmatic solutions - for example ensuring that technical teams assist non-technical end users, or temporarily implementing manual processes.
Unlocking the full value of data
Data transformations are notoriously costly and time consuming. But it doesn't have to be that way: the decoupled, decentralized nature of modern technologies and data management practices allow for a gradual, iterative, but also targeted approach to change. When done right, this approach to data transformation provides enormous opportunities for organizations to leapfrog their competitors and create the data foundation for boundless ROI.
This article was written by Freek Gulden, Lead Data Engineer, Tamara Kloek, Principal, Data & AI Transformation, and Wouter Huygen, Partner & CEO.
In this first of a series of articles, we discuss the gap between the theory and practice of scalable data management.
Fifteen trillion dollars. That’s the impact of AI by 2030 on global GDP according to PwC. Yet MIT research shows that, while over 90% of large organizations have adopted AI, only 1 in 10 report significant value creation. (Take the test to see how your organization compares here.) Granted, these numbers are probably to be taken with a grain of salt. But even if these numbers are only directionally correct, it’s clear that while the potential from AI is enormous, unlocking it is a challenge.
Enters data management.
Data management is the foundation for successful AI deployment. It ensures that the data driving AI models is as effective, reliable, and secure as possible. It is also a rapidly evolving field: traditional approaches, based on centralized teams and monolithic architectures, no longer suffice in a world of exploding data. In response to that, innovative ideas have emerged, such as data mesh, data fabric, and so on. They promise scalable data production and consumption, and the elimination of bottlenecks in the data value chain. The fundamental idea is to distribute resources across the organization and enable people to create their own solutions. Wrap this with an enterprise data distribution mechanism, and voilà: scalable data management! Power to the people!
A fully federated model is not the end goal. The end goal is scalability, and the degree of decentralization is secondary.
Tamara Kloek, Principal at Rewire, Data & AI Transformation Practice Area.
There is one problem however. The theoretical concepts are well known, but they fall short in practice. That’s because there are too many degrees of freedom when implementing them. Moreover, a fully federated model is not always the end goal. The end goal is scalability, and the degree of decentralization is secondary. So to capitalize on the scalability promise, one must navigate these degrees of freedom carefully, which is far from trivial. Ideally, there would be a playbook with unambiguous guidelines to determine the optimal answers, and explanations on how to apply them in practice.
So how do we get there? Before answering this question, let’s take a step back and review the context.
Data management: then and now
In the 2000s, when many organizations undertook their digital transformation, data was used and stored in transactional systems. For rudimentary analytical purposes, such as basic business intelligence, operational data was extracted into centralized data warehouses by a centralized team of what we now call data engineers.
This setup no longer works. What has changed? Demand, supply and data complexity. All three have surged, largely driven by the ongoing expansion of connected devices. Estimates vary by source, but by 2025 the number of connected (IoT) devices is projected to be between 30 to 50bn globally. This trend creates new opportunities and reduces the gap between operational and analytical data: analytics and AI are being integrated into operational systems, using operational data to train prediction models. And vice versa: AI models generate predictions to steer and optimize operational processes. The boundary between analytical and operational data becomes blurred, and requires a reset on how and where data is managed. Lastly, privacy and security standards are ever increasing, not least driven by new a geopolitical context and business models that require data sharing.
Organizations that have been slow to adapt to these trends are feeling the pain. Typically they experience:
- Slow use-case development, missing data, data being trapped in systems that are impossible to navigate, or bottlenecks due to centralized data access;
- Difficulties in scaling proofs-of-concepts because of legacy systems or poorly defined business processes;
- Lack of interoperability due to siloed data and technology stacks;
- Vulnerable data pipelines, with long resolution times if they break, as endless point-to-point connections were created in an attempt to bypass the central bottlenecks;
- Rising costs as they patch their existing system by adding people or technology solutions, instead of undertaking a fundamental redesign;
- Security and privacy issues, because they lack end-to-end observability and security-by-design principles.
The list of problems is endless.
New paradigms but few practical answers
About five years ago, new data management paradigms emerged to provide solutions. They are based on the notion of decentralized (or federated) data handling, and aim to facilitate scalability by eliminating the bottlenecks that occur in centralized approaches. The main idea is to introduce decentralized data domains. Each domain takes ownership of its data by publishing data products, with emphasis on quality and ease of use. This makes data accessible, usable, and trustworthy for the whole organization.
Domains need to own their data. Self-serve data platforms allow domains to easily create and share their data products in a uniform manner. Access to essential data infrastructure is democratized, and, as data integration across different domains is a common requirement, a federated governance model is defined. This model aims to ensure interoperability of data published by different domains.
In sum, the concepts and theories are there. However, how you make them work in practice is neither clear, nor straightforward. Many organizations have jumped on the bandwagon of decentralization, yet they keep running into challenges. That’s because the guiding principles on data, domain ownership, platform and governance provide too many degrees of freedom. And implementing them is confusing at best, even for the most battle-hardened data engineers.
That is, until now.
Delivering on the scalable data management promise: three enablers and a playbook
Years of implementing data models at clients have taught us that the key to success lies in doing two things in parallel that touch on the “what” and “how” of scalable data management. The first step is to translate the high-level principles of scalable data management into organization-specific design choices. This process is structured around three enablers - the what of scalable data management:
- Data, where data is viewed as a product.
- Organization, which covers the definition of data domains and organizational structure.
- Platforms, which by design should be scalable, secure, and decoupled.
The second step addresses the how of scalable data management: a company-specific playbook that spells out how to bring things together. This playbook is characterized by the following principles:
- Value-driven: goal is to create value from the start, with data being the underlying enabler.
- Reusable: capabilities are designed and developed in a way that they are reusable across value streams.
- Iterative: the process of value creation balances the demands on data, organization and platform with reusable capabilities that drive scalability in iterative, incremental steps.
The interplay between the three enablers (data, organizations, platforms) and playbook principles (value-driven, reusable, and iterative) are summarised in the chart below.
Exhibit 1. The playbook for successful scalable data management.
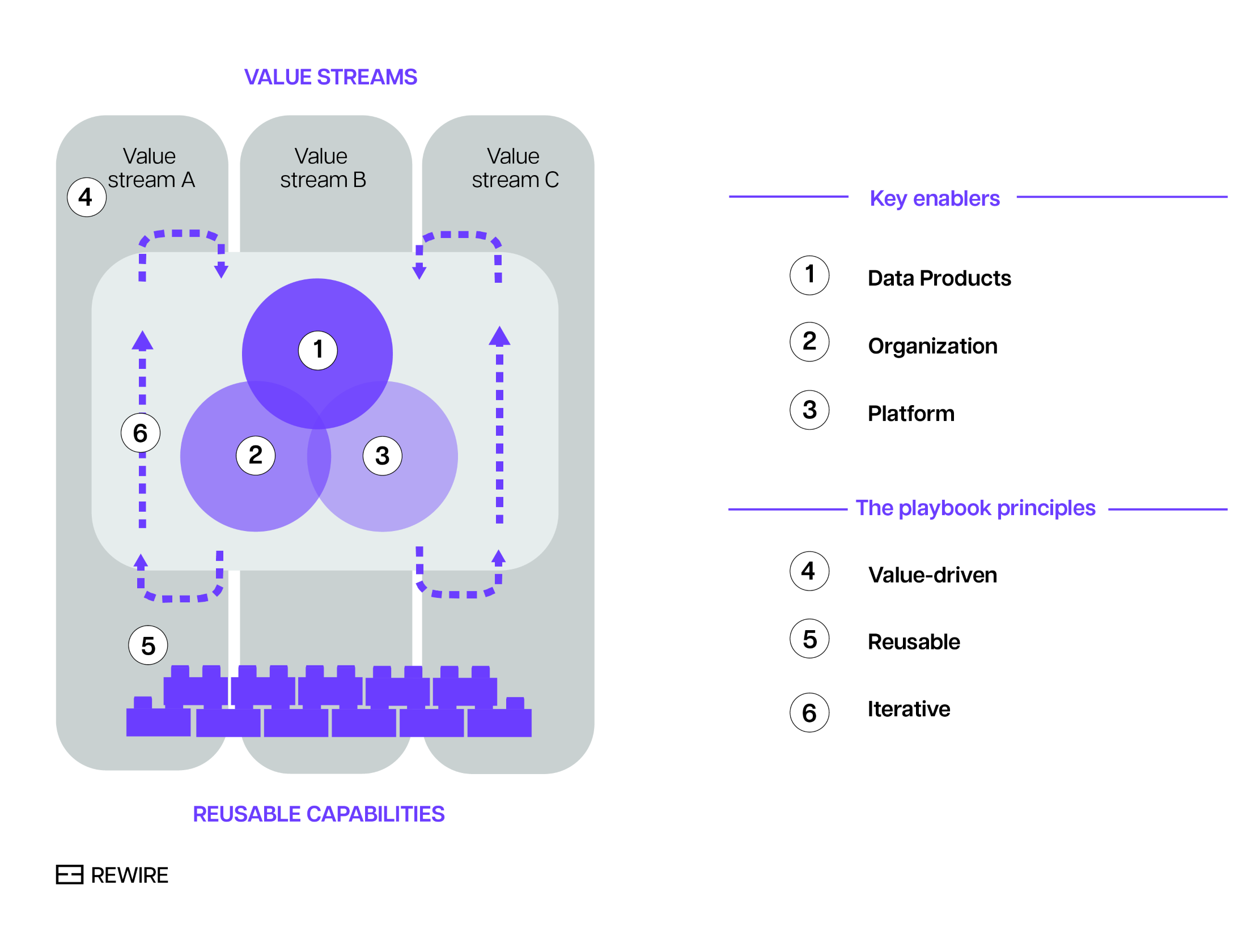
Delivering on the promise of scalability provides enormous opportunities for organizations to leapfrog the competition. The playbook to scalable data management - designed to achieve just that - has emerged through collaborations with clients across a range of industries, from semiconductors to finance and consumer goods. In future blog posts, we discuss the finer details of its implementation and the art of building scalable data management.
This article was written by Freek Gulden, Lead Data Engineer, Tamara Kloek, Principal, Data & AI Transformation, and Wouter Huygen, Partner & CEO.