The 2024 Nobel Prizes in Physics and Chemistry put the spotlight on AI. While the Physics laureates, John Hopfield and Geoffrey Hinton, contributed to its theoretical foundations, two of the three Chemistry laureates – specifically, Demis Hassabis and John Jumper – were rewarded for putting it into use.
John Hopfield developed the Hopfield network in 1982, a form of recurrent artificial neural network that can store and retrieve patterns, mimicking how human memory works. It operates by processing and recognizing patterns even when presented with incomplete or distorted data. His work was significant because it helped bridge the gap between biology and computer science, showing how computational systems could simulate the way the human brain stores and retrieves information.
Geoffrey Hinton co-invented the Boltzmann Machines, a type of neural network that played an important role in understanding how networks can be trained to discover patterns in data. He also popularized the use of backpropagation, an algorithm for training multi-layer neural networks, which considerably improved their capacity to learn complex patterns. Hinton’s contributions ultimately led to AI systems like GPT (Generative Pre-trained Transformers), which underpins ChatGPT, and AlphaFold the AI program that earned Demis Hassabis and John Jumper their Nobel prize in Chemistry.
AlphaFold solved one of biology’s greatest challenges: accurately predicting the 3D structure of proteins from their amino acid sequences. This problem had stumped scientists for decades, as protein folding is essential to understanding how proteins function, which is crucial for drug discovery, disease research, and biotechnology. AlphaFold’s predictions were so accurate that they matched experimental results with near-perfect precision, revolutionizing the field of biology. This breakthrough has wide-ranging implications for medicine and has already begun to accelerate research into diseases, drug discovery, and bioengineering.
Towards AI-driven disruption of traditional business models
Beyond the world of academia and frontier research, the AI techniques developed by the 2024 laureates are permeating the business world too. For one, the capabilities to analyse, identify patterns, and make sense of vast datasets, particularly unstructured data, rely at least partially on them.
From supply chain optimization to consumer behaviour analysis, AI holds the promise of making data-driven decisions faster, and automating a growing range of tasks. Large companies have already launched initiatives to capitalize on this, with some notable successes. Witness the case of a telecom company that generated an ROI 2.5x higher than average thanks to the judicious use of AI; or the case of an energy provider that delivered savings for consumers while increasing its own revenues; or this Supply Chain example that minimized waste and lost sales, while reducing the need for manual intervention at store level. These cases are no exceptions. Increasingly, the deployment of advanced algorithms and data management techniques play a central role in gaining competitive advantage.
Ultimately, AI ability to make sense of vast quantities of data will accelerate innovation and paves the way for new business models that will disrupt existing ones. From biotech to finance and manufacturing, the possibilities are endless, and all industries will eventually be impacted. More prosaically, the breakthroughs of the 2024 Nobel laureates herald an era when AI is not just a futuristic concept, but a key driver of competitiveness right now.
Technology and innovation expert Tom Goodwin on the merits of GenAI and how to leverage its potential.
During Rewire LIVE, we had the pleasure of hosting Tom Goodwin, a friend of Rewire and pragmatic futurist and transformation expert who advises Fortune 500 companies on emerging technologies such as GenAI. Over the past 20 years, he has studied the impact of new technology, new consumer behaviors and the changing rules of business, which makes him uniquely suited to understand the significance of GenAI today.
At the core of Tom’s thinking lies a question that all leaders should ponder: if, knowing everything you know now, were to build your company from scratch, what would it look like? At times counter-intuitive, Tom’s insights, steeped in history, provide valuable clues to answer this question. In this article, we share a handful of them.
INSIGHT 1: Technology revolution happens in two stages. In the first stage we add to what was done before. In the second stage we rethink. That’s when the revolution really happens.
Tom’s insight is derived from the Perez Framework, developed by Carlota Perez, a scholar specialized in technology and socio-economic development. The framework – based on the analysis of all the major technological revolutions since the industrial revolution – stipulates that technological revolutions first go through an installation phase, then a deployment stage. In the installation phase, the technology comes to market and the supporting infrastructure is built. In the deployment phase, society fully adopts the technology. (The transition between the two phases is typically marked by a financial crash and a recovery.)
During the first phase, there’s a frenzy – not dissimilar to the hype that currently surrounds GenAI. Everyone jumps on the technology, everyone talks about it. However, nothing profound really changes. For the most part, the technology only adds to the existing ways of doing things. In contrast, during the second stage, people finally make sense of the technology and use it to rethink the way things are done. That’s when the value is unleashed.
Take electricity as an example. In the first stage, electricity brought the electric iron, the light, the fan, the oven. These were all things that existed before. In the second stage, truly revolutionary innovations emerged: the radio, the TV, the telephone, the microwave, the microwave dinner, factories that operate 24/7, and so on. The second stage required a completely different mindset vis-à-vis what could do be done and how people would behave.
This begs the question: what will be the second stage of GenAI – and more broadly AI – be? What will be the telephone, radio, microwave for AI? Tom’s assertion here is that the degree of transformation is less about how exciting that technology is, and it’s much more about how deeply you change. Better AI will be about systems that are completely rethought and deep integrations, rather than UI patches.
Watch the video clip.
INSIGHT 2: Having category expertise, knowing how to make money, having relationships, and having staff who really know what they’re doing is probably more important than technology expertise.
Across many industries, battle lines are drawn between large traditional companies that have been around for a long time and the digitally-enabled, tech first, mobile-centric startup types. Think Airbnb vs Marriott, Tesla vs. BMW, SpaceX vs NASA, and so on.
The question is who’s going to win. Is it the digitally native companies who have created themselves for the modern era? Or is it the traditional companies that have been around for a long time? Put another way, is it easiest to be a tech company and learn how to make money in your target industry? Or be a big company who already knows how to make money but must now understand what a technology means and adapt accordingly?
Up until recently, the assumption was that the tech companies would win the battle. This proved true for a while: Netflix vs. Blockbusters, Apple vs. Nokia, etc. The assumption was that this would carry on. Understanding the technology was more important than understanding the category.
Tom’s observation is that in the past four years, these assumptions have been challenged. For example, traditional banks have got really good at understanding technology. Neobanks might be good at getting millennials to share the cost of a pizza, but they’re not that good at making money. So there’s this slow realisation that maybe digital-first tech companies are not going to win – because big companies are getting pretty good at change.
Taking a step back, it seems that the narrative of disrupt or die isn’t always true: a lot of the rules of business have not changed; incumbents just need to get a bit more excited about technology. Ultimately, having category expertise, knowing how to make money, having relationships, and having staff who really know what they’re doing is probably more important than tech expertise.
Watch the video clip.
INSIGHT 3: The AI craze is enabling a more flexible investment climate. This is an incentive for leaders to be bold.
Generative AI has spurn heated debates about the evolution of AI and divided experts and observers into two opposing groups: the AI cheerleaders and the sceptics. The former believe that AI is going to change everything immediately. The latter think that it’s a bubble.
History is littered with innovations that went nowhere. A handful of them however proved to be transformational – if in the long run. Only time will tell which group GenAI will join. In the meantime, there’s a growing realization that significant investment may be required to make meaningful steps with AI, hence a more flexible climate for capex – which is an incentive for leaders to be bold.
Tom’s insight reflects this situation: change is hard and expensive, and so regardless of one’s position in the debate, GenAI provides a unique window of opportunity to get the investor that you wouldn’t normally get. It is an amazing time to have an audience who normally wouldn’t listen to you.
Conclusion
These were but a handful of the many insights that Tom shared with us during Rewire LIVE. Taking a step back, it is clear that we are far from having realized the full value of GenAI – and, more broadly, AI. In the words of Tom, AI is a chance to dream really big and leave your mark on the world. It is yours for grab.
About Tom Goodwin
Tom Goodwin is the four time #1 in “Voice in Marketing” on LinkedIn with over 725,000 followers on the platform. He currently heads up “All We Have Is Now”, a digital business transformation consultancy, working with Clients as varied as Stellantis, Merck, Bayer, and EY to rethink how they use technology.
Tom hosts “The Edge” a TV series focusing on technology and innovation, and “My Wildest Prediction”, a podcast produced and distributed by Euronews. He has published the book “Digital Darwinism” with Kogan Page, and has spoken in over 100 cities across 45 countries.
With a 23 year career that spans creative, PR, digital and media agencies, Tom is an industry provocateur as a columnist for the Guardian, TechCrunch and Forbes and frequent contributor to GQ, The World Economic Forum, Ad Age, Wired, Ad Week, Inc, MediaPost and Digiday.
To find out more about Tom, visit www.tomgoodwin.co
Rewire CEO Wouter Huygen reviews the arguments for and against GenAI heralding the next industrial revolution, and how business leaders should prepare.
Is generative AI under- or overhyped? Is it all smoke and mirrors, or is it the beginning of a new industrial revolution? How should business leaders respond? Should they rush to adopt it or should they adopt a wait-and-see approach?
Finding clear-cut answers to these questions is a challenge for most. Experts in the field are equally divided between the cheerleaders and the skeptics, which adds to the apparent subjectivity of the debate.
The GenAI cheerleaders can point to the fact that performance benchmarks keep being beaten. Here the underlying assumption is the “AI Scaling Hypothesis”. That is, as long as we throw in more data and computing power, we’ll make progress. Moreover, the infrastructure required for GenAI at scale is already there: an abundance of cloud-based data and software; the ability to interact with the technology using natural language. Thus, innovation cycles have become shorter and faster.
On the other hand, GenAI skeptics make the following points: first, the limitations of GenAI are not bugs, they’re features. They’re inherent to the way the technology works. Second, GenAI lacks real world understanding. Third, LLMs demonstrate diminishing returns. In short, there are hard limits to the capabilities of GenAI.
The lessons of History indicate that while there might be some overhype around GenAI, the impact could be profound – in the long run. Leaders should therefore develop their own understanding of GenAI and use it to define their vision. Shaping the future is a long-term game that starts today.
Watch the video (full transcript below).
The transcript has been edited for clarity and length.
Generative AI: the new magic lantern?
Anyone recognizes this? If you look closely, not much has changed since. Because this is a basic slide projector. It’s the Magic Lantern, invented around 1600. But it was not only used as a slide projector. It was also used by charlatans, magicians, people entertaining audiences to create illusions. This is the origin of the saying “smoke and mirrors”. Because they used smoke and mirrors with the Magic Lantern to create live projections in the air, in the smoke. So the Magic Lantern became much more than a slide projector – actually a way of creating illusions that were by definition not real.
You could say that Artificial Intelligence is today’s Magic Lantern. We’ve all seen images of Sora, OpenAI’s video production tool. And if you look at OpenAI’s website, they claim that they’re not working on video production. They actually intend to model the physical world. That’s a very big deal if that is true. Obviously it’s not true. At least I think I’m one of the more sceptical ones. But those are the claims being made. If we can actually use these models to model the physical world, that’s a big step towards artificial general intelligence.
Is GenAI overhyped? Reviewing the arguments for and against
If AI is today’s Magic Lantern, it begs the question, where are the smoke and where are the mirrors? And people who lead organizations should ponder a few questions: How good are AI capabilities today? Is AI overhyped? What is the trajectory? Will it continue to go at this pace? Will it slow down? Re-accelerate? How should I respond? Do we need to jump on it? Do we need to wait and see? Let everybody else do the first experience, experience the pains, and then we will adopt whatever works? What are the threats and what are the risks? These are common questions, but given the pace of things, they are crucial.
To answer these questions, one could look to the people who develop all this new technology. But the question is whether we can trust them. Sam Altman is looking for $7 trillion. I think the GDP of Germany is what? $4 trillion or $5 trillion. Last week Eric Schmidt, ex-Google CEO, stated on TV that AI is underhyped. He said the arrival of a non-human intelligence is a very, very big deal. Then the interviewer asked: is it here? And his answer was: it’s here, it’s coming, it’s almost here. Okay, so what is it? Is it here or is it coming? Anyway, he thinks it’s underhyped.
We need to look at the data, but even that isn’t trivial. Because if you look at generative AI, Large Language Models and how to measure their performance, it’s not easy. Because how do you determine if a response is actually accurate or not? You can’t measure it easily. In any case, we see the field progressing, and we’ve all seen the news around models beating bar exams and so on.
The key thing here is that all this progress is based on the AI scaling hypothesis, which states that as long as we throw more data and compute at it, we’ll advance. We’ll get ahead. This is the secret hypothesis that people are basing their claims on. And there are incentives for the industry to make the world believe that we’re close to artificial general intelligence. So we can’t fully trust them in my opinion, and we have to keep looking at the data. But the data tells us we’re still advancing. So what does that mean? Because current systems are anything but perfect. You must have seen ample examples. One is from Air Canada. They deployed a chatbot for their customer service, and the chatbot gave away free flights. It was a bug in the system.
That brings us to the skeptical view. What are the arguments? One is about large language modelling or generative AI in general: the flaws that we’re seeing are not bugs to be fixed. The way this technology works, by definition, has these flaws. These flaws are features, they’re not bugs. And part of that is that the models do not represent how the world works. They don’t have an understanding of the world. They just produce text in the case of a Large Language Model.
On top of that, they claim that there are diminishing returns. If you analyze the performance, for instance, of the OpenAI stuff that’s coming out, they claim that if you look at the benchmarks, it’s not really progressing that much anymore. And OpenAI hasn’t launched GPT-5, so they’re probably struggling. And all the claims are based on these scaling laws, and those scaling laws can’t go on forever. We’ve used all the data in the world, all the internet by now. So we’re probably hitting a plateau. This is the skeptical view. So on the one hand we hear all the progress and all the promises, but there are also people saying, “Look, that’s actually not the case if you really look under the hood of these systems.”
As for questions asked by organization leaders: “What do I need to do?” “How fast is this going?” Here, the predictions vary. In the Dutch Financial Times, here’s an economist saying it’s overhyped, it’s the same as always, all past technology revolutions took time and it will be the same this time. On the other hand, a recent report that came out saying this time is different: generative AI is a different type of technology and this is going to go much faster. The implication being that if you don’t stay ahead, if you don’t participate as an organization, you will be left behind soon.
The argument for generative AI is that the infrastructure is already there. It’s not like electricity, where we had to build power lines. For generative AI, the infrastructure is there. The cloud is rolled out. Software has become modular. And the technology itself is very intuitive. It’s very easy for people to interact with it because it’s based on natural language. All of those arguments are the basis for saying that this is going to go much faster. And I think some of us recognize that.
Looking ahead: how leaders should prepare
There’s a difference between adopting new tools and really changing your organization. When we think about the implications, at Rewire we try to make sense of these polarized views and form our own view of what is really happening and what it means for our clients, for our partners, and the people we work with. We have three key takeaways.
The first one is that we firmly believe that everybody needs to develop their own intuition and understanding of AI. Especially because we’re living in the smoke and mirror phase. It means that it’s important for people who have the role of shaping their organization to understand the technology and develop their own compass of what it can do, to navigate change.
The second is that you need to rethink the fundamentals. You need to think about redesigning things, re-engineering things, re-imagining your organization, asking what if, rather than adopting a tool or a point solution. You must think how your organization is going to evolve, what will it look like in five years’ time and how do we get there?
The third, is that yes, I agree with the fact of this Andrew McAfee, the economist that says generative AI is different because it goes faster. To a certain extent that’s true. But not to the point where full business models and full organizations and end-to-end processes change. Because that’s still hard work, it’s transformational work that doesn’t happen overnight. So the answers are nuanced. It’s not one extreme or the other. It is a long-term game to reap the benefits of this new technology.
What Generative AI can and cannot do. And what it means for the future of business.
Meet Edmond de Belamy, the portrait painting displayed above. On Thursday October 25th in 2018, Edmond was auctioned off by Christie’s for a whopping $432,500. The signature on the bottom right of Edmond shows its artist to be

This impressive-looking formula represents a GAN network: a generative AI-model avant-la-lettre. Edmond de Belamy became the first AI-generated artwork to be auctioned off by a major auction house.
The Belamy portrait was the first time we were truly impressed by the capabilities of a GenAI model. Nowadays, its quality is nothing special. In fact, we have rapidly gotten used to image generation models producing photorealistic images, text generation models that generate e-mail texts and meeting summaries better than we could ourselves, and even LLMs that support us developers and data scientists in writing code.
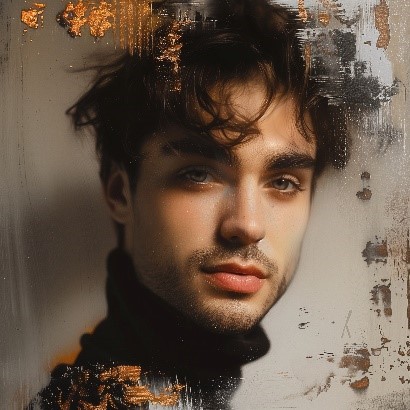
The breakthrough of GenAI, heralded by the release of ChatGPT in December 2022, has truly been amazing. It may almost lead you to believe that the age of humans is over, and the age of machines has now truly begun.
That is, until you start asking these GenAI models the hard questions. Such as:
Can you give me an odd number that does not have the letter “E” in it?
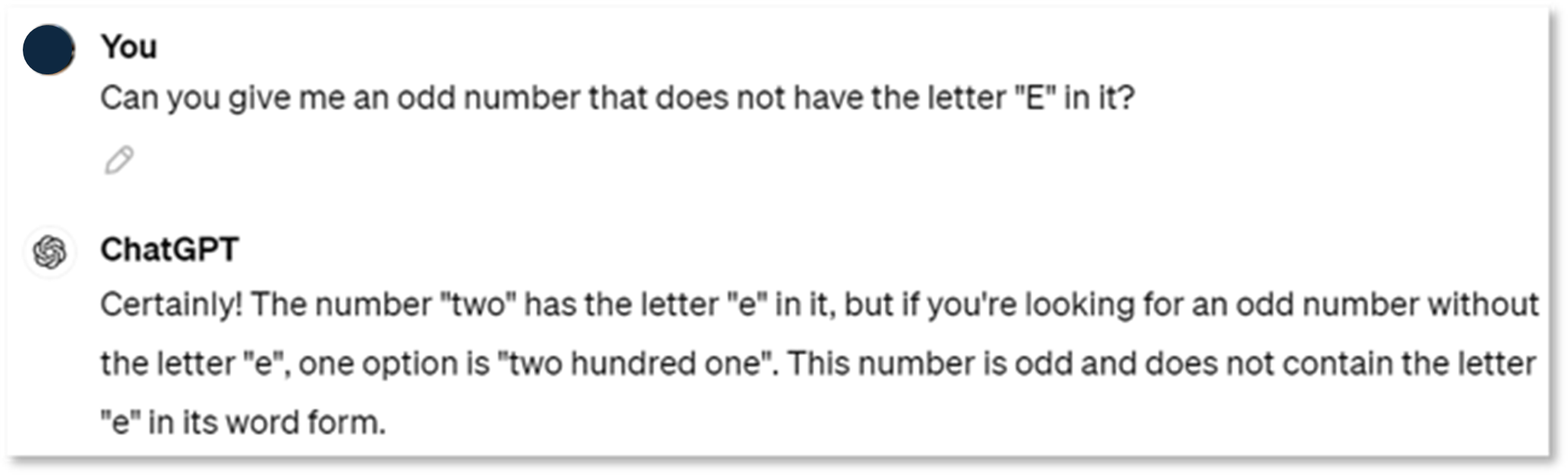
Anyone who can read could tell you GPT-4 botched that question. The correct answer would have been: no, I cannot, because such a number does not exist. Despite its ‘reasoning’, ChatGPT manages to confidently announce the number “two” has an “e” in it (note: it doesn’t), and continues to produce “two hundred one” as an example of an odd number that does not have an “e” in it (note: it does).
Another favorite pastime of ours is to ask LLM models to play chess with us. Our typical experience: they will claim they know the rules, and proceed to happily play illegal moves halfway through the game, or conjure up ghost pieces that manage to capture your knight out of nowhere.
The internet is full of entertaining examples of ChatGPT getting tasks wrong that humans solve without a second thought. These issues are not ChatGPT-specific either. Other LLM-providers, such as Anthropic, Google, Meta, and so on, face similar challenges.
So, on the one hand, GenAI models have proven to be an impressive breakthrough. On the other hand, it is clear that they are far from infallible. This begs the question:
What is the breakthrough of GenAI really?
And more importantly:
What does this mean for someone wanting to make the most of this technology?
Dissecting the GenAI breakthrough
In the 21st century we have seen the breakthrough of machine learning ending the ‘AI winter’ of the 1990s. Machine learning is essentially computers generalizing patterns in data. Three things are needed to do that well: algorithms for identifying patterns, large amounts of data to identify the patterns in, and computation power to run the algorithms.
By the start of the 2000s, we did know a fair bit about algorithms. The first Artificial Neural Networks, which form the foundation of ‘deep learning’, were published in 1943 (McCulloch et al., 1943). Random forest algorithms, the precursors to modern tree ensemble algorithms, were initially designed in 1995 (Breiman et al., 2001).
The rise of the digital age added the other two ingredients. We saw exponential growth in the amount of stored data worldwide. Simultaneously computing power kept getting exponentially cheaper and more easily accessible. All of a sudden, ‘machine learning’ and therefore AI, started to fly. Apparently, tossing large amounts of data, and huge amounts of computing power at a suitable algorithm can achieve a lot.
With machine learning we managed to have a go at quite a few problems that were previously considered difficult: image recognition, speech-to-text, playing chess. Some problems remained remarkably difficult, however. One of which was automated understanding and generation of human language.
This is where LLMs come in. In 2018, the same year our good fellow Edmond was created, OpenAI published a remarkable paper. They introduced a class of algorithms for language understanding called Generative Pre-trained Transformers, for short: “GPT” (Radford et al., 2018). This combined two key ideas. One that allowed them to “pre-train” language models on low-quality text as well as beautifully crafted pieces. And another that allowed these algorithms to benefit efficiently from large amounts of computing power. Essentially, they asked these models to learn how to predict the next word in a sentence, ideally enabling them to generate their own sentence if they became good enough at that.
GPT-1 was born with this paper in 2018, but OpenAI did not stop there. With their new algorithms they were able to scale GPT-1 both in model size, but just as importantly in terms of how much data it could be fed. GPT-3, the model that powered ChatGPT at its launch in 2022, was reportedly trained on almost 500 billion text tokens (which is impressive, but not even close to the ‘entire internet’, as some enthusiasts claim), and with millions of dollars of computing power.
Despite its insane jump in performance, the fundamental task of GPT-3 remains the same as it was for GPT-1. It is a very large, very complex machine learning language model trained to predict the next word in a sentence (or predict the next ‘token’, to be more precise). What is truly remarkable, however, is the competencies that emerge from this skill, which impressed even the most sceptical.
Emergent competencies of Large Language Models
Predicting the next word is cool, but why are these LLMs heralded by some as the future of everything (and by some as the start of the end of humanity)?
Despite being trained as “next-word-predictors” LLMs have begun to show competencies that truly do make them game changers. To be able to predict the next word based on a prompt, they have to do three things:
- Understand: making sense of unstructured data from humans and systems;
- Synthesize: processing information to ‘connect the dots’ and formulate a single, sensible response;
- Respond: generating responses in human or machine-readable language that provide information or initiate next steps.

Let’s unpack all this by looking at an example.
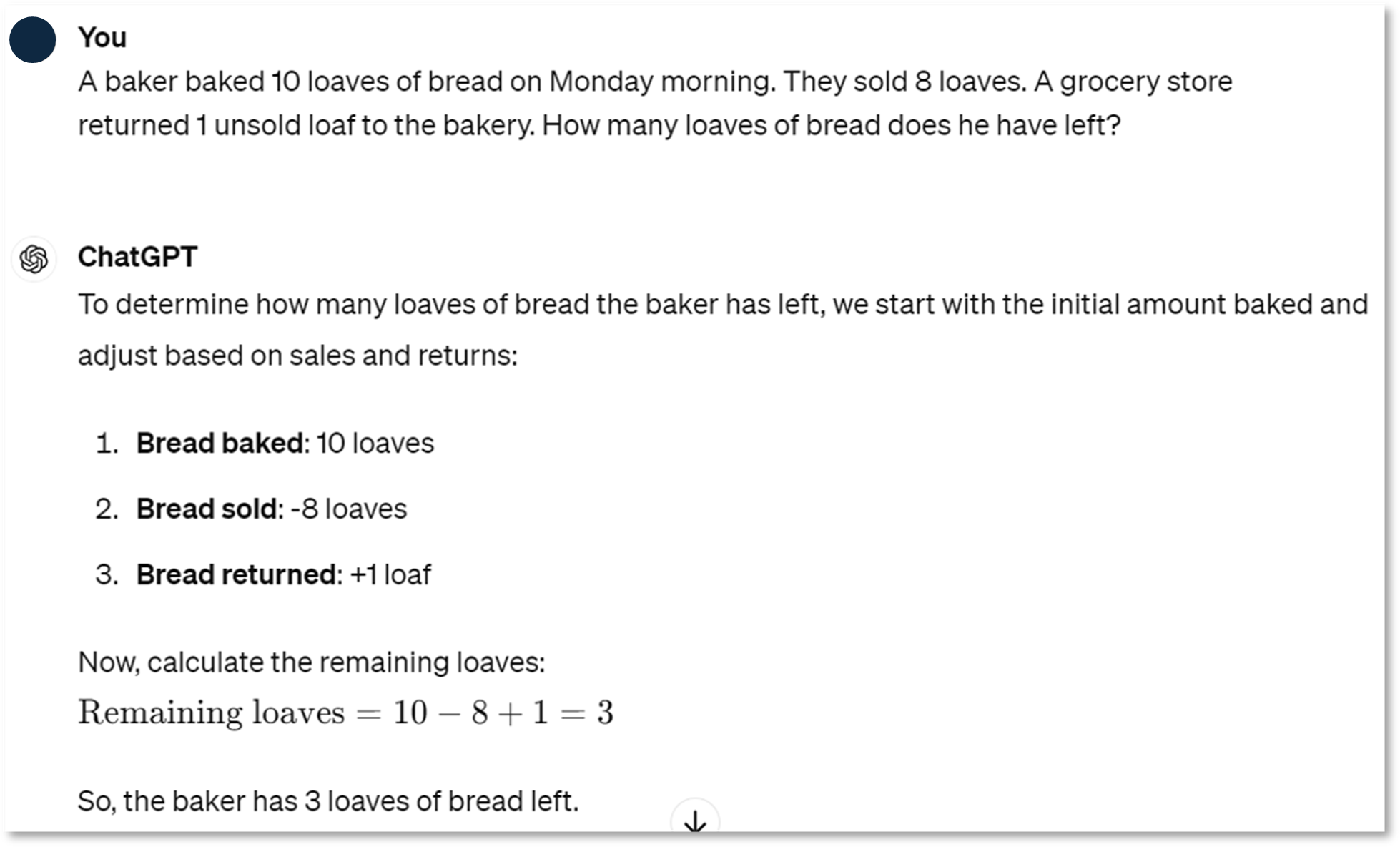
In this example, we asked ChatGPT powered by GPT-4 to solve a problem that may seem easy to anyone with basic arithmetic knowledge. Let’s pause to appreciate why ChatGPT’s ability to solve the problem is remarkable regardless.
Firstly, ChatGPT understands what we are asking. It manages to grasp that this question requires logical reasoning and gives us an answer that includes argumentation and a calculation. Furthermore, it can synthesize a correct response to our request, generating essentially ‘new’ information that was not present in the original prompt: the fact that we will have 3 loaves of bread left at the end of the day. Finally, it can respond with an answer that makes sense. In this case, it’s a human language response, wrapped in some nice formatting, but ChatGPT can also be used to generate ‘computer language’ in the form of programming code.
To put this into perspective: previous systems that were able to complete such prompts, would have generally been composed of many different components working together, tailored to this particular kind of problem. We’d have a text understanding algorithm, that extracts the calculation to be done from the input prompt. Then a simple calculator to perform the calculation, and a ‘connecting’ algorithm that feeds the output from the first algorithm into the calculator. Finally, we’d have a text generating system that takes the output and inputs the text into a pre-defined text template. With ChatGPT – we can have one model perform this whole sequence, all by itself. Lovely.
To put it in even more perspective: these types of problems were still difficult for older versions of ChatGPT. It shows that these emerging competencies are still improving in newer versions of state-of-the-art models.
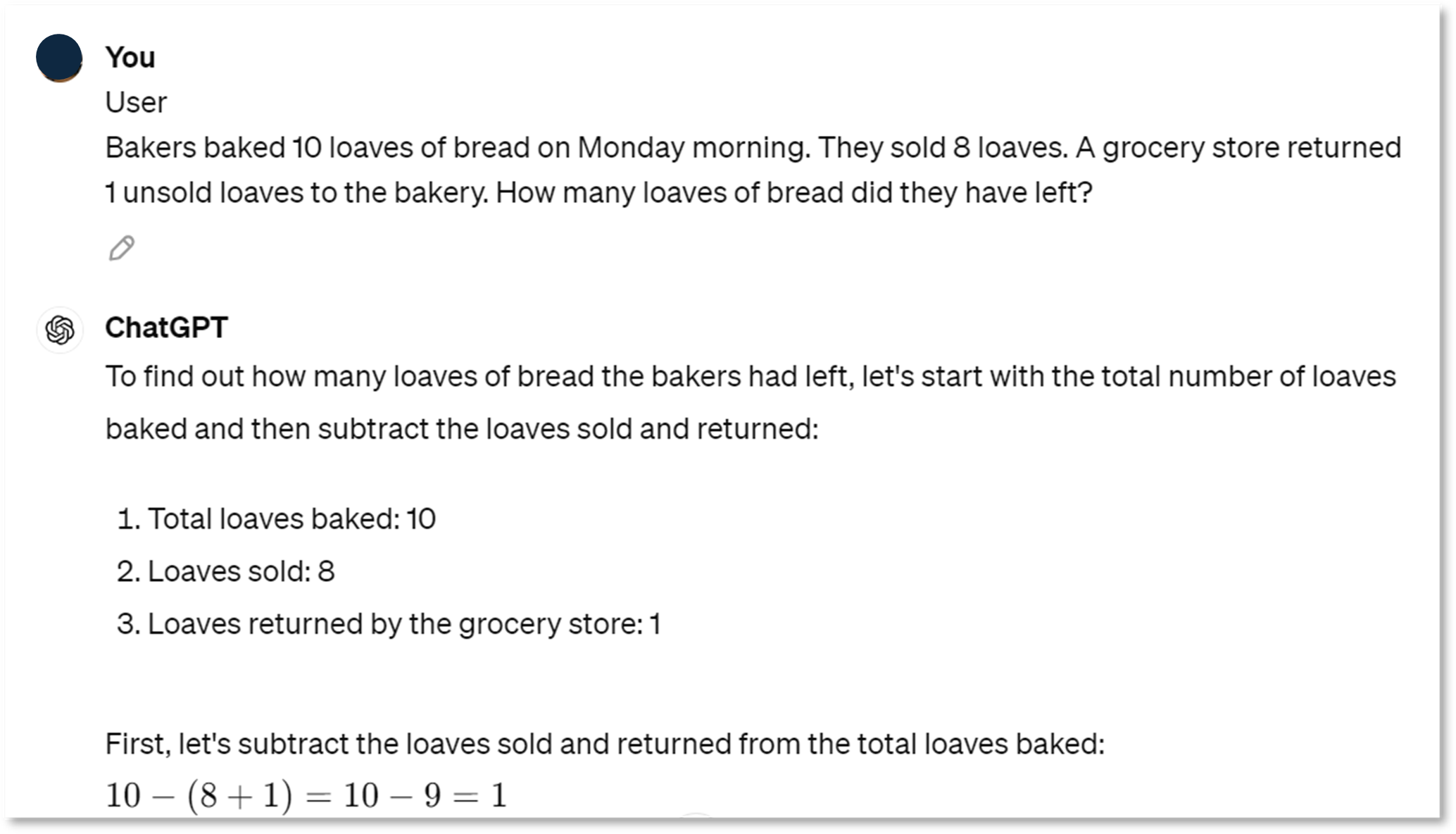
So far, we haven’t even considered models that can do understanding, synthesis and generation across multiple types of input and outputs (text, images, sound, video, etc.) These multi-modal models are all the rage since early 2024. The world of GenAI is truly moving quickly.
So what does it mean for businesses?
The talents of GenAI are truly impressive, making them a very versatile tool that opens up endless possibilities. It can craft beautiful e-mails, help generate original logos, or speed up software development.
Even more promising is GenAI’s ability to make sense of unstructured data at scale. Estimates put the amount of unstructured data to be about 80-90% of all the data that companies possess. Extracting value from this data through traditional means is time-consuming and challenging at best. This has led to unstructured data being largely ignored for many commercial use cases. Yet GenAI can plough through it and generate outputs tailored to the desired use cases. You can uncover the needles in haystacks to fuel human decisions, or enable more traditional AI systems to learn from this data. Imagine how powerful these systems would become if you increased the amount of useful information available to them by a factor 5 to 10.
Now, if you’ve been following some of the AI news lately, you’ll know we can take it even one step further and think about ‘Agentic AI’, that is, agents powered by AI. These systems that cannot only think, but actually do. In future, they will likely enable large scale automation at first, and later complete organizational transformations.
Research into how to make this work is in full swing as of the summer of 2024. The extent to which autonomous AI agents are already feasible is a hotly debated topic. At any rate, ‘simple’ agents, are now being developed that are beginning to capitalize on the AI promise. Making the most of these will require their users to carefully consider how to manage their performance, and balance two opposing characteristics: hallucinations (i.e. nonsensical results, which some argue are an inescapable feature of LLMs), and their effectiveness.
Against this backdrop, it won’t be long before the early adopters get a head start on the laggers. Teams and organizations that take the time to identify opportunities and capitalize on them are set to move far ahead of the competition.
Fine-tuning can worsen factual correctness in specialized application domains. We discuss the implications.
This article originally published on LinkedIn. The writer, Wouter Huygen is partner and CEO at Rewire.
A new paper reveals that fine-tuning is not a wonder drug for prevailing LLM hallucinations. Rather the reverse: fine-tuning can actually worsen performance when aiming to develop factual correctness in specialized application domains.
Using supervised learning on new knowledge fine-tunes hallucinations, instead of enhancing accuracy
These findings could have profound implications. What if these areas precisely provide most of the value from LLM use cases?
The hard problem of hallucinations
The beauty of LLMs is that they are very generic and general-purpose: they contain “knowledge” on a very wide range of subjects covered in the training data. This forms the basis for the claim that the current path will get us (close) to AGI. I don’t think that is true, but that’s for another day.
Clearly, generative AI currently works only up to a point. Measuring hallucination rates is notoriously difficult, but roughly speaking the tech works well in 80% of the cases. And yes, performance depends on many factors including the prompting abilities of the user. That being said, getting rid of the remaining 20% is arguably the biggest headache of the AI industry.
A long standing question in neuroscience and philosophy is how consciousness arises in the brain. How does a bunch of molecules and electromagnetic waves produce the miracle of our conscious experience? This is referred to as the hard problem of consciousness. But what if science has the premise all wrong? What if consciousness does not arise from matter, but matter is (an illusion) formed in consciousness?
Hallucinations are the current hard problem-to-crack for AI
Similarly, hallucinations are a cause of generative AI technology, not a consequence. The technology is designed to dream up content, based on probabilistic relationships captured in the model parameters.
Big tech proclaims that the issue can be solved through further scaling, but experts in the field increasingly recognize we have to view it as a remaining feature, not a bug. After all, who would not be hallucinating after reading the entire internet ;-)
For the short term, the applicability of LLMs – despite their amazing feats – remains more limited than we might hope. Especially in high stakes situations and/or very specialized areas. And these might just be the areas that herald the most value (e.g. in healthcare, proving accurate diagnostic/treatment solutions).
Unless fundamental algorithmic breakthroughs come along, or scaling proves to work after all, we have to learn how to make the best of what we've got. Work with the strengths, while minimizing downside impact.
Using Fine-Tuning to develop domain specific applications
Since the beginning of the Gen AI hype, fine-tuning is touted as one of the ways to improve performance on specific application areas. The approach is to use supervised learning on domain-specific data (e.g. proprietary company data) to fine-tune a foundational (open source) model to specialize it for a certain use case and increase factuality.
Intuitively this makes sense. The foundation model is pre-trained on generic text prediction with a very broad base of foundational knowledge. Further fine-tuning would then provide the required specialization, based on proprietary and company-specific facts.
Fine-tuning does not work well for new information
A recent paper investigates the impact of fine-tuning on new information. The authors aimed to validate the hypothesis that new knowledge can have unexpected negative impact on model performance, rather than improving it in a specific area. The outcomes are surprising, counter-intuitive at first glance, and impactful.
Fine-tuning with new knowledge works much slower than for existing knowledge (i.e. knowledge that was included in the pre-training data set). But most importantly, beyond a certain point of training, new knowledge deteriorates model performance on existing knowledge. In other words, incorporating specific new information in fine-tuning increases hallucinations. Worse yet, the hallucination rate grows linearly with more training in unknown content.
In intuitive terms, it seems as if the model gets confused with new information and “unlearns” existing knowledge.
Exhibit 1. Train and development accuracies as a function of the fine-tuning duration, when fine-tuning on 50% Known and 50% Unknown examples.
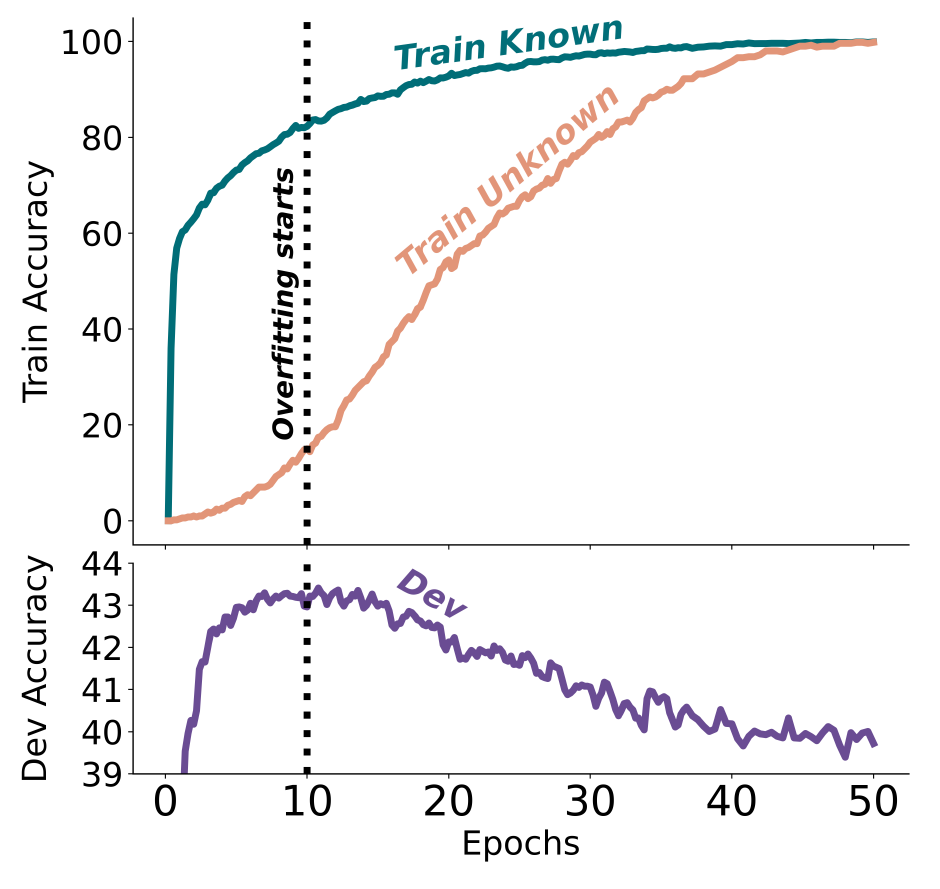
Source: paper from Zorik Gekhman et al.
These conclusions have serious implications for anyone aiming to develop specialized LLM use cases. Fine-tuning remains useful for strengthening model performance in known areas as well as improving the form and structure of the desired output. But using fine-tuning to increase factuality on new information does not work well and has undesirable, opposite affects.
The unfortunate correlation between accuracy and value
Using LLMs to build knowledge assistants is a promising use case across many fields. These use cases thrive well in highly knowledge-intensive industries, allowing users to query situation specific information on-demand. This includes healthcare workers, pharmaceutical advisors, customer service, professional services, etc. Not only do these assistants increase effectiveness and efficiency of their users, they also allow to accumulate enterprise knowledge and IP in a much more sustainable and scalable manner. They become like digital co-workers that never resign, unless you fire them.
As long as humans can be in the loop, verifying output, or when the impact of inaccurate information is low, the current LLM technology is already good enough. But in many situations, most of the value would actually come from reliability and factual correctness rather than an 80%- answer that can be manually adjusted (like drafting an email).
What to do instead?
To enhance performance in specific application areas amidst existing technological constraints, companies and developers must adopt a pragmatic and empirical engineering approach. This involves employing a combination of sophisticated techniques to forge optimal solutions. Innovations like Retrieval-Augmented Generation (RAG), fine-tuning processes accounting for new versus existing knowledge, advanced context embedding, and post-processing output verification are reshaping our methodologies daily.
The new insights discussed here demonstrate the importance to stay abreast of the fast developing field to continue pushing the performance boundaries of Gen AI applications. Until new breakthroughs happen in foundation models, we have to keep learning new tricks of the trade to get the most out of today's state of the art.