How a low-cost, open-source approach is redefining the AI value chain and changing the reality for corporate end-users
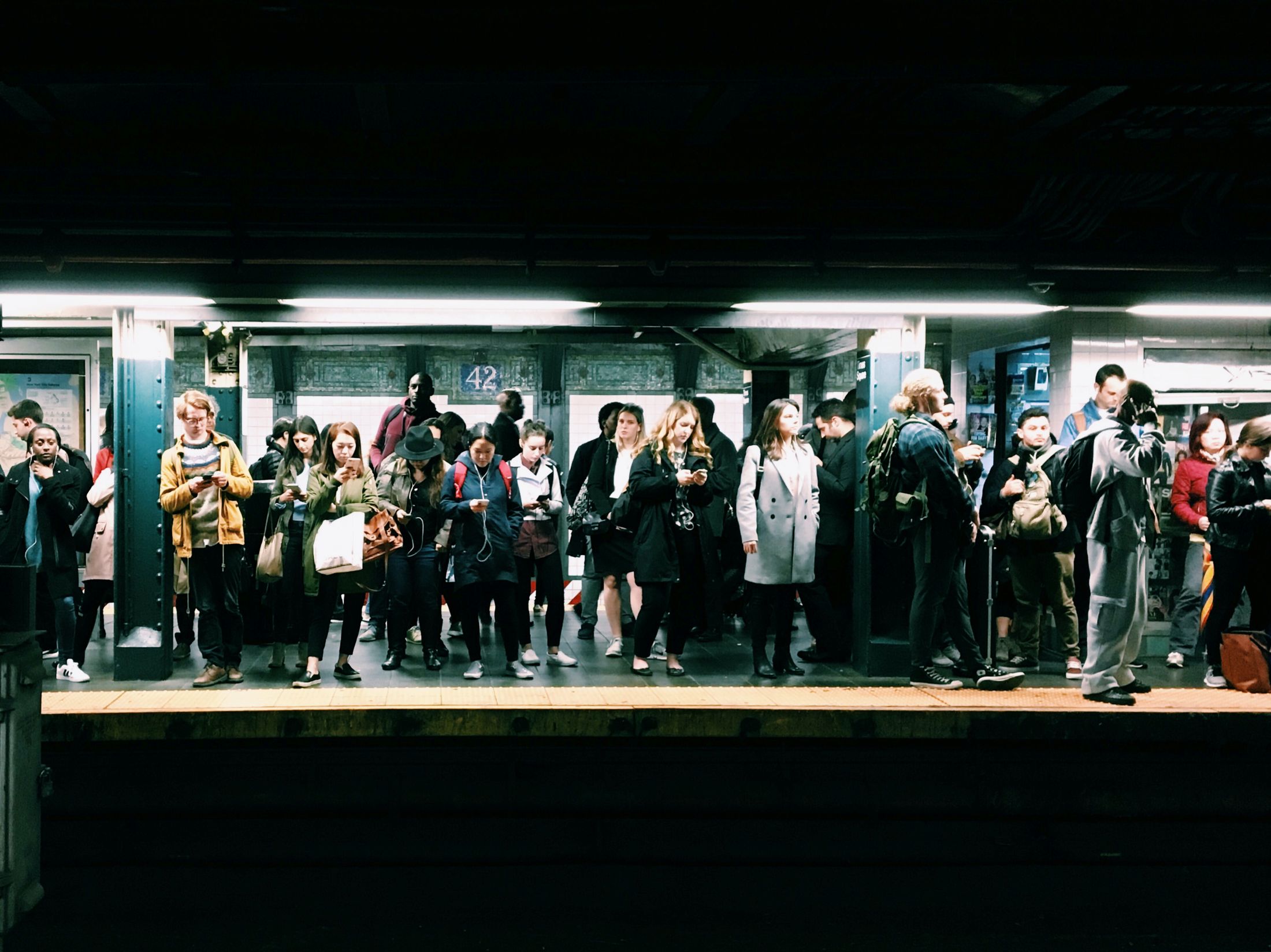
At this point, you’ve likely heard about it: DeepSeek. Founded in 2023 by Liang Wenfeng, co-founder of the quantitative hedge fund High-Flyer, this Hangzhou-based startup is rewriting the rules of AI development with its low-cost model development, and open-source approach.
Quick recap first.
From small steps to giant leaps
There are actually two model families launched by the startup: DeepSeek-V3 and DeepSeek R1.
V3 is a Mixture-of-Experts (MoE) large language model (LLM) with 671 billion parameters. Thanks to a number of optimizations it can provide similar or better performance than other large foundational models, such as GPT-4o and Claude-3.5-Sonnet. What’s even more remarkable is that V3 was trained in around 55 days at a fraction of the cost for similar models developed in the U.S. —less than US$6 million for DeepSeek V3, compared with tens of millions, or even billions, of dollars in investments for its Western counterparts.
R1, released on January 20, 2025, is a reasoning LLM that uses innovations applied to the V3 base model to greatly improve its performance in reasoning. DeepSeek-R1 achieves performance comparable to OpenAI-o1 across math, code, and reasoning tasks. The kicker is that R1 is published under the permissive MIT license: this license allows developers worldwide to modify the model for proprietary or commercial use, which paves the way for accelerated innovation and adoption.
Less is more: reshaping the AI value chain
As other companies emulate DeepSeek’s achievements, its cost-effective approach and open-sourcing of its technology signals three upcoming shifts:
1. Lower fixed costs and increased competition. DeepSeek shows that cutting-edge LLMs no longer require sky-high investments. Users have reported running the V3 model on consumer Mac hardware and even foresee its use on devices as lightweight as a Raspberry Pi. This opens the door for more smaller players to develop their own models.
2. Higher variable costs driven by higher query costs. As reasoning models become widely available and more sophisticated, their per-query costs rise due to the energy-intensive reasoning processes. This dynamic creates new opportunities for purpose-built models – as opposed to general-purpose models like OpenAI’s ChatGPT.
3. Greater value creation down the AI value chain. As LLM infrastructure becomes a commodity (much like electricity) and companies develop competing models that dent the monopolistic power of big tech (although the latter will continue to push the boundaries with new paradigm breakthroughs), the development of solutions in the application layer – where most of the value for end-users resides – becomes much more accessible and cost-effective. Hence, we expect accelerated innovations in the application layer and the AI market to move away from “winner-takes-all” dynamics, with the emergence of diverse applications tailored to specific industries, domains, and use cases.
So what’s next for corporate end-users?
Most corporate end-users will find that having a reliable and “good enough” model matters more than having the absolute best model. Advances in reasoning such as R1 could be a big step for AI agents that deal with customers and perform tasks in the workplace. If those are available more cheaply, corporate adoption and bottom lines will increase.
Taking a step back, a direct consequence of the proliferation of industry and function-specific AI solutions built in the application layer is that AI tools and capabilities will become a necessary component for any company seeking to build competitive advantage.
At Rewire, we help clients prepare themselves for this new reality by building solutions and capabilities that leverage this new “AI commodity” and turning AI potential into real-world value. To explore how we can help you harness the next wave of AI, contact us.